Artificial Intelligence in Breast Cancer Detection
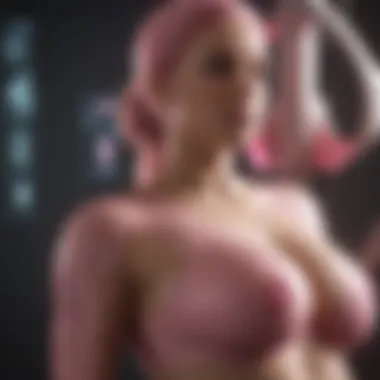
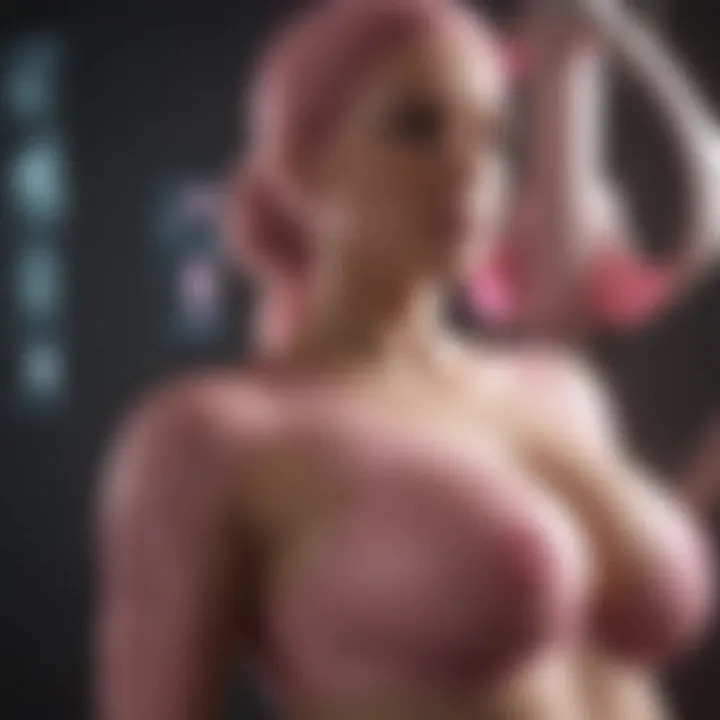
Intro
Artificial intelligence (AI) is reshaping many fields within healthcare, and its application in breast cancer detection and management is particularly noteworthy. The integration of AI technologies has become a transformative force, offering not only advancements in early diagnosis but also improvements in treatment methodologies. By examining AI's multifaceted role in breast cancer, this article aims to provide insights into the evolving landscape of medical practices in oncology, particularly focusing on efficiency and accuracy in detecting malignant cells.
The significance of AI in medicine cannot be overstated. As breast cancer remains one of the most prevalent cancers globally, early detection is paramount in improving survival rates. AI systems are capable of analyzing complex datasets, which can substantially enhance diagnostic processes. Furthermore, ethical considerations surrounding AI's integration require careful evaluation, ensuring that while we harness its capabilities, we also address potential issues regarding patient privacy and data security.
Research Overview
Key Findings
Recent studies highlight several critical findings in the domain of AI application for breast cancer:
- AI technologies, such as machine learning and deep learning, significantly improve the accuracy of mammography readings.
- Early detection through AI-assisted imaging can lead to a decrease in false negatives and improved survival rates.
- Personalized treatment plans created by AI algorithms show promising results in optimizing patient outcomes.
Study Methodology
Studies often employ a combination of retrospective and prospective analyses. Advanced imaging datasets are processed using AI algorithms to determine their effectiveness in real-world conditions. Some research utilizes public datasets to train models, while clinical trials offer insights into AI’s integration in actual healthcare settings. The outcomes are frequently compared against traditional diagnostic methods to gauge improvements.
Background and Context
Historical Background
The journey towards AI in medical diagnostics began decades ago. Initial applications were rudimentary and focused on data analysis. However, with technological advancements and the advent of robust machine learning algorithms, the scope has evolved dramatically. Researchers have since developed AI systems capable of interpreting imaging data, thus marking a significant milestone in breast cancer detection.
Current Trends in the Field
Today, AI's role in breast cancer management encompasses various functions:
- Image Recognition: AI algorithms are used to evaluate mammograms and other imaging modalities effectively.
- Predictive Analytics: AI analyzes patient data and predicts outcomes, aiding in personalized treatment pathways.
- Integration with Healthcare Systems: Many hospitals are beginning to incorporate AI tools into their radiology departments, enhancing operational efficiency and diagnostic accuracy.
"The potential for AI to transform breast cancer management hinges on collaboration between technologists and healthcare practitioners."
Preface to Artificial Intelligence in Healthcare
Artificial Intelligence (AI) plays a crucial role in modern healthcare systems. The integration of AI technologies into healthcare is reshaping how medical professionals diagnose, treat, and manage diseases. This is especially pertinent in oncology, where the stakes are high, and accurate and timely decisions are critical. AI systems assist in analyzing vast amounts of medical data, providing insights that enhance the quality of patient care.
Overview of Artificial Intelligence Technologies
Machine Learning
Machine Learning is a subset of AI focused on developing algorithms that enable computers to learn from and make predictions based on data. In breast cancer detection, machine learning techniques can identify patterns in mammography data, leading to earlier and more accurate diagnoses. One key characteristic of machine learning is its ability to improve over time as it processes more data. This adaptability makes it a popular choice in this context. However, machine learning requires substantial data sets for training, which can be a limitation when data is scarce or not representative of the broader population.
Deep Learning
Deep Learning, a specialized branch of machine learning, employs neural networks with many layers to analyze data. It shines in interpreting medical imaging, such as mammograms and MRI scans. This technology's ability to automatically detect features that may not be visible to the human eye is a key advantage. Deep learning often leads to higher accuracy in identifying abnormalities. However, it necessitates extensive computational resources and large volumes of data, which can be a barrier to widespread use in breast cancer management.
Natural Language Processing
Natural Language Processing (NLP) enables computers to understand and interpret human language. In breast cancer care, NLP allows for better management of unstructured data, such as clinical notes and research articles. Furthermore, it helps clinicians extract relevant information quickly from massive data sets, thereby enhancing decision-making processes. Its unique feature is that it bridges the gap between human language and machine understanding. Nevertheless, NLP's effectiveness may vary depending on the quality of the data and its context.
Significance of AI in Oncology
Advancements in Cancer Treatment
AI has revolutionized advancements in cancer treatment by enabling more precise targeting of therapies. Algorithms analyze genetic profiles and treatment responses, leading to personalized medicine that is tailored to an individual’s needs. This targeted approach not only improves patient outcomes but also minimizes unnecessary treatments. AI’s role in streamlining drug development processes is a noteworthy benefit, although it is important to consider the costs and time involved in implementing these advanced technologies.
Reduction of Diagnostic Errors
One significant impact of AI is the reduction of diagnostic errors, which can have severe consequences in oncology. AI systems can assist radiologists by highlighting areas of concern in imaging studies, reducing the chances of oversight. This enhancement in diagnostic confidence helps in early detection and treatment initiation. The ability of AI to continuously learn from new data means that it can adapt and refine its diagnostic capabilities over time. However, reliance on AI also raises concerns about over-dependence, where clinicians may trust AI assessments over their expertise.
Current AI Applications in Breast Cancer
Artificial Intelligence plays a transformative role in breast cancer diagnosis and management. It enhances the accuracy of imaging, streamlines pathology analyses, and improves predictions of patient outcomes. The integration of AI within these domains will lead to improved healthcare experiences, shorter time to diagnosis, and more personalized treatment approaches.
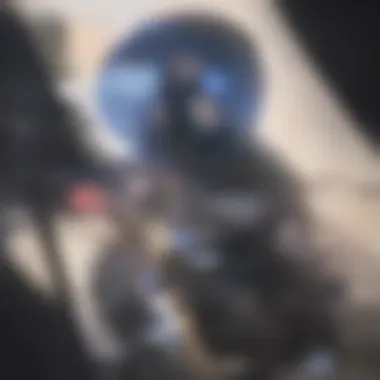
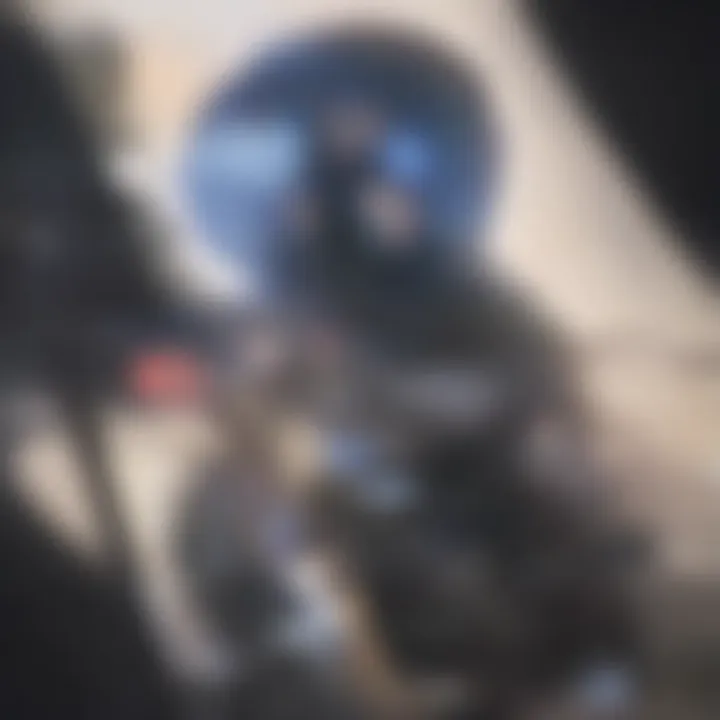
Diagnostic Imaging Techniques
Diagnostic imaging serves as a cornerstone in breast cancer detection. AI advances this process, ensuring precision in identifying malignancies that human eyes may sometimes miss.
Mammography Analysis
Mammography is the most common method for breast cancer screening. The implementation of AI in mammography analysis has revolutionized how radiologists interpret images. The key characteristic of AI in this context is its capability to recognize patterns in imaging data that can be difficult for human professionals to discern.
This technology's unique feature lies in its high sensitivity, significantly reducing the rates of false negatives. Radiologists using AI systems have reported a marked improvement in diagnostic accuracy. However, the reliance on AI must be tempered by human oversight to prevent potential overreliance on technology that could lead to false positives or missed malignancies.
Ultrasound Interpretation
Ultrasound is another vital technique for breast cancer assessment, particularly in women with denser breast tissue. AI enhances ultrasound interpretation by automating the analysis process, thus increasing efficiency. Its key characteristic is the speed at which it processes images, allowing for timely decisions in patient management.
The unique capability of AI to differentiate between benign and malignant lesions helps in directing appropriate care. However, just like with mammography, there exists a challenge. The automation may lead to underestimation of important clinical evidence that a trained radiologist might catch.
Magnetic Resonance Imaging (MRI)
MRI has become a critical imaging modality, particularly for patients at high risk of breast cancer. AI has been incorporated into MRI workflows to enhance the accuracy of tumor characterizations. The standout feature of AI in MRI analysis is its multi-parametric assessments, which incorporate various imaging attributes to form a comprehensive picture of tumor biology.
The advantages of AI integration involve higher detection rates of tumors compared to conventional interpretation alone. However, the disadvantages include the requirement for extensive training data, and the difference in performance can vary based on the quality of input into the AI systems.
Pathology and Histopathological Analysis
Pathology remains central to confirming breast cancer diagnoses. AI is improving the speed and reliability of tissue sample evaluations.
Tissue Sample Evaluation
The evaluation of tissue samples is crucial in determining cancer status. AI systems automate the process of reading slides to identify potential malignancies. The main benefit of this application is the increased speed in diagnostics, which leads to faster treatment planning.
The unique aspect of AI in tissue evaluation is its accurate quantitative assessment of cellular features, ensuring that no detail is overlooked. However, there are concerns regarding data diversity; inadequate training datasets may impact the performance and accuracy of the systems.
Automated Diagnosis Systems
Automated diagnosis systems utilize AI to interpret data from multiple sources, streamlining the pathology process. The important characteristic of such systems is their ability to operate on large datasets efficiently, reducing the burden on pathologists.
These systems’ unique feature is their capacity to integrate various diagnostic modalities to provide comprehensive assessments. However, a drawback persists in terms of the need for extensive validation in clinical settings before widespread adoption can occur.
Predictive Analytics for Patient Outcomes
Predictive analytics enhances the understanding of patient outcomes by utilizing historical data to forecast future events. This is particularly important in breast cancer management, where tailored interventions can significantly improve survival rates.
Prognostic Models
Prognostic models analyze datasets to predict the likely course of breast cancer in patients. The primary benefit is the identification of high-risk patients who may require intensified monitoring or therapy.
The unique feature of predictive models involves dynamic learning, adapting over time as new data becomes available. This can lead to improved accuracy in predictions. The downside includes the potential for overfitting models to past data, which may not always reflect future patient populations.
Survival Rate Predictions
Survival rate predictions focus on estimating outcomes based on numerous variables. This serves to guide treatment decisions and patient counseling. A key characteristic of AI in this area is its ability to process vast datasets quickly.
The significant advantage lies in offering patients informed expectations regarding their health journey. However, one must acknowledge the limitations caused by variations in demographic factors, as predictive algorithms may not generalize well across diverse populations.
Benefits of AI in Breast Cancer Management
Artificial Intelligence (AI) has made significant strides in enhancing breast cancer management. The integration of AI technologies into diagnostic and treatment processes has the potential to improve healthcare outcomes. The benefits of AI in this context are manifold, ranging from increased diagnostic accuracy to the development of personalized treatment approaches.
Enhanced Accuracy and Efficiency
AI’s ability to enhance accuracy in medical imaging and diagnostics is crucial for effective breast cancer management. By utilizing advanced algorithms, AI can analyze imaging data with remarkable precision, leading to better detection rates. This enhancement directly correlates to improved patient outcomes, making it a key aspect of AI’s role in oncology.
Reduction in False Positives and Negatives
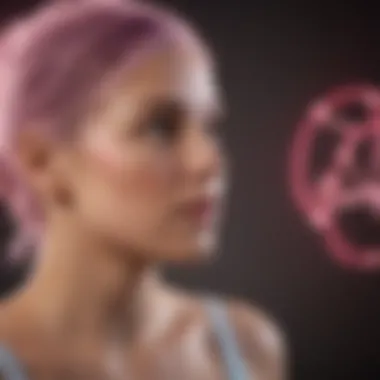
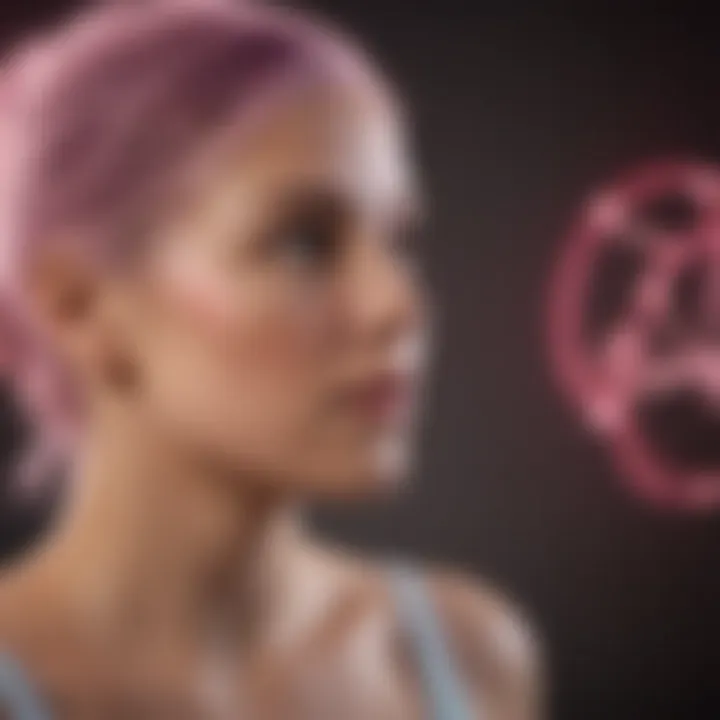
The reduction in false positives and negatives is a pivotal benefit of employing AI in breast cancer detection. With the employment of AI algorithms, there is a decrased likelihood of misdiagnosis. This improvement not only reduces patient anxiety and unnecessary follow-up procedures but also ensures that patients receive timely and appropriate treatment based on accurate assessments.
One significant characteristic of AI-driven diagnostics is its adaptability in learning from diverse datasets. This ability to learn means AI systems can continuously improve their accuracy. Consequently, this feature makes AI a popular choice among healthcare providers seeking to minimize errors in breast cancer detection.
Streamlined Workflow for Radiologists
Streamlined workflows result from the integration of AI technologies in clinical settings. Radiologists are often faced with high workloads, and AI can help alleviate the burden by pre-analyzing imaging data. This efficiency allows radiologists to focus on more complex cases that require human expertise, thus optimizing the overall workflow.
The key aspect of this benefit lies in the time-saving capabilities of AI tools. By processing and flagging potential areas of concern in mammograms or MRIs, radiologists can prioritize their reviews more effectively. This efficiency is indeed advantageous for ensuring more cases are examined in a shorter period.
Personalized Treatment Approaches
Personalized treatment approaches represent another significant advantage of AI in managing breast cancer. This concept involves tailoring therapy based on individual patient characteristics, including genetic information and tumor biology. AI can analyze vast amounts of data to identify the most effective treatment options for each patient.
Tailoring Therapies to Individual Patients
The ability to tailor therapies to individual patients enhances treatment outcomes. AI systems can analyze genomic data to predict which therapies are likely to be most effective based on a patient’s unique genetic makeup. This targeted approach reduces the trial-and-error aspect of treatment selection, allowing for more efficient use of resources.
This characteristic makes personalized treatment plans not just a beneficial strategy but a necessary evolution in cancer care. The unique feature of AI's predictive capabilities offers significant advantages, including decreased side effects and improved response rates to treatments.
Improved Monitoring and Adjustments
The improvement in monitoring and adjustments represents an essential function of AI in breast cancer management. Continuous monitoring through AI tools can provide real-time data that allows healthcare providers to make informed decisions promptly. The ability to track a patient’s response to treatment enhances clinical outcomes.
A noteworthy feature of AI in monitoring is its capacity for predictive analytics, which helps anticipate potential complications or treatment failures. This proactive approach allows for timely adjustments in treatment plans. Although the application of AI in monitoring offers numerous advantages, there is always a need for careful consideration of the integration process to ensure that patient care remains at the forefront of AI implementations.
"AI is not a replacement for clinicians but a powerful tool that enhances their capabilities to provide better patient care."
Overall, the benefits of AI in breast cancer management illustrate the potential it has to not only enhance diagnostic accuracy but also to revolutionize treatment approaches. By leveraging AI technologies, healthcare professionals can provide more efficient, personalized care to patients.
Challenges and Limitations of AI in Breast Cancer
The integration of artificial intelligence in breast cancer detection and management is not devoid of challenges and limitations. Recognizing these issues is crucial for optimizing the efficacy of AI technologies. This section will examine several specific elements that illustrate how these challenges can impact the development and deployment of AI systems in the oncology field. The relevance of addressing these obstacles is paramount, as they influence patient outcomes, healthcare delivery, and the trustworthiness of AI applications.
Data Quality and Availability
The effectiveness of AI systems relies heavily on the quality and availability of data used in training algorithms. Insufficient or biased data sets can lead to inaccuracies in diagnosis and treatment recommendations. Addressing data quality involves several important considerations.
Panel Diversity in Training Data
Diversity in training data is a significant aspect of AI model development. It refers to the inclusion of varied demographic profiles, such as age, ethnicity, and cancer subtypes, within the data set. Diverse data can enhance the robustness of AI algorithms. The key characteristic of a diverse data panel is its ability to reduce bias, which can characterize the outcome of AI predictions. A diverse training set is a beneficial choice in the context of this article because it aims to ensure that AI systems can perform accurately across different patient populations.
Nevertheless, achieving a sufficiently diverse data panel poses challenges.
- Advantages: More representative data leads to improved generalizability and lower chances of misdiagnosis for underrepresented groups.
- Disadvantages: Collecting diverse data requires extensive collaboration across various healthcare systems, which may not always be feasible due to institutional barriers.
Data Privacy Concerns
Data privacy is another major concern related to AI applications in healthcare. The handling of sensitive patient information raises ethical issues, particularly regarding informed consent and data protection. The key characteristic of data privacy concerns is the heightened scrutiny around how patient data is utilized within AI frameworks.
From a beneficial standpoint, emphasizing data privacy can enhance patient trust and facilitate greater acceptance of AI technologies.
- Advantages: Ensuring data privacy can foster stronger patient relationships and encourage more individuals to participate in AI-driven initiatives.
- Disadvantages: Stricter data protections may limit the amount of available training data for AI systems, thus inhibiting their performance and accuracy in real-world scenarios.
Interpretability and Trust Issues
Understanding how AI makes decisions is paramount for clinical acceptance. Interpretability pertains to the degree to which the workings of an AI system can be explained in understandable terms. It plays a critical role in fostering trust between patients and healthcare providers.
Understanding AI Decision-Making Processes
The AI decision-making process must be transparent for effective integration into clinical practice. Understanding these processes means that healthcare professionals can evaluate the validity of the outputs generated by AI models. A strong characteristic of interpretability is the capacity to trace how certain inputs lead to specific decisions.
- Advantages: Improved understanding can boost clinician confidence in AI tools, leading to better collaboration.
- Disadvantages: Many AI models, particularly deep learning networks, operate as black boxes. This opacity reduces the likelihood that healthcare practitioners will fully trust their recommendations.
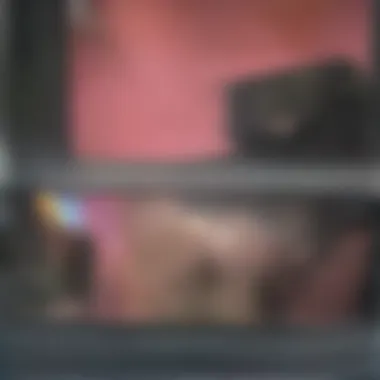
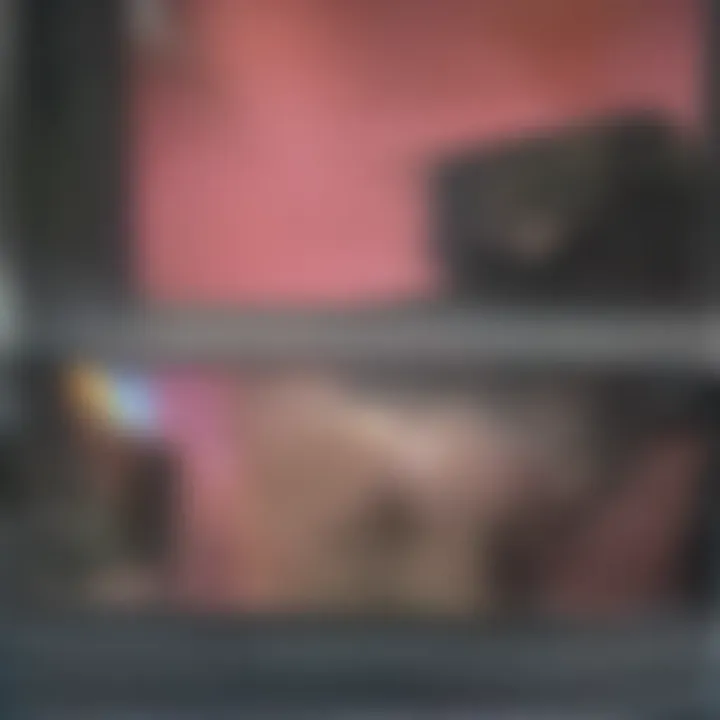
Clinical Adoption Barriers
Barriers to clinical adoption of AI solutions stem from multiple issues, including the lack of training for healthcare providers and skepticism regarding AI's reliability. Addressing these barriers is essential for successful AI integration in breast cancer management. The unique feature of clinical adoption barriers lies in their capability to impede the timely implementation of potentially beneficial technologies.
- Advantages: By recognizing and addressing these barriers, healthcare facilities can create a more conducive environment for AI technologies.
- Disadvantages: Overcoming these barriers often requires significant investment in training and infrastructure, which may be a hurdle for many institutions.
Ethical Considerations in AI Deployment
The incorporation of artificial intelligence in breast cancer detection and management raises critical ethical considerations. The use of AI not only enhances efficiency and accuracy but also brings along concerns that necessitate careful analysis. Addressing these concerns is essential as we seek to create a framework that balances technological benefits with ethical boundaries. In particular, it is necessary to focus on bias and fairness, as well as informed consent and regulation.
Bias and Fairness
Impact of Bias on Patient Outcomes
Bias within AI systems can lead to significant disparities in patient outcomes. Often, AI models are trained on datasets that do not reflect the diverse population they serve. This lack of representation can impact diagnosis and treatment decisions for certain demographics. A study found that AI algorithms underperformed in identifying breast cancer in women of color, leading to potential misdiagnosis. This situation illustrates how a bias in algorithms may perpetuate health inequities. Addressing bias is crucial for achieving fairness in AI applications in healthcare.
Strategies to Mitigate Bias
To combat bias in AI, several strategies can be employed. Ensuring diverse representation in training datasets is imperative for developing equitable algorithms. Techniques such as data augmentation can help diversify training samples. Implementing fairness-aware algorithms also provides ways to counteract inherent biases in AI. However, while these strategies can improve equity, they also introduce complexity in model training and might require additional resources to implement effectively. Thus, a balanced approach is necessary to ensure ethical AI use.
Informed Consent and Regulation
Patient Awareness of AI Involvement
Patient involvement in understanding AI's role in their healthcare journey is vital. Patients should be aware when AI is used in diagnostics or treatment recommendations. This transparency fosters trust and empowers patients to make informed decisions. However, the extent to which patients receive this information varies, leading to concerns about their ability to consent meaningfully. Improving patient education about AI technologies is necessary to support informed decision-making in clinical settings.
Regulatory Frameworks for AI Applications
An effective regulatory framework is essential for the safe deployment of AI in healthcare. Current regulations need to evolve to encompass the rapidly changing landscape of AI technologies. Defining guidelines for algorithm accountability, data usage, and patient privacy will provide a solid foundation for AI applications. However, there is a challenge in balancing innovation with regulation, as excessive regulation may stifle technological progress. Therefore, a flexible and adaptive regulatory approach is required to keep pace with advancements in AI.
Future Directions of AI in Breast Cancer Research
The future of artificial intelligence in breast cancer research offers a landscape filled with potential. As the technology evolves, opportunities for better detection and management continue to emerge. This section outlines significant elements, benefits, and considerations surrounding the future roles AI can play in this field.
Emerging Technologies and Innovations
Integration of AI with Genomics
The integration of AI with genomics is becoming increasingly important in the context of cancer research. By combining genetic information with AI algorithms, researchers can better understand tumor behavior and patient responses to treatment. This relationship allows for more personalized treatment plans. A key characteristic of this integration is its ability to analyze large datasets迅速, providing insights that would be difficult to achieve through traditional methods. One unique feature is the capability to identify genetic markers that predict individual responses to therapies. This advantage promotes precision medicine, helping oncologists tailor therapies that optimize outcomes. Nevertheless, collecting quality genomic data can be a challenge, and potential ethical implications regarding genetic privacy must be addressed.
Advancements in Telemedicine
Telemedicine has transformed the way healthcare is delivered, enhancing access to treatment for patients in remote areas. AI can play a crucial role in optimizing telemedicine services by providing virtual consultations, which are particularly beneficial for breast cancer survivors or patients unable to visit clinics frequently. A key characteristic of this advancement is its ability to facilitate remote monitoring, enabling healthcare providers to manage patient data effectively from afar. Unique features include chatbots and virtual assistants that help patients with their queries, reducing the workload on healthcare professionals. While telemedicine improves convenience and accessibility, it can also present challenges in terms of technology literacy among older patients and the need for secure data sharing.
Collaborative Efforts between AI and Healthcare Professionals
Multidisciplinary Teams in Cancer Care
Collaboration between AI systems and healthcare professionals is crucial for effective cancer care. Multidisciplinary teams, which include oncologists, radiologists, pathologists, and data scientists, work together to improve patient outcomes. A key characteristic of this model is the diversity of expertise, which enhances problem-solving capabilities. Each team member brings unique skills to the table, allowing for a more rounded approach to treatment. One unique advantage of this collaboration is the seamless integration of AI tools within existing workflows, providing decision support systems that assist clinicians in making informed choices. However, challenges can arise from silos in communication and the need for ongoing training to keep up with technological changes.
Training and Education for Practitioners
Training and education are vital in ensuring that healthcare professionals are prepared to leverage AI tools effectively in their practice. As more AI applications emerge, continuous education will help practitioners stay updated on best practices and new innovations. A key characteristic of education in this context is its focus on practical applications, rather than just theoretical knowledge. Unique features may include simulation-based training, where practitioners can practice using AI systems in a controlled environment. This training has the potential to improve confidence and proficiency in using AI, ultimately leading to better patient care. On the downside, not all institutions may have the resources for comprehensive training programs, leading to gaps in knowledge among professionals.
AI continues to reshape the landscape of breast cancer detection and management, emphasizing the importance of integrating technology with collaborative human effort.
As we look ahead, the interplay between emerging technologies, team collaborations, and education will be paramount in harnessing the full potential of AI to revolutionize breast cancer care.
End
Recapitulating the Potential of AI
Artificial intelligence signifies a transformative force within the realm of breast cancer detection and management. It offers enhanced accuracy in diagnostics, contributing to earlier detection rates and subsequently improving treatment success. Furthermore, AI systems are capable of processing vast amounts of data far quicker than human counterparts, thereby reducing the time between diagnosis and intervention.
In sum, AI not only streamlines workflows for healthcare providers but also ensures that personalized treatment protocols are more readily developed. These advancements are essential in combating breast cancer effectively and efficiently. The potential for AI to assimilate data from genomics and other emerging technologies is significant. This could revolutionize patient-tailored therapies and predictive analytics in oncology.
Final Thoughts on Future Integration
Looking towards the future, the integration of AI in breast cancer management appears promising, yet challenges remain. The collaboration of multidisciplinary teams is paramount in navigating the complexities associated with patient care. Training and education for healthcare practitioners on the functionalities of AI could enhance its application in practical settings.
Moreover, as AI continues to evolve, it will be crucial to remain vigilant about ethical considerations and patient privacy. Understanding how AI tools operate and maintaining transparency in decision-making processes will build trust among healthcare professionals and patients alike. As the landscape of medical technology shifts, the continued evolution of AI in breast cancer management could redefine best practices, leading to improved outcomes and survival rates for patients.