Integrating AI and Plant Biology for Sustainable Solutions
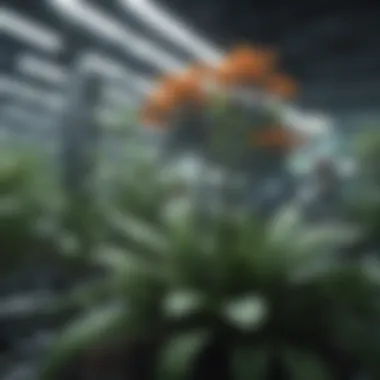
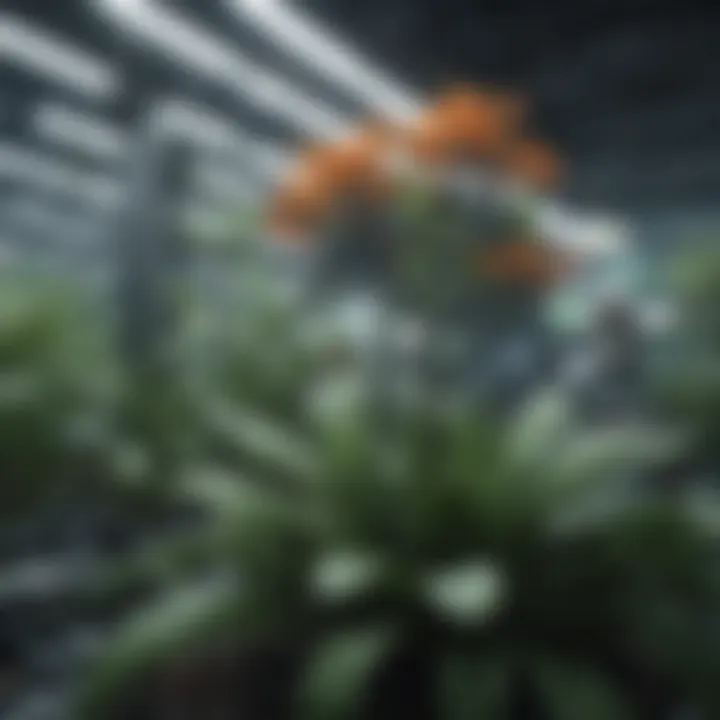
Intro
In the evolving landscape of scientific exploration, the convergence of artificial intelligence and plant biology marks a significant milestone. The utilization of AI to enhance plant biology research reveals a wealth of opportunities for agricultural innovation and improvements in food security. Understanding this intersection is essential not only for the advancement of these fields but also for addressing some of the pressing challenges in global agriculture today.
AI provides researchers with unprecedented analytical power, enabling more efficient data processing and interpretation. This integration allows scientists to generate insights from vast datasets generated through plant studies, which traditional methods often struggle to handle. As we begin to unpack this complex intersection, the implications of AI in plant biology become increasingly relevant.
The subsequent sections will delve into an overview of research findings, historical context, and the current trends shaping this interdisciplinary relationship.
Prologue to Plant AI
The integration of artificial intelligence (AI) within plant biology represents a fundamental shift in how we understand and interact with the natural world. The importance of this topic lies not only in the technological advancements it brings but also in its potential to address pressing global challenges. From food security to environmental sustainability, the confluence of AI and plant sciences offers transformative possibilities that are worthy of exploration.
Defining Plant AI
Plant AI refers to the application of artificial intelligence technologies specifically tailored for plant research and agricultural practices. This entails using machine learning, data analysis, and other AI techniques to process complex biological data about plants. A primary aim of Plant AI is to enhance our understanding of plant physiology, improve crop yields, and develop sustainable practices. By leveraging vast datasets, AI can identify patterns that may not be apparent to human researchers, thus offering insights into plant behavior and growth conditions.
Historical Context and Evolution
The intersection of plant biology and AI is not merely a recent trend; it has a rich historical background. Initially, botany was grounded in observational studies, with researchers manually collecting data and analyzing plant traits. The rise of computational technologies in the late 20th century opened new avenues, allowing for more sophisticated data analysis. Early AI applications in this field focused on simple tasks such as cropping pattern recognition or soil condition analysis.
As technology evolved, so did the capabilities of AI tools. The development of deep learning algorithms in the 2010s marked a significant leap forward. These algorithms enabled more advanced image recognition, allowing researchers to analyze plant diseases through photographs. Today, we see AI systems that can predict crop yields based on environmental conditions and genetic information, suggesting a trajectory of increasing sophistication and integration within plant research.
In summary, understanding the importance and evolution of Plant AI illuminates the critical role this field plays in modern biology and agriculture. As we advance, the synergy between AI and plant sciences holds the promise of revolutionizing how we approach sustainable agriculture and food production.
The Role of AI in Plant Research
The integration of artificial intelligence in plant research is fundamentally reshaping the approach to studying plant biology. Understanding the role AI plays is crucial not only in advancing research but also in applying those findings into practical, environmentally sustainable applications. AI enables researchers to handle vast amounts of data efficiently, transforming how they observe, analyze, and ultimately derive conclusions from their studies.
One significant benefit of AI in plant research is its powerful capability to analyze complex data sets quickly. Traditional methods often require prolonged periods of manual analysis, limiting the scope of research. AI reduces the time needed for data processing, allowing researchers to focus on interpretation and application. This efficiency could lead to faster discoveries and advancements in the field.
Data Analysis and Predictive Modeling
Data analysis is a cornerstone of modern plant research. With the increase in available data from various sources, user-friendly tools powered by AI are essential. Predictive modeling is one application where AI shines. It enables researchers to make informed predictions about plant growth, disease outbreaks, and environmental responses.
For example, machine learning algorithms can be trained on historical data to identify patterns that predict plant behavior under different conditions. This predictive insight facilitates proactive measures, improving crop management and sustainability strategies.
Moreover, tools such as TensorFlow provide platforms where researchers can build customized models. The potential for data-driven decision-making enhances both academic research and applied agricultural practices.
Genomic Studies
Genomic studies in plant biology require immense data analysis due to the complexity of genomes. AI simplifies this process by providing advanced analytical techniques that can uncover relationships between genes and traits.
Techniques like CRISPR gene editing have emerged alongside AI, creating an even more dynamic research environment. AI algorithms can help design CRISPR targets more effectively, predicting the results before actual experimentation. As genomic data continues to grow, the reliance on AI to decipher this information will become increasingly critical.
Researchers can harness AI for developing more resilient plant varieties, equipped to withstand harsh climates and diseases. Such advancements will be key in addressing global food security issues.
Image Recognition in Botany
Image recognition technology, a branch of AI, greatly impacts botany by streamlining the identification and classification of plant species. This technology utilizes convolutional neural networks to analyze and interpret images of plants, enhancing both research and educational outcomes.
Automated systems can now identify various species with a high level of accuracy, reducing the time researchers spend on manual identifications. Applications like PlantSnap and Google Lens exemplify how accessible this technology has become for both researchers and the public.
"The future of botanical research stands to gain significantly from the capabilities of AI in image recognition."
Language barriers in nomenclature may become less significant as standardized models provide clear classifications across regions. This shift towards digitized identification opens avenues for data collection from non-experts, potentially expanding databases of plant biodiversity.
Through the effective use of AI, plant research is entering a new era. The capacity to analyze vast datasets, improve genomic studies, and streamline image recognition will undoubtedly enhance our understanding of plant biology and agriculture. These advancements could usher in sustainable farming practices and contribute to mitigating food security challenges.
Enhancing Agricultural Practices with AI
Artificial intelligence is reshaping the agricultural sector in profound ways. The integration of AI technologies into agricultural practices enhances efficiency, productivity, and sustainability. These improvements are crucial for meeting the increasing global food demands while mitigating environmental impacts. As agricultural practices evolve, it becomes necessary to understand specific elements such as precision agriculture, automated crop monitoring, and resource management, which become central to this transformation.
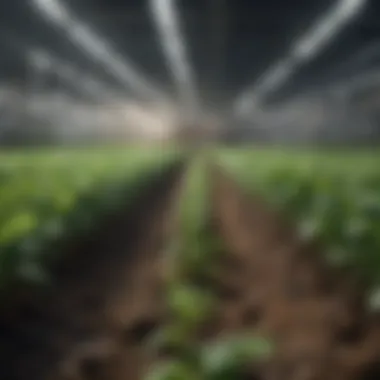
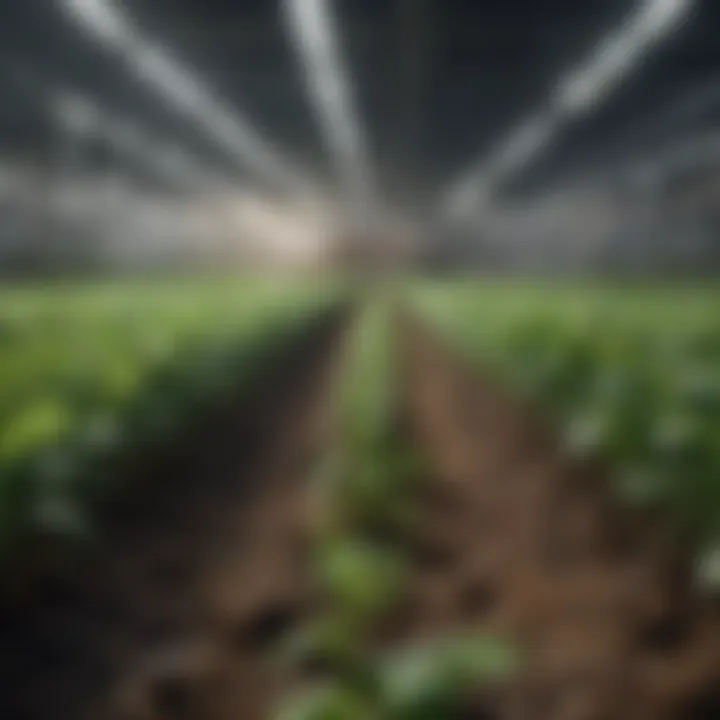
Precision Agriculture
Precision agriculture refers to the practice of using AI technologies to manage farming inputs more effectively. This includes leveraging data analytics, sensors, and machine learning algorithms to optimize crop yields and reduce wastage. Farmers can access real-time data regarding soil conditions, weather patterns, and crop health. This information allows them to make informed decisions about irrigation, fertilization, and pest control.
By utilizing precision agriculture, farmers can achieve an increase in crop output while minimizing the usage of resources. For instance, technologies such as GPS-guided equipment and drones equipped with imaging sensors enable farmers to monitor their fields accurately. This precision leads to less resource expenditure and enhanced environmental protection. Consequently, the application of AI not only addresses economic needs but also supports sustainable agricultural practices.
Automated Crop Monitoring
Automated crop monitoring systems are vital in enhancing agricultural practices through AI innovation. These systems often use drones and satellite imagery to collect data over large areas with minimal human intervention. Machine learning algorithms further analyze this data, identifying potential crop issues such as disease outbreaks or nutrient deficiencies.
One distinct advantage of automated monitoring is the immediacy of response it facilitates. Instead of lengthy inspections by farmers, AI systems can provide alerts regarding crop health in real time. This allows for prompt interventions, which can prevent loss of yield or quality.
Farmers can also benefit from historical analysis, where AI systems keep track of crop performance over decades. This predictive capability assists farmers in planning for future seasons based on past data, influencing decisions about crop varieties and cultivation methods.
Resource Management
Resource management stands at the forefront of agricultural efficiency when AI is applied strategically. AI systems can analyze resource routes and consumption patterns, providing insights that enable better management of water, fertilizers, and pesticides. The introduction of AI-powered irrigation systems exemplifies this resource optimization.
For example, systems equipped with sensors can determine the moisture levels in soil and automatically adjust irrigation schedules. Such automation ensures that crops receive the needed water without flooding fields, thereby preserving water resources.
Another significant area of focus is fertilizer management. AI solutions can calibrate applications based on soil needs, crop types, and previous yield data. This tailored approach reduces chemical runoff, which can harm local ecosystems.
Implementing AI in resource management promotes sustainability and boosts profitability by reducing waste and maximizing productivity.
In summary, enhancing agricultural practices with AI is vital for modern farming. By focusing on areas like precision agriculture, automated crop monitoring, and resource management, farmers can increase productivity while adhering to sustainable practices. The implications of such technology are profound, facing increased global food demands and environmental pressures. Through continual adaptation and integration of AI, the agricultural landscape can shift towards a more efficient and ecologically responsible future.
AI Applications in Plant Pathology
The intersection of artificial intelligence (AI) and plant pathology is increasingly recognized as significant. Plant diseases can have devastating effects on crop yields, and timely detection is crucial for effective management. AI offers advanced tools for predicting and identifying plant diseases. These technologies not only enhance understanding but also improve response strategies. This section delves into two key applications of AI in plant pathology: disease detection and management, and the impact on crop yield.
Disease Detection and Management
AI systems excel in processing large amounts of data efficiently. Machine learning algorithms can analyze data from various sources, such as satellite images, drone surveillance, and in-field sensors. This capability allows for early detection of plant diseases that might otherwise go unnoticed until they cause significant damage. For instance, convolutional neural networks are used in image recognition, enabling the identification of specific symptoms associated with various plant diseases.
- Early detection leads to timely intervention.
- Use of AI minimizes the reliance on chemical treatments.
- Algorithms evolve and improve through continuous data input.
Additionally, these systems can assist in managing diseases through predictive analytics. By analyzing environmental conditions and historical data, AI can forecast outbreaks, allowing farmers to implement preventative measures. Being proactive rather than reactive is key. AI-driven models can recommend targeted treatments, optimizing resource use and improving overall crop health.
"AI's predictive capabilities empower farmers to make informed decisions and mitigate the risks associated with plant diseases."
Impact on Crop Yield
The application of AI in managing plant diseases has a profound impact on crop yield. Improved disease detection and management directly correlate to healthier plants and increased productivity. AI enables farmers to adopt precision agriculture techniques that focus on improving yield based on specific plant needs.
- Enhanced crop monitoring reduces losses.
- Disease management increases resilience in plants.
- Predictive tools help in planning resource allocation.
A focus on crop yield driven by AI technologies can transform agricultural practices. When disease pressures are effectively managed, crops can flourish, leading to secure food supplies, which is crucial in the face of global challenges such as climate change and population growth. The benefits are clear, making AI a vital component in modern plant pathology.
Sustainability and Environmental Impact
The discussion on sustainability and environmental impact underscores the critical role that artificial intelligence (AI) can play in plant biology. As global populations rise and climate change poses increasing challenges, the need for sustainable agricultural practices becomes paramount. AI offers innovative solutions that enhance the efficiency and resilience of agricultural systems.
One of the core benefits of employing AI in this domain is the enhanced ability to manage resources judiciously. Through data-driven insights, AI can significantly reduce waste and optimize inputs such as water, nutrients, and pesticides. This not only boosts productivity but also minimizes environmental degradation. Furthermore, integrating AI systems can assist in tracking and managing the carbon footprint of farming operations effectively.
Climate Resilience through AI
AI's role in promoting climate resilience in agriculture cannot be overlooked. By leveraging advanced modeling techniques, AI can forecast weather patterns and assess the potential impacts of climate events on crop production.
For instance, predictive analytics powered by AI can identify the best planting times and crop varieties suited for the changing climatic conditions. Farmers can make informed decisions based on real-time data, ensuring that their practices align with environmental conditions. Additionally, AI can help in developing resilient crop varieties through genomic studies, which supports the long-term adaptability of agriculture in the face of climate unpredictability.
Reducing Chemical Usage
Reducing chemical usage is another pivotal aspect of sustainability in agriculture. AI can facilitate precision agriculture, allowing farmers to apply fertilizers and pesticides more selectively. By employing AI-driven technologies such as drones equipped with imaging sensors, farmers can monitor crop health at granular levels.
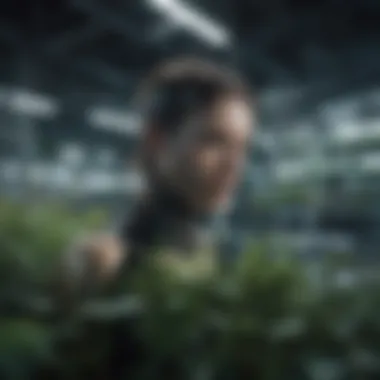
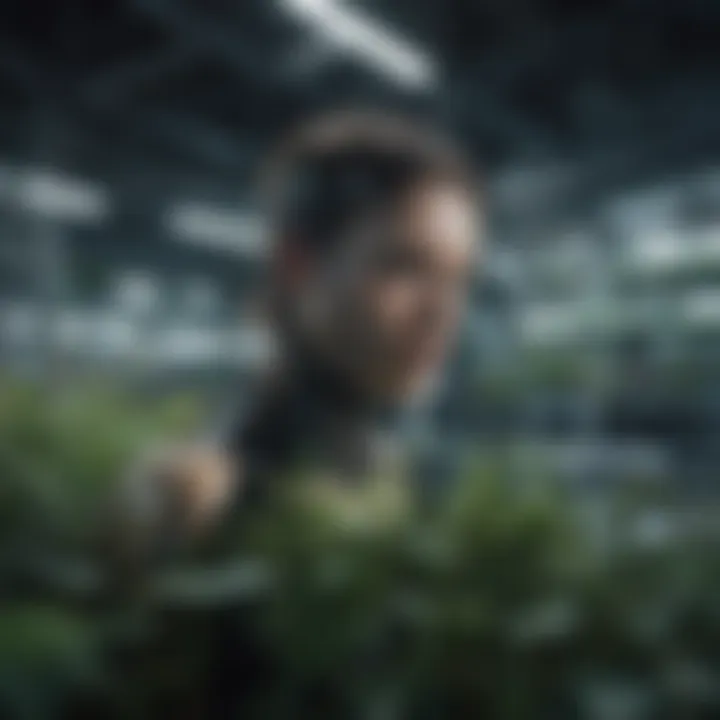
The insights gained from AI analyses enable targeted applications of chemicals only where necessary, thereby minimizing the overall quantity used. This targeted approach not only saves costs for farmers but also significantly reduces the risk of chemical runoff into local ecosystems, leading to healthier soil and water bodies.
Challenges and Limitations of Plant AI
The integration of artificial intelligence into plant biology presents remarkable opportunities but also poses significant challenges and limitations. Understanding these factors is crucial for stakeholders involved in this domain. Addressing the challenges can pave the way for optimizing AI applications in agriculture and plant research, ultimately resulting in more sustainable practices and improved crop yields. However, the journey of implementing AI in plant biology is not without hurdles, making it essential to recognize and tackle these issues systematically.
Data Privacy and Security
One of the foremost concerns with the utilization of AI in plant biology is data privacy and security. Given that AI relies heavily on vast amounts of data, including sensitive information about cultivation practices, genetic modifications, and crop management practices, the protection of such data becomes paramount.
Inadequate measures can lead to unauthorized access, exposing farmers and researchers to potential exploitation by competitors or malicious entities. Furthermore, the intertwining of agricultural data with consumer privacy raises ethical questions regarding how this information is stored and utilized.
To combat these challenges, stakeholders must implement robust security protocols, including encryption and access controls, ensuring data integrity and confidentiality. Regular audits can also help in mitigating risks and safeguarding sensitive information.
Integration Hurdles in Existing Infrastructure
Integrating artificial intelligence into existing agricultural practices requires consideration of technological compatibility with current systems. Many farms operate on legacy systems that may not be equipped to support advanced AI applications. This disparity creates a gap that presents both technical and financial challenges.
Farmers may struggle with high initial set-up costs needed for new technologies, including sensors, software, and hardware, which can deter them from adopting AI solutions. Moreover, training personnel to effectively utilize these new systems can add further complexity to the integration process.
Efforts must be made to ensure that AI technologies designed for plant biology are user-friendly and accessible. Collaboration among tech companies, agricultural experts, and farmers can help create solutions that are integratively applied without disrupting existing workflows. Recommended strategies include conducting feasibility studies prior to implementation, providing training workshops, and leveraging incremental upgrades to minimize operational disruptions.
"The effectiveness of AI in plant biology will largely depend on how well these challenges are addressed."
As the field evolves, stakeholders must continuously engage in discussions to confront these integration challenges and explore solutions that facilitate seamless adoption. This proactive approach will help in realizing the full potential of AI in plant research and agriculture.
Ethical Considerations
The integration of artificial intelligence in plant biology brings numerous advantages, but it also necessitates a careful examination of ethical considerations. As researchers and practitioners harness AI for advancing plant research and improving agricultural practices, it becomes crucial to address the ethical implications of such technologies. Understanding these implications aids in ensuring that innovations are inclusive, equitable, and responsible.
Ethical considerations in this context encompass various aspects that must be scrutinized. One significant factor is the potential bias in AI algorithms, which can manifest in several ways, affecting the outcomes of research and applications in agriculture. Furthermore, the impact on employment within the agricultural sector cannot be overlooked. As automation becomes more prevalent, it may lead to displacement of jobs, necessitating a thoughtful approach to workforce transitions.
A careful balancing act is required to navigate these ethical challenges. Here are a few reasons why ethical considerations are essential:
- Promoting Fairness: Ensuring that AI models are designed to minimize bias helps create a more equitable research environment.
- Maintaining Public Trust: Transparency in AI applications fosters trust among stakeholders, including farmers, researchers, and consumers.
- Supporting Inclusivity: Engaging diverse perspectives in AI development can lead to more innovative and relevant solutions for various agricultural communities.
"Ethical considerations are vital to harnessing the full potential of AI in plant biology and addressing concerns that could hinder progress."
Ultimately, discussing these ethical issues contributes to a more responsible integration of technology into plant biology. Now, let us focus on the specific implications that arise from the bias in AI algorithms.
Bias in AI Algorithms
Bias in AI algorithms poses a significant challenge within the realm of plant biology. This bias can emerge in various stages of the AI development process, from data collection to algorithm design. When algorithms are trained on incomplete or skewed datasets, they may produce skewed results, which can adversely impact research findings and practical applications.
Certain factors facilitate the emergence of bias:
- Data Selection: If the datasets used for training AI do not adequately represent diverse plant species or farming practices, the resultant models will reflect these limitations.
- Algorithm Design: The design of algorithms can unintentionally favor specific outcomes, especially when assumptions are made regarding the data.
Consequently, the consequences of biased AI can be profound. For example, decision-making tools developed through biased algorithms might lead to ineffective crop management practices or inaccurate disease identification, resulting in economic losses for farmers.
Impact on Employment in Agriculture
The incorporation of AI in agriculture can significantly alter the employment landscape. As precision agriculture and autonomous systems become more prevalent, traditional roles may be diminished or transformed. The concern revolves around the potential job displacement versus the creation of new opportunities in the sector.
Several aspects highlight the implications on employment:
- Automation of Tasks: Many manual jobs, such as planting, harvesting, and monitoring crops, may be automated, reducing the need for labor in those areas.
- New Skill Requirements: The demand for tech-savvy workers will increase, necessitating skilled labor in managing and maintaining AI systems.
- Resilience and Adaptation: Workers may need to adapt to new roles that emerge as a result of technological integration, which could involve continuous learning.
In addressing these implications, it is essential to promote educational initiatives that train the workforce in emerging technologies while supporting transitions for those displaced by automation.
Future Prospects of Plant AI
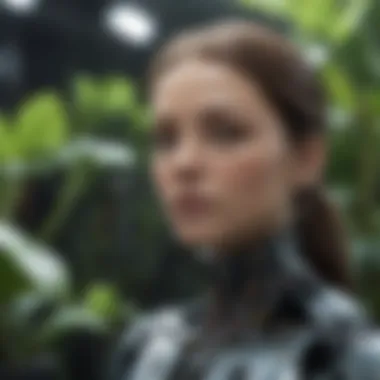
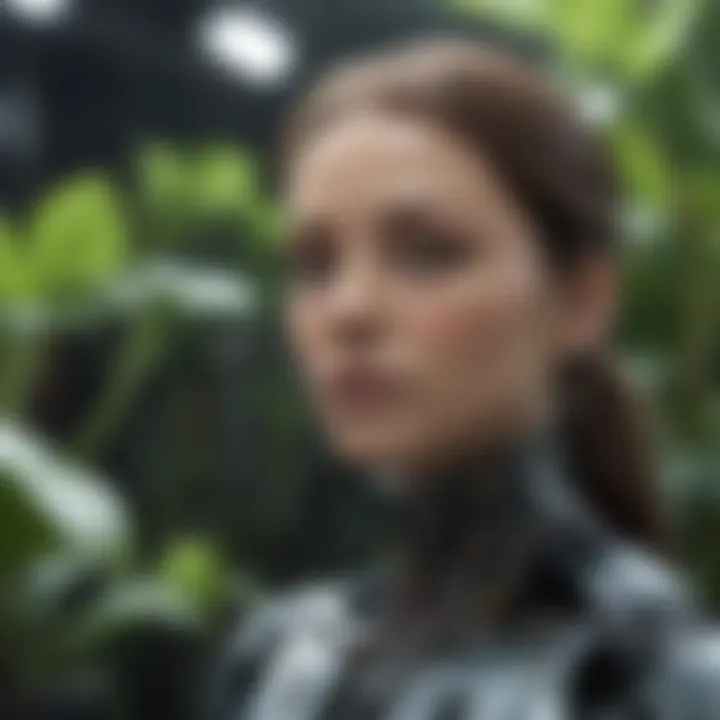
The intersection of artificial intelligence and plant biology holds numerous possibilities for innovation and advancement. The future prospects of Plant AI are crucial because they promise to reshape agricultural practices, increase research efficiency, and contribute to environmental sustainability. As technology continues to advance, the methods and tools available to researchers can lead to groundbreaking discoveries and practices that can address pressing global challenges, such as food security and climate change.
Innovative Research Directions
The future of plant biology enhanced by AI is filled with innovative research directions. Researchers are beginning to leverage advanced machine learning techniques to analyze vast datasets generated from genomic studies. This data can yield insights on plant genetics, allowing scientists to identify desired traits more efficiently. Moreover, AI can facilitate high-throughput phenotyping, enabling researchers to assess plant characteristics at unprecedented scales.
Some promising areas of research include:
- Plant Genomics: AI methodologies could indentify gene functions and interactions, leading to improved crop varieties.
- Metabolomics: Machine learning can analyze the metabolic profiles of plants, aiding in the discovery of novel compounds.
- Synthetic Biology: AI can help in the design of synthetic pathways, optimizing the production of beneficial compounds in plants.
Each of these areas highlights the transformative power of AI in plant biology research. Enhanced research capabilities can expedite the development of crops that can thrive in varying climatic conditions, thus fostering food security.
Collaborative Efforts Across Disciplines
The successful implementation of AI in plant biology requires collaboration across disciplines. Integration of knowledge from various fields will be vital in harnessing the full potential of AI applications. Biological scientists, computer scientists, agronomists, and ecologists must work together to address complex problems.
The significance of this interdisciplinary cooperation includes:
- Shared Expertise: With varied expertise, teams can design more robust AI models applicable to diverse plant biology challenges.
- Holistic Approaches: Combining perspectives from ecology, agriculture, and data science can contribute to innovative solutions.
- Resource Optimization: Collaborations can lead to pooling of resources, ensuring efficient use of funding and research facilities.
Ultimately, collaborative efforts are essential for driving the future of Plant AI. By breaking down silos between disciplines, researchers will be better equipped to tackle intricate issues and enhance the resilience of agricultural systems.
Future advancements in Plant AI are set to redefine the landscape of agriculture, bridging the gap between technology and botany in ways previously unimaginable.
Case Studies of Successful Plant AI Implementations
The integration of artificial intelligence in plant biology has led to significant advancements. Examining case studies of successful implementations enlightens us about practical applications and lessons learned. This section focuses on two aspects: global initiatives and local success stories. Both bring insight into how AI can enhance plant research and agricultural practices.
Global Initiatives
Several global initiatives highlight the role of AI in addressing challenges related to plant biology. These large-scale projects demonstrate comprehensive approaches that various organizations and governments have taken.
One notable example is the AI for Earth program by Microsoft. This initiative funds projects that utilize AI to tackle environmental issues, including agriculture. Researchers are developing models to predict crop yields and identify diseases through satellite imagery and data analysis. These methods not only improve efficiency but also assist farmers in making informed decisions.
Another critical initiative is the Digital Agriculture project in Australia. This venture integrates AI with traditional farming methods. AI algorithms process vast amounts of data, from soil health to weather patterns. Thus, farmers receive real-time insights into optimum planting times and appropriate resource use. The results have shown improved crop performance and reduced costs for farmers, leading to more sustainable agricultural practices.
"Robust AI applications can revolutionize farming techniques and ensure food security globally."
These projects emphasize the importance of collaboration between technology companies, researchers, and agricultural stakeholders. The shared goal is to leverage AI not only for profit but also for the benefit of society and the environment.
Local Success Stories
Local success stories capture how smaller-scale implementations of AI are transforming agricultural practices. These stories reflect the diversity of approaches based on geographical and cultural contexts.
One compelling example is Agri-Tech in the Netherlands. Here, startups use AI to monitor plant health in greenhouses. Sensors collect data on humidity, temperature, and light levels. By analyzing this data, farmers can optimize growing conditions. This approach has led to higher yields in limited spaces, which is essential in densely populated areas.
In India, a local initiative called Kisan Drones showcases AI in action. Farmers use drones equipped with AI-powered imaging technology to assess crop health. The drones identify specific areas that need attention, reducing the need for chemical fertilizers and pesticides. The localized data allows farmers to apply resources more strategically, minimizing environmental impact while maximizing productivity.
These localized efforts demonstrate the direct application of AI in farming. They offer practical solutions that can be adapted and scaled according to differing needs.
Examining these case studies provides a deeper understanding of how AI is reshaping plant biology and agriculture. They reveal the benefits of innovative technology in making agricultural processes more efficient and sustainable.
End
The conclusion of this article serves as a vital element in encapsulating the key themes and insights derived from our exploration of artificial intelligence within plant biology. It is important because it synthesizes the complexities discussed throughout the various sections, providing a clear overview of the converging paths of AI and plant sciences.
Summary of Key Insights
In reviewing the intersection of AI and plant biology, several key insights emerge:
- Enhanced Research Capabilities: AI facilitates data analysis and predictive modeling, allowing researchers to uncover patterns and relationships that may remain hidden in large datasets.
- Improved Agricultural Efficiency: The application of AI in precision agriculture enhances resource management, optimizes crop monitoring, and ultimately increases productivity while minimizing waste.
- Disease Management: AI aids in the detection and management of plant diseases, having a direct impact on crop yield and health.
- Ethical Considerations: The advancement of AI is accompanied by important ethical discussions concerning bias, data privacy, and potential impacts on employment within the agricultural sector.
- Sustainability: AI contributes to sustainability efforts, helping to reduce chemical usage and enabling climate-resilient agricultural practices.
Consequently, understanding these insights is essential for students, researchers, and practitioners who wish to navigate the evolving landscape where plant biology and artificial intelligence intersect.
The Path Forward
Looking ahead, it is clear that the integration of AI into plant biology presents numerous opportunities for continued innovation. The path forward includes:
- Collaborative Research: Encouraging interdisciplinary efforts between plant biologists, data scientists, and ethicists can lead to more comprehensive solutions to the challenges faced in agriculture and plant research.
- Continued Investment in Technology: Investment in advanced computational tools and AI resources will amplify research capabilities, making high-quality data analysis more accessible.
- Focus on Ethical AI: Addressing the potential biases within AI algorithms and ensuring equitable access to technology should be prioritized to mitigate any adverse impacts.
- Scaling Successful Models: Learning from case studies of successful AI applications will provide valuable guidance for implementing similar strategies in diverse agricultural settings.