Navigating Challenges of AI in Healthcare
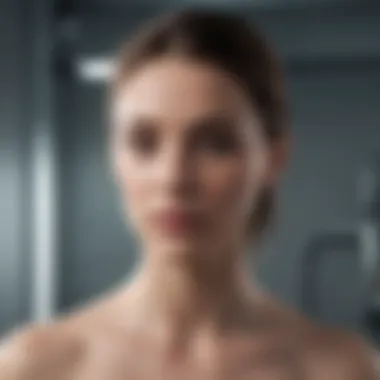
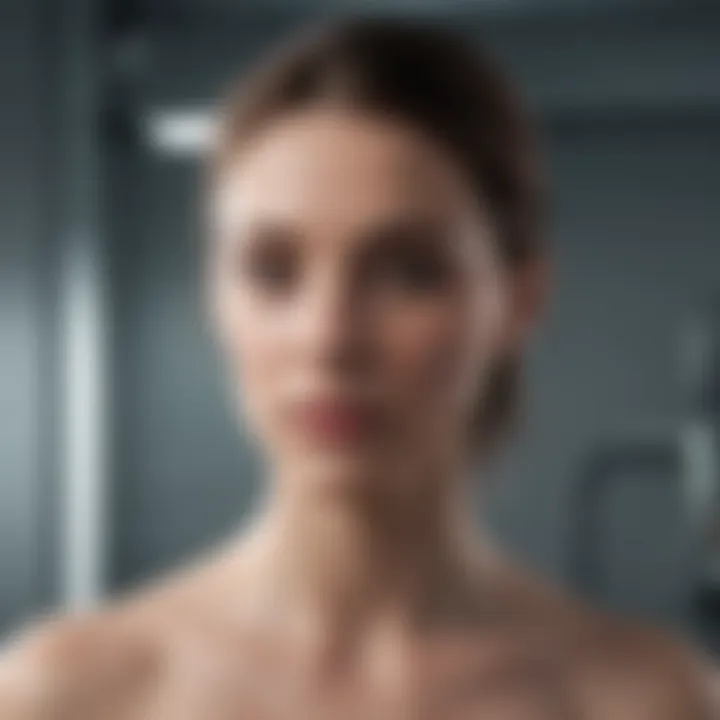
Intro
As we plunge into this complex landscape of healthcare, artificial intelligence looms large with its promise of revolutionary change. However, the integration of AI into medical practices isn’t all sunshine and roses. Beneath the glittering surface lies a web of challenges, ethical quandaries, and potential pitfalls that need careful scrutiny. To truly understand the implications of AI in healthcare, we must peel back the layers of this intricate issue, exploring how it alters patient care, impacts workforce dynamics, and casts shadows on health equity.
In this exploration, we will delve into the pressing matters of data privacy, algorithmic biases, and the labyrinthine regulatory frameworks surrounding AI technologies. Let’s not forget the human element—how do these innovations shape the patient experience, and what do they mean for the professionals on the front lines? By dissecting these concerns, we aim to equip stakeholders, including students, researchers, and healthcare professionals, with the knowledge to navigate this evolving field effectively.
Research Overview
Key Findings
The deployment of AI in healthcare has become more prevalent, but it does not come without its challenges. Key findings from recent studies indicate that:
- Ethical dilemmas reign supreme: The moral implications of AI decisions in patient care are contentious. For instance, should AI systems prioritize efficiency over individualized patient care?
- Data privacy concerns: With vast amounts of personal health data at stake, ensuring privacy is paramount. Data breaches can lead to severe consequences, including identity theft and loss of patient trust.
- Algorithmic bias: Not all AI systems are created equal. Bias in training data can result in AI systems that inadvertently favor one demographic over another, exacerbating health disparities.
Study Methodology
To gain a comprehensive understanding of these issues, a mixed-methods approach was adopted in recent research studies. Both qualitative interviews with healthcare professionals and quantitative surveys provide a dual lens through which to view the impacts of AI. This methodology allows for a richer understanding of the practical challenges faced by practitioners on the ground.
Background and Context
Historical Background
The interaction between technology and medicine is not new. From the stethoscope to robotic surgery, healthcare has continually evolved. Yet, the introduction of AI marks a significant turnaround. AI entered public conversation in the healthcare arena in the early 2010s, with initial efforts focusing on diagnostic tools. Over the years, it has surged beyond diagnostics, now encroaching on areas such as treatment recommendations and patient management.
Current Trends in the Field
Today, various companies are making strides in AI applications. Some notable trends include:
- Telehealth integration: As a result of the COVID-19 pandemic, telemedicine solutions utilizing AI are becoming commonplace.
- Predictive analytics: AI is increasingly deployed to analyze trends and predict healthcare needs, influencing everything from resource allocation to staffing.
- Patient engagement tools: Systems designed to interact directly with patients, guiding them through their treatment journeys, are gaining traction.
"The essence of AI's potential in healthcare lies in its ability to process and analyze vast datasets at speeds unattainable by humans."
As these trends continue to flourish, challenges must also be addressed. Balancing innovation with ethical standards and ensuring equitable access to these technologies will require ongoing dialogue and collaboration among all stakeholders.
Prolusion to AI in Healthcare
Artificial Intelligence (AI) is gradually weaving its way into the fabric of healthcare, promising transformative changes that could enhance patient outcomes and streamline medical processes. The infusion of AI into this sector is not just a trend; it's a shift toward a data-driven approach aimed at identifying patterns, optimizing workflows, and personalizing care. However, as with any significant change, there are challenges and concerns that must be navigated to ensure that the benefits are realized.
Understanding the importance of AI in healthcare requires a closer look at how it can improve efficiency and accuracy in diagnosis and treatment. For instance, AI systems can analyze medical imaging far quicker and sometimes more accurately than radiologists can. The implications are profound; with AI as a valuable tool in their arsenal, medical professionals can make better-informed decisions, which could mean the difference between life and death.
Yet, the integration of AI also demands careful consideration of ethical, practical, and social implications. These can often overshadow its positive aspects. For instance, how do we ensure that these complex algorithms are transparent and accountable? What about the prospect of bias creeping into AI systems and affecting patient care? These significant questions highlight why a comprehensive understanding of AI's role within healthcare is critical.
"AI in healthcare isn’t just about technology; it’s about augmenting human capabilities while mitigating risks."
In light of these challenges, this article aims to explore not only the current landscape of AI in healthcare but also the multifaceted concerns that accompany its implementation. As we delve into the depths of this discussion, it becomes evident that while AI possesses the power to revolutionize healthcare, it must be approached with caution. Understanding both the benefits and challenges is essential for steering these innovations toward aiding humanity rather than creating new dilemmas.
Understanding AI Technology
At its core, AI is a discipline of computer science that simulates human intelligence processes, encompassing learning, reasoning, and self-correction. This technology is not a solitary entity; it encompasses various approaches, including machine learning, natural language processing, and neural networks. The aim is to create systems capable of performing tasks that usually require human intellect.
For example, a simple AI-based chatbot can triage patient queries by analyzing symptoms and recommending next steps. Implementations in diagnostic medicine are notably more complex, involving massive datasets from which AI can learn to recognize patterns often invisible to the human eye. The advancement in computational power and vast amounts of healthcare data available today mean that AI can handle an exponential increase in complexity.
Current Applications
The applications of AI in healthcare are as diverse as they are impactful. Some key areas where AI is currently utilized include:
- Medical Imaging: AI algorithms can scan and interpret MRI, CT, and X-ray images with high precision, often detecting anomalies that human radiologists might overlook. This technology improves diagnostic speed and accuracy.
- Predictive Analytics: Leveraging historical patient data helps in predicting outcomes. Hospitals use AI to forecast patient admissions, which can optimize staffing and resource allocation.
- Personalized Medicine: AI can analyze genetic data to help tailor treatment plans specific to the individual characteristics of each patient, moving away from the one-size-fits-all approach.
- Robotics in Surgery: Robotic surgical systems can perform complex procedures with enhanced precision, reducing recovery times for patients and improving surgical outcomes.
- Virtual Health Assistants: With capabilities to engage, remind patients for medication, and provide health management advice, these AI-driven interfaces support both patients and healthcare providers in daily healthcare management.
As AI continues to evolve, these applications are likely to expand, paving the way for innovative solutions in healthcare. However, the excitement surrounding these advancements must also be balanced with a keen awareness of the accompanying challenges and concerns.
Ethical Concerns
The integration of Artificial Intelligence (AI) in healthcare prompts a myriad of ethical concerns that must be addressed to ensure the technology evolves responsibly. As the medical landscape changes, issues surrounding patient rights, decision-making authority, and the very nature of doctor-patient relationships take center stage. Navigating these ethical dilemmas becomes crucial not just for the industry but also for the patients who depend on these innovations for their well-being.
Patient Consent
Patient consent is a cornerstone of ethical medical practice, and AI poses unique challenges in this regard. With algorithms analyzing vast amounts of data, the question arises: how do we ensure that patients fully understand what they are consenting to when their health information is used by AI technologies?
- The complexity of AI systems could lead to situations where patients might not comprehend the extent to which their data is leveraged.
- Furthermore, the speed at which information is processed and decisions made by AI may outpace traditional consent protocols, raising questions about the ongoing need for consent regarding the continuous use of patient data.
- A significant concern is whether blanket consent, often obtained during initial consultations, remains valid as AI technology and data handling evolve. Such practices need careful scrutiny to uphold patient autonomy while allowing for technological advancement.
These considerations underscore a pivotal need for transparency. Emphasizing clear communication and allowing patients to opt-in or opt-out could enhance trust and ensure that individuals retain control over their personal health information.
Patients must not just consent; they must comprehend what they agree to in the ever-evolving AI landscape.
Autonomy and Decision-Making
The autonomy of patients and the decision-making processes in healthcare are being reshaped by AI systems. As these technologies become more sophisticated, they begin to influence clinical decisions traditionally reserved for caregivers. This shift raises essential questions about the balance between human judgment and machine recommendations.
- There’s often a blurred line where AI input goes from assistive to prescriptive. Medical professionals may find themselves relying on AI recommendations more heavily, which could undermine their own expertise.
- Additionally, the potential for overreliance on AI could disempower patients, turning them from active participants in their health choices into passive recipients of medical directives.
- In this dynamic, ensuring that patients have a voice in their care remains paramount. To facilitate this, it might be beneficial to establish robust frameworks where AI recommendations are presented as just one component of a broader clinical decision-making process.
Ultimately, a balance must be struck. AI should serve as an aid, enhancing the decisions made by trained professionals, rather than replacing the nuanced human interactions that form the backbone of effective patient care.
Data Privacy Issues
Data privacy in healthcare is a critical concern, especially with the rising tide of Artificial Intelligence. As healthcare systems increasingly rely on AI to drive efficiencies and improve patient care, significant amounts of sensitive health data are generated, processed, and stored. This situation raises crucial questions about how that data is protected and who has access to it.
With a wealth of data comes the responsibility to handle it with care. Failure to prioritize data privacy can lead to severe consequences, not only for patients but also for healthcare providers.
Compliance with Regulations
Compliance with regulations is one of the foremost aspects of data privacy. Government regulations like the Health Insurance Portability and Accountability Act (HIPAA) in the United States require healthcare organizations to implement stringent data protection measures. These rules aim to safeguard patient information against unauthorized access and data breaches.
In many cases, achieving compliance is not a straightforward task. For instance, implementing necessary security protocols can be time-consuming and costly. Organizations must also continuously update their practices to adhere to evolving legislation. Moreover, confusion can arise when integrating AI systems that manipulate data in new ways. Public perception around compliance affects whether patients trust healthcare systems to protect their information.
"Without the proper safeguards in place, the innovations brought by AI could compromise the very trust that patients place in healthcare providers."
Risks of Data Breaches
The risk of data breaches is another pressing concern in AI implementation. Cybersecurity attacks are on the increase, and healthcare is no exception. A single data breach can expose countless records, putting patients at risk for identity theft or fraud. For example, incidents involving ransomware attacks on hospitals have made headlines, with criminals holding critical data hostage until a ransom is paid.
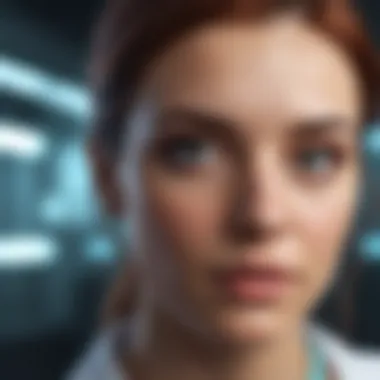
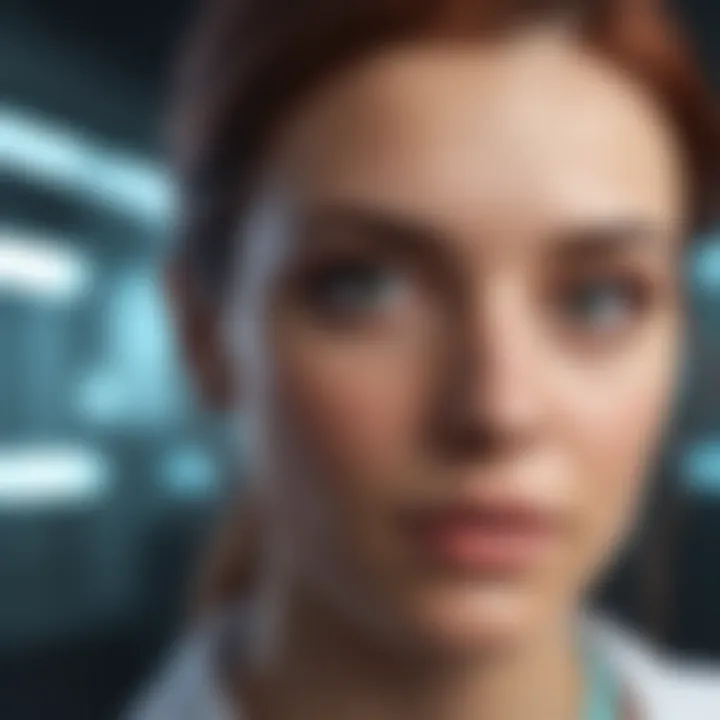
When examining the vulnerabilities inherent in AI systems, the complexity of these algorithms can create unforeseen gaps in security. A lack of robust encryption measures during data transmission, or inadequate training for staff on potential risks, can lead to large-scale security failures. As patients become aware of these risks, their willingness to share sensitive health information may decline, thereby impacting the effectiveness of AI systems designed to learn from vast datasets.
In the end, the data privacy issues surrounding AI in healthcare are not just about compliance or protecting sensitive information; they represent a complex interplay between regulatory frameworks and the evolving landscape of technology. Finding a balance between innovation and security is paramount.
Algorithmic Bias and Fairness
As we seriously delve into the realm of AI in healthcare, one of the most pressing concerns arises from the notion of algorithmic bias and fairness. This topic isn't just an academic exercise; it goes right to the heart of how AI presents solutions or problems in a medical setting. Given the sensitive nature of healthcare, any misalignment in algorithms can lead to devastating consequences. Grasping the importance of algorithmic bias is key to understanding its potential implications on patient care.
It’s essential to recognize that AI systems are only as good as the data they are trained on.
Sources of Bias in AI Systems
Bias doesn’t appear out of thin air; it’s often rooted in the datasets that serve as the foundation for these AI systems. Several factors contribute to bias in AI:
- Historical disparities: Many datasets reflect previous inequalities. For instance, if historical data highlights a specific demographic's health outcomes over others, the algorithm might inadvertently favor that group.
- Sampling issues: A lack of diverse representation in the datasets can skew results. If a dataset is predominantly made up of one racial or socioeconomic group, AI decisions could favor that group, leaving others at a disadvantage.
- Feature selection: When data scientists decide which features to include in an algorithm, their choices can introduce bias. For example, excluding certain health factors or social determinants can lead to unfair outcomes.
- Design and implementation: The way algorithms are constructed matters. Subtle decisions in coding can result in disparities in how algorithms process data.
Recognizing these sources of bias is just the first step. Identifying and working to mitigate them is what healthcare practitioners and tech developers should prioritize.
Impact on Patient Outcomes
The ramifications of algorithmic bias are far-reaching. In healthcare, biased algorithms can influence clinical decisions, resource allocation, and even treatment recommendations. Let’s break this down:
- Diagnosis accuracy: If an AI system is designed based on biased data, the accuracy of diagnoses can suffer greatly. For example, an algorithm trained primarily on data from one demographic may misinterpret symptoms in others, potentially leading to misdiagnosis.
- Treatment equity: When treatment recommendations lean toward one group because of biased algorithms, it creates unequal treatment access. Imagine a scenario where a heart condition treatment is recommended primarily based on a model learned from a certain ethnicity; others may not receive necessary interventions.
- Trust in the healthcare system: Continuous exposure to biased systems can erode public trust in healthcare. If marginalized communities feel that the technology doesn't serve them fairly, they may distrust healthcare solutions altogether.
"The implications of algorithmic bias can ripple through healthcare systems, altering both trust and care quality across diverse populations."
In the end, addressing bias in AI is not just about correcting a technical error; it’s about ensuring health equity and fostering trust within the healthcare system. As we embrace AI technology, the onus remains on us to scrutinize and enhance these systems to safeguard against inequities that could adversely affect patient outcomes.
Regulatory Challenges
Incorporating artificial intelligence into healthcare is not just about technology; it involves navigating a labyrinth of regulatory requirements that can either pave the way for success or hold back innovation. Regulatory challenges are critical because they ensure the safety and effectiveness of AI applications. This oversight is essential for maintaining public trust and ensuring that health technologies truly serve their purpose. Numerous regulations govern medical devices, data use, and patient safety; thus, understanding how AI fits into these frameworks is vital.
Frameworks for Approval
When discussing frameworks for approval, it is crucial to understand how AI applications are categorized and evaluated by regulatory bodies. In the United States, for example, the Food and Drug Administration (FDA) has developed pathways to assess AI tools under the same lens as medical devices, which includes rigorous testing for safety and efficacy. This approval process can be lengthy and complex, often requiring extensive clinical trials to validate AI algorithms.
A possible challenge in this framework is the pace of technological advancement. While AI systems evolve quickly, regulatory processes tend to lag. This disparity can deter developers from investing in AI healthcare solutions. To help bridge this gap, several regulatory authorities have started offering expedited pathways and guidance documents tailored for AI. However, developers still must navigate complicated regulations that vary greatly across different countries and regions.
- Key Considerations for Framework Approval:
- Understanding specific regulatory requirements for different types of AI products
- Preparing comprehensive documentation for submission
- Conducting clinical trials that meet both regulatory and scientific standards
Evolving Standards
Regulation doesn’t remain static. It evolves along with technology, and that adaptability is both a necessity and a challenge. Returning to the FDA as an example, the agency is currently refining its guidance to better accommodate the unique characteristics of AI systems, particularly those utilizing machine learning. These evolving standards are essential for maintaining public safety while also fostering innovation in healthcare.
One significant aspect of evolving standards is performance monitoring after AI technologies are deployed. Traditional regulations may regard products as static once approved, but AI can change its behavior based on new data. This necessitates a system for ongoing evaluation and post-market surveillance that can quickly identify any adverse outcomes or unintended consequences.
- Challenges of Evolving Standards:
- Keeping up-to-date with continuous developments in AI technology
- Implementing robust post-market surveillance mechanisms
- Ensuring stakeholders, from developers to healthcare providers, understand new standards
"Navigating regulatory challenges is not simply an obstacle; it's also an opportunity for stakeholders to define and refine how we ensure AI enhances healthcare effectively and ethically."
Thus, the landscape of regulatory challenges is both complex and evolving, demanding vigilance from every player in the healthcare sector. As we strive to harness AI technologies, clear guidelines and collaborative frameworks will be paramount to safeguard patient welfare while unleashing the full potential of innovation.
Impact on Patient Care
The integration of Artificial Intelligence in healthcare isn't just a fanciful concept; it has real implications on patient care. When we talk about the impact on patient care, we're not only looking at how AI improves operations but also at how it influences the patient experience, clinical outcomes, and the very fabric of medical practice. As technology becomes a more integral part of healthcare systems, understanding its effects on patients is paramount.
Quality of Diagnosis
In the realm of medical diagnosis, precision and speed are golden. AI has stepped into this arena, offering tools that assist physicians in diagnosing conditions with remarkable accuracy. For example, AI algorithms can analyze medical imaging faster than ever before—consider the case of detecting tumors in MRIs or CT scans. These tools sift through vast amounts of data, finding patterns that may elude human eyes. The diagnostic accuracy potentially improves, resulting in earlier detections and better treatment outcomes.
However, this doesn't come without caveats. Though AI can enhance diagnostic capabilities, reliance solely on algorithms raises questions about their interpretative finesse. A misdiagnosis by an AI, however infrequent, could have devastating impacts on a patient's health. Hence, clinicians must integrate AI findings with their clinical acumen; it's not simply about the numbers but the context of patient history and symptoms. Additionally, a systematic approach for validating AI tools before deployment is vital, ensuring they can be trusted in real-life scenarios.
"AI doesn’t replace the doctor; it enhances the doctor’s ability to serve patients better."
Patient-Provider Relationships
Relationships in healthcare are built on trust, communication, and empathy. The introduction of AI can both help and hinder these dynamics. On one hand, AI tools like chatbots and virtual health assistants can make appointment scheduling easier, provide quick responses to patient queries, and enable self-monitoring. These tools enhance accessibility, putting information at patients' fingertips whenever they need it.
However, there’s a double-edged sword here. Over-reliance on technology can distance the patient from the provider. Patients may feel like they are just another data point in a vast AI system rather than a unique individual with specific needs. Personal connections are key in healthcare—doctors who know their patients' histories, worries, and personalities can deliver care tailored to those individuals. Striking a balance between utilizing AI effectively and maintaining the human touch in healthcare is essential for cultivating trust.
In summary, the impact of AI on patient care can be seen as a complex tapestry. Quality diagnoses can lead to improved outcomes but must be interpreted wisely. Meanwhile, patient-provider relationships demand careful nurturing as technology becomes more prevalent. It's a delicate dance of progress and preservation. Healthy dialogues around these topics are crucial for shaping the future of healthcare in a way that aligns technological advancements with patient needs.
Workforce Dynamics
In the landscape of healthcare, the introduction of artificial intelligence brings not just technological advancements but also a transformation in workforce dynamics. This area is particularly significant because the integration of AI into medical practices impacts not only how care is delivered but also the very structure of the healthcare workforce itself. Understanding this dynamic is essential for all stakeholders—be it healthcare providers, policy makers, or educators—who must navigate the changes that AI technology heralds.
Job Displacement Concerns
The discussion surrounding job displacement is often laden with anxiety. Many fear that AI will replace human roles, particularly in areas where repetitive tasks are prevalent. For instance, in radiology, machines now can analyze images with astonishing speed and sometimes even greater accuracy than a human radiologist. While some jobs may indeed vanish, there is the possibility of a paradigm shift rather than outright replacement. This shift might see healthcare professionals moving from administrative or low-skill activities to roles that require higher cognitive functions and interpersonal skills.
Here are several points to consider regarding job displacement concerns:
- Nature of Work Transformation: Jobs may change from manual tasks to more analytical roles, placing emphasis on human judgment, ethics, and patient interaction.
- Reskilling Opportunities: As AI takes over certain tasks, there is a pull for current workers to acquire new skills that align with evolving job roles in healthcare.
- Increased Demand for Care: With an aging population and growing health complexities, the demand for healthcare services is on the rise, potentially creating new roles rather than diminishing them.
"While AI may alter the employment landscape, it does not inherently spell doom for jobs in healthcare; instead, it compels a reevaluation of the skill sets required to thrive."
Need for New Skill Sets
In conjunction with job displacement, there is a pressing necessity for new skill sets among healthcare workers. As AI systems become more embedded in daily operations, healthcare professionals will need to adapt to using these advanced tools effectively. A study by the World Economic Forum indicated that by 2025, over 85 million jobs may be displaced, but at the same time, 97 million new roles may emerge that are more suited to the new division of labor.
Some essential skills now at the forefront include:
- Data Interpretation: The ability to understand and analyze data outputs from AI systems will be critical. This includes familiarity with health informatics.
- Technological Proficiency: There’s a growing demand for hands-on proficiency with AI technologies, such as machine learning algorithms, data analytics software, and telemedicine platforms.
- Interdisciplinary Collaboration: Today’s healthcare requires collaboration across various disciplines, integrating skills from areas such as IT, ethics, and behavioral sciences.
- Emotional Intelligence: As machines take over many technical tasks, the human touch—emotional intelligence and communication—will become even more vital in patient care.
In summary, workforce dynamics in the implementation of AI in healthcare presents both challenges and opportunities. It's less about machines replacing humans and more about how humans can evolve to harness AI as part of their repertoire in providing quality health care.
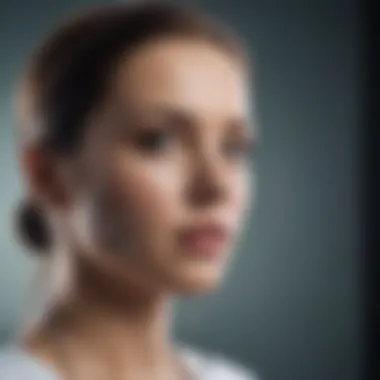
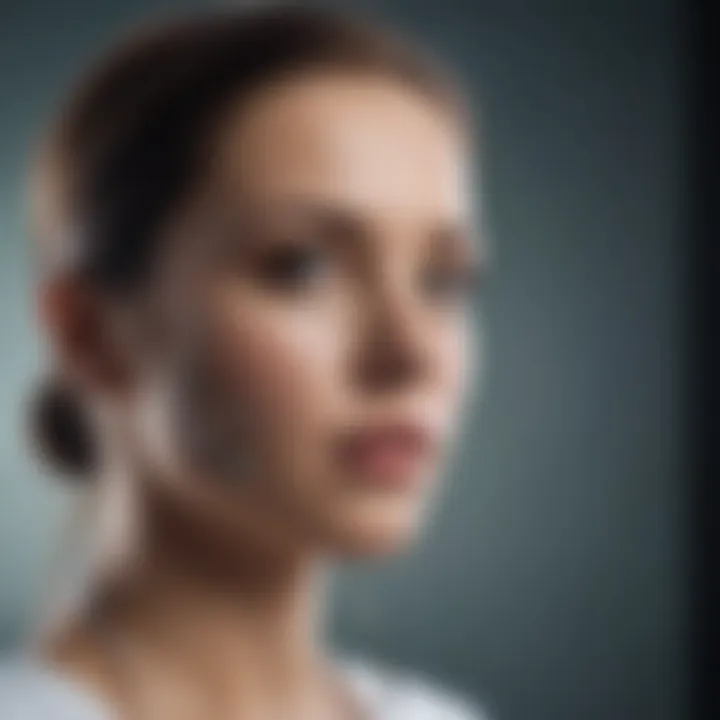
Health Inequality
Health inequality is a pressing concern that cannot be overlooked when discussing the integration of artificial intelligence in healthcare. As AI technologies advance, they present significant opportunities for improving patient outcomes and streamlining healthcare services. However, they also run the risk of exacerbating existing health disparities if equitable access and distribution are not prioritized. It's crucial to dissect the nuances within this topic to ensure that advancements serve all segments of the population rather than a privileged few.
Access to AI Technologies
Access to AI technologies in healthcare is often a tale of two cities. On one hand, urban areas are witnessing remarkable advancements with the rollout of sophisticated AI applications that assist in diagnostics, treatment planning, and patient management. On the other hand, rural and underserved communities remain largely in the dark, struggling to receive even basic healthcare services.
- Variability in Infrastructure: Many healthcare institutions lack the necessary infrastructure to implement AI systems effectively. High-speed internet, advanced computational resources, and training for healthcare professionals are fundamental to harnessing AI’s full benefits. Unfortunately, these resources are not uniformly available across different regions.
- Financial Barriers: Implementing AI-driven solutions often requires considerable investment. As a result, hospitals with tight budgets or those in less affluent areas may lag behind their more prosperous counterparts.
- Training and Knowledge Gaps: Without proper training, healthcare workers may be ill-prepared to utilize AI technologies. This knowledge gap affects who can confidently integrate these tools into practical healthcare settings, fostering additional divides.
The contrasting access to AI tools highlights the urgent need for targeted investments in infrastructure and education, especially in marginalized regions.
Disparities in Health Outcomes
Even if access to AI technologies were equitable, disparities in health outcomes still loom large. Various factors prohibit equal benefits from AI advancements:
- Demographic Factors: Racial, ethnic, and socioeconomic factors can lead to uneven health outcomes. Algorithms that lack inclusivity can perpetuate or even intensify these disparities. When AI systems are trained primarily on data from one demographic, the underlying assumptions can lead to misdiagnoses or inadequate treatment recommendations for underrepresented groups.
- Resource Allocation: In many healthcare ecosystems, AI might be better utilized in affluent areas—where hospitals are better staffed and equipped—while ignoring poorer populations. This prompts a cycle where those who need assistance the most continue to be marginalized, deepening existing inequalities.
- Public Trust Issues: If individuals from certain communities do not trust the technology or the healthcare system in general, they may be less likely to engage with AI-driven treatment options. This hesitation can be particularly acute in populations that have historically experienced discrimination or neglect.
"As AI technologies proliferate, ensuring broad equitable access will determine whether these innovations serve as tools for universal improvement or serve to deepen the chasms of inequity."
In summary, both access to AI technologies and disparities in health outcomes are integral to understanding health inequality in the context of AI in healthcare. Addressing these issues requires a multi-faceted approach that not only promotes equitable access but also fosters inclusive algorithms and community trust. These steps are essential to turn AI’s potential into a reality that uplifts all individuals, regardless of their socio-economic status or geographical location.
Public Perception
Understanding how the public perceives AI in healthcare is crucial for successful implementation and acceptance. The growing complexity of AI systems is paralleled by an evolving understanding among patients, healthcare professionals, and regulatory bodies. Public perception hinges on trust, awareness, and engagement with these technologies.
Trust in AI Solutions
Trust forms the bedrock of acceptance for any new technology, particularly in healthcare where lives are at stake. When patients and healthcare providers lack faith in AI solutions, the integration of these technologies faces significant roadblocks. The skepticism often arises from a lack of understanding, potential backlash from past failures or privacy concerns.
For example, consider an AI diagnostic tool designed to detect diseases earlier than traditional methods. If patients feel that their sensitive information could be mishandled or that a machine lacks the empathy necessary for human judgment, they are less likely to trust the tool’s recommendations. Building this trust requires transparency about data usage, algorithmic processes, and the overall intent of AI systems. Regular communication about the successes and limitations of AI can help demystify the technology and cultivate a more trusting environment.
It's essential to engage patients in conversations about what AI can and cannot do. They need to be reassured that these tools are designed to complement, not replace, human expertise. A survey conducted by Health Affairs found that 58% of respondents expressed hesitancy surrounding AI in medical decision-making, emphasizing the need for public confidence in AI applications.
Awareness and Understanding
Awareness about AI technologies plays a key role in shaping public perception. Generally, people tend not to trust what they do not understand. The intricate workings of AI, such as machine learning or natural language processing, often remain opaque to the average person.
To bridge this gap, educational campaigns are indispensable. Here are a few methods to enhance awareness:
- Workshops and Seminars: Hosting community events where experts explain AI’s role in healthcare can dispel myths and promote informed discussions.
- Social Media and Content Campaigns: Well-crafted informational posts, videos, and articles on platforms like Facebook and Reddit can reach a broader audience and drive engagement.
- Collaboration with Trustworthy Organizations: Partnering with established health organizations can lend credibility to awareness efforts, making the information more palatable.
Fostering a deeper understanding requires addressing common apprehensions head-on. Many individuals worry about job displacement within the healthcare sector or potential biases in AI systems. These concerns need careful navigation, ensuring that information is not only accurate but framed in a way that fosters dialogue rather than fear.
Moreover, when healthcare professionals are well-informed about AI, they can serve as ambassadors to help educate the public. A doctor who understands the intricacies of AI can reassure patients, answering their questions in a relatable manner that fosters connection over apprehension.
"Engaging the public and maintaining transparency in AI’s functionalities can turn skepticism into trust, paving the way for smoother AI integration in healthcare."
Overall, the interplay between trust and awareness underscores the importance of public perception in the successful implementation of AI in healthcare. Keeping the conversation going, addressing concerns openly, and clarifying the objectives of AI technologies can lead to a more informed and accepting public.
Establishing this trust can unlock the potential for AI, transforming healthcare's landscape into one where both patients and providers can confidently seek and leverage innovative solutions.
Future Prospects
The incorporation of Artificial Intelligence (AI) in the healthcare sector is undeniably on the rise. The future prospects of AI technology in healthcare present a dual-faceted narrative—one filled with transformative potential as well as significant challenges. Understanding these prospects is necessary to grasp how AI may redefine how healthcare is delivered, patient outcomes are enhanced, and operational efficiencies are realized across the industry.
AI holds the promise of revolutionizing patient care. With advancements in algorithms, AI systems may provide diagnostic support that is not just faster but also more accurate than traditional methods. The tools might analyze vast datasets from medical records or imaging sources to identify patterns and anomalies that can lead to earlier intervention strategies. For instance, in oncology, AI can flag irregularities in imaging scans that a human eye might miss, thus allowing healthcare providers to tailor treatment sooner.
However, these innovations prompt concerned conversations about ethical considerations, data privacy, and the overall reliability of AI systems.
Innovative Solutions
One key element that comes to the forefront under the umbrella of future prospects is the development of innovative solutions tailored to the unique characteristics of healthcare. It is vital to create AI applications that not only enhance diagnostics but also address specific clinical needs.
Rather than a one-size-fits-all approach, AI solutions must be adaptable and patient-centric. For instance, personalized medicine powered by AI could analyze genetic data and lifestyle factors to suggest individualized treatment plans. AI-powered tools could aid healthcare professionals in remote areas, where access to specialists is limited, by offering real-time decision support based on current case scenarios.
Moreover, the convergence of AI with other technologies such as blockchain can enhance security and trust in AI applications. By allowing secure sharing of patient data, blockchain aids in addressing privacy concerns while maintaining the integrity of information.
Some of the promising innovative solutions include:
- Predictive Analytics: Tools that foresee disease outbreaks or treatment outcomes.
- Virtual Health Assistants: AI-driven chatbots that provide immediate medical advice based on symptoms.
- Enhanced Imaging Techniques: AI systems that improve the resolution and accuracy of medical imaging.
This forward-thinking perspective emphasizes that innovation should consider not only technical advancement but also ethical deployment, providing sustainable pathways for AI integration in healthcare.
Integration Strategies
The road ahead for AI in healthcare involves well-planned integration strategies, which are essential for success. Without a coherent approach to integration, even the most innovative solutions can fall short of their potential. Developing comprehensive strategies involves several key considerations that cater to both technical and operational facets.
Incorporating AI should also involve a collaborative commitment from all stakeholders. The integration process demands close collaboration between technologists and healthcare professionals to facilitate smoother implementation in clinical settings. The real-world applicability of AI systems can thus improve dramatically when both parties work together.
A few vital integration strategies include:
- Training and Education: Developing ongoing training programs for healthcare professionals to familiarize them with AI tools and benefits.
- Pilot Programs: Initiating small-scale implementations to test AI solutions and garner feedback before larger deployment.
- Interoperability: Ensuring that AI systems can work fluently across various existing healthcare systems is critical to prevent data silos and enhance usability.
The future of AI in healthcare is not just about technological advancements but also about the methods of integration that embrace collaboration, adaptability, and user-friendliness.
"The potential for AI to enhance the healthcare landscape is immense, yet it is our strategies for integration that will determine its success and ethical deployment."
In summary, the future prospects of AI in healthcare showcase how much there is to gain through innovative solutions and strategic integration. Both are imperative for reaping the full scope of benefits that AI promises to deliver in the medical field.
Interdisciplinary Approaches
In the journey of integrating Artificial Intelligence in healthcare, employing interdisciplinary approaches emerges as a vital cog in the machine. Various fields must come together, combining their expertise, to navigate the choppy waters of AI implementation. This collaboration holds profound weight in ensuring that AI technologies serve their intended purpose effectively and ethically. Health professionals, data scientists, ethicists, and engineers, when pooled together, form a robust framework that can tackle the multifaceted challenges presented by AI.
Collaboration Between Fields
When different disciplines converge, magic can happen. The merging of healthcare with artificial intelligence is not just about tech meeting medicine; it's about bridging knowledge gaps and maximizing opportunities for improvement across the board.
For instance, healthcare advocates can work side by side with data scientists to fine-tune algorithms that aid in diagnosis by providing contextual knowledge, while technical experts ensure that these tools adhere to the highest standards of usability and safety. This collaboration could streamline processes that have long been bogged down by bureaucracy and inefficiency.
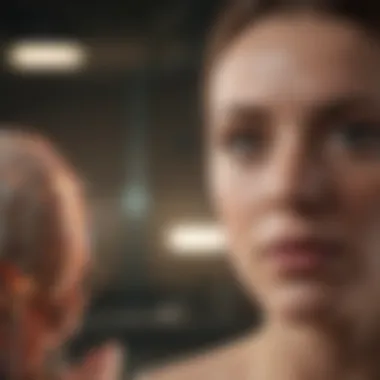
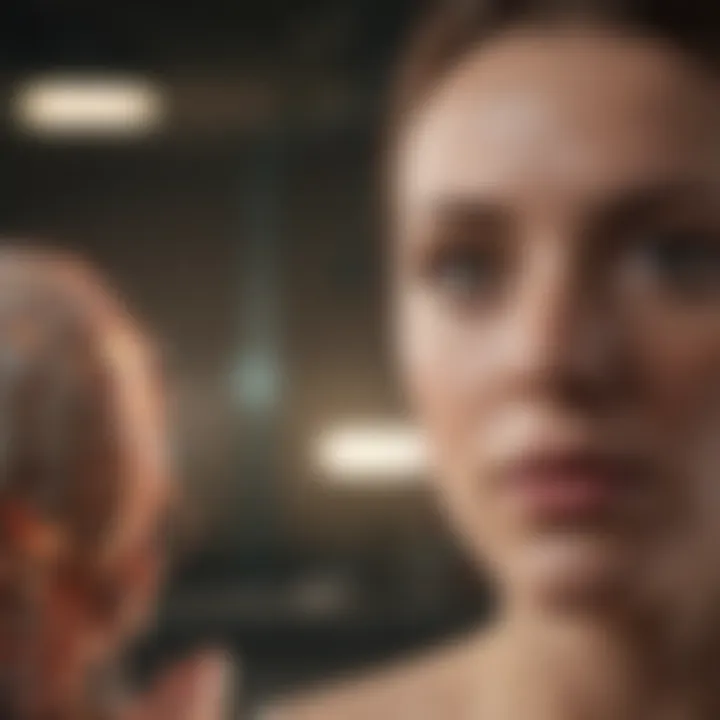
Furthermore, engaging ethicists in the conversation ensures that real-world impacts are kept in check. They can actively participate in shaping guidelines to avoid pitfalls like bias and ensure patient consent is respected. In short, by working together, these fields create a synergistic effect, leading to innovations that wouldn't be possible in isolated silos.
"Interdisciplinary collaboration not only enriches AI solutions, but also enhances their acceptance in healthcare settings, addressing concerns before they become crises."
Enhancing Research Outcomes
When talking about interdisciplinarity, a notable benefit is seen in research outcomes. The outcomes of research projects can significantly improve when there’s a multi-faceted approach involved. For instance, consider a study focused on using AI to predict patient outcomes post-surgery. If only surgeons were involved, the insights gained may be too narrow. However, if researchers from social sciences, computer science, and clinical practice all contribute, the findings could cover a broader spectrum, accounting for patient psychology and social determinants of health.
Some key ways interdisciplinary methods can enhance research outcomes include:
- Diverse Perspectives: Varied professional backgrounds lead to a more comprehensive analysis of problems.
- Innovative Methodologies: Different disciplines often utilize unique methods, which can invigorate research designs and lead to new discoveries.
- Broader Impact: Research findings can be more applicable and relevant to a diverse patient population when multiple viewpoints are included.
In tackling the challenges and concerns surrounding AI in healthcare, fostering interdisciplinary collaboration isn't just beneficial—it is essential. If stakeholders from various backgrounds join forces, AI tools can be more resilient, practical, and mindful of the diverse needs they aim to serve.
Technological Limitations
The integration of Artificial Intelligence into healthcare brings along a host of technological limitations that need addressing. These limitations can significantly affect not only the implementation process but also the broader impact of AI on patient care and health management. Understanding these challenges is crucial for stakeholders involved in AI developments, as legacy systems and inadequately designed interfaces can limit the effectiveness and usability of these advanced technologies.
User Interface Challenges
User interfaces (UI) serve as the bridge between human users and complex AI systems. Despite advancements in AI technology, the design of user interfaces remains a critical barrier. A poorly designed interface can confuse healthcare providers, detracting from the core functionality of AI tools. If the interface does not align well with the workflows of health professionals, it may discourage usage.
The importance of intuitive design cannot be overstated in this environment. As healthcare systems are already overloaded, introducing a complicated AI tool can add unnecessary stress. Taking feedback from the actual users during the development phase can tremendously enhance the user experience. For example, a hospital that incorporated suggestions from nurses was able to reduce training time on its AI diagnostic tool significantly.
To highlight, consider the implications:
- Complexity: If an interface is not user-friendly, practitioners might miss vital insights that AI can provide, undermining the technology’s value.
- Customizability: Healthcare providers often work in varied settings with different patient needs. A one-size-fits-all interface is rarely optimal.
- Training Issues: Lack of comprehension can lead to ineffective use of the technology, risking patient care.
Moreover, integration of voice recognition features within the UI could simplify data entry tasks for clinicians. This could enable them to focus more on patient interactions and less on technology, although the effectiveness of voice recognition can vary across different accents and speech patterns.
Integration with Existing Systems
The integration of AI into established healthcare systems poses another layer of complexity. Many healthcare facilities operate on outdated software platforms that may not be compatible with modern AI tools. This can result in significant hurdles in data sharing, patient tracking, and overall operational efficiency.
The challenge is not simply technical; it also involves practical considerations. Legacy systems often require costly upgrades or replacements, presenting a financial hurdle that smaller institutions may find hard to overcome. Additionally, integration can lead to disruptions in regular workflows, which is daunting for medical staff who are already pressed for time.
Considerations for successful integration include:
- Interoperability: The new AI systems must communicate with existing Electronic Health Records (EHR) seamlessly. Without proper connections, essential patient data may remain siloed.
- Gradual Transition: Institutions may find success in phased implementations, beginning with pilot programs to assess effectiveness and solicit user feedback.
- Data Migration: Transferring data can be cumbersome and involves ensuring data integrity and privacy – a non-negotiable aspect in healthcare.
"The greatest danger in times of turbulence is not the turbulence; it is to act with yesterday's logic." – Peter Drucker.
Addressing technological limitations involving user interfaces and system integrations is vital for harnessing the potential of AI in healthcare. These hurdles can dampen the revolutionary capabilities of AI, making it imperative for stakeholders to plan accordingly and adapt technologies to fit into existing frameworks rather than forcing systems together without consideration.
Case Studies of AI Failures
Understanding AI failures in healthcare is not merely an academic exercise; it serves as a crucial reflection on how far we've come—and how far we still have to go. Every failure holds a critical lesson that can inform better practices for the future. The analysis of these incidents reveals specific elements of AI integration that often go overlooked in discussion. These cases shed light on the real-world implications of deploying artificial intelligence in sensitive domains like healthcare, where the stakes are incredibly high.
Notable Incidents
- IBM Watson for Oncology: When IBM introduced Watson for Oncology, many had high hopes for its ability to revolutionize cancer treatment decisions. However, it was later revealed that the AI system often relied on a limited set of cases and offered treatment recommendations that had not undergone sufficient clinical trials. This led to critical errors in recommending treatment protocols, raising eyebrows among medical professionals.
- Google's DeepMind and the NHS: In a push to streamline patient care, DeepMind partnered with the NHS to develop algorithms for diagnosing eye diseases. Unfortunately, the AI system was shown to perform inconsistently across various demographics, resulting in missed or incorrect diagnoses for certain groups. This case highlighted the troublesome prospect of algorithmic bias and its effects on health outcomes.
- Babylon Health's Symptom Checker: Babylon Health launched a symptom checker that aimed to provide preliminary medical advice. Reports surfaced where the AI provided inaccurate or incomplete analyses, causing some users to overlook serious health issues while relying too heavily on the app's output. This incident illuminated the dangers of AI misuse, particularly when people place undue trust in technology over medical professionals.
These incidents are just a few examples that highlight the importance of reviewing AI failures. Each situation denotes the necessity for robust validation mechanisms and checks before patient-facing deployment.
Lessons Learned
From these notable cases emerge vital lessons for the healthcare AI landscape:
- Thorough Validation: The stories emphasize a clear need for extensive validation of AI algorithms with diverse datasets. This mitigates the risk of bias and ensures that recommendations are sound across various demographics.
- Clinical Collaboration: Involving healthcare professionals in the development and ongoing evaluation of AI systems becomes paramount. This collaboration helps ground AI systems in real-world practices and needs.
- Transparency: Clear communication about AI's capabilities and limits is vital. Patients and providers alike need a comprehensive understanding of how AI processes information and makes decisions to avoid over-reliance on technology.
- Ethical Considerations: Every case necessitates a review of ethical implications tied to patient welfare. Being aware of potential missteps can shape the roadmap of AI deployment, aiming for a future where technology complements rather than competes with clinical expertise.
"The road to success is paved with failures that inform our next steps."
A critical approach to these incidents is imperative to develop better models, systems, and practices. Thus, examining failures isn't just about accepting mistakes; it's about harnessing knowledge to improve outcomes and ensuring that future AI implementations are both beneficial and ethically sound.
Best Practices for Implementation
The successful integration of Artificial Intelligence into healthcare hinges on a robust framework of best practices. These practices not only pave the way for effective AI usage but also ensure that the technology aligns with the core principles of patient care and safety. It's crucial to recognize that implementing AI is not a one-size-fits-all affair. Each healthcare institution must tailor its approach based on specific needs and the demographic it serves.
Stakeholder Involvement
Involving stakeholders at every stage of the AI implementation process is paramount. Stakeholders include healthcare providers, patients, technologists, and regulatory bodies. Their input helps to create a more comprehensive understanding of the needs that AI can address.
- Engagement: Regular engagement with these groups fosters an environment of collaboration. It ensures that the technology is user-friendly and meets the requirements of all involved.
- Feedback Loops: Establishing feedback loops allows for continuous improvement based on real-world experiences. For instance, doctors who frequently interact with AI tools can provide insights about usability and efficacy. This input is gold dust for refining AI algorithms and interfaces.
- Education and Training: With diverse stakeholders at play, offering tailored education about AI can minimize resistance and foster a culture of acceptance towards new technologies.
As an added note, emphasizing interdisciplinary communication is essential. For example, a doctor might have crucial insights about patient behavior that a data scientist might not have considered. Sharing knowledge can bridge gaps and yield better outcomes for AI applications.
Continuous Evaluation
Setting the stage for continuous evaluation is another linchpin in the effective use of AI in healthcare. Evaluation processes must be ongoing and adaptive to the rapidly changing landscape of both technology and patient needs.
- Performance Metrics: Establishing clear metrics is necessary to gauge the efficacy of AI systems. Hospitals and clinics should identify key performance indicators that track AI's impact on patient care, such as accuracy in diagnostics and patient satisfaction rates.
- Adapting to Feedback: No system is perfect from day one. Continuous evaluation allows healthcare providers to adapt AI systems based on aggregated patient feedback and outcomes. For example, if a particular AI tool underperforms in a specific area, knowing this early on can avert larger systemic issues.
- Regulatory Compliance: Regular evaluations are critical in ensuring adherence to healthcare regulations. As guidelines evolve, AI systems may need re-evaluation to remain compliant.
"For AI to thrive in healthcare, a cyclical process of involvement, evaluation, and enhancement must be engineered."
In summary, staking a claim in best practices ensures that AI integration in healthcare is both effective and ethical. By involving stakeholders and committing to continuous evaluation, institutions can unlock the true potential of AI technologies while safeguarding patient well-being. This approach can help to diminish the insecurities surrounding AI and pave the way for a more harmonious union between technology and healthcare.
Epilogue
In the evolving landscape of healthcare, the integration of AI technology stands as both a promising advancement and a troublesome endeavor. The multitude of challenges outlined throughout this article encapsulates the essence of why careful examination is necessary.
Summarizing Key Points
The risks and concerns associated with AI are many:
- Ethical dilemmas surrounding patient consent and decision-making autonomy.
- Data privacy issues, with compliance to regulations being a major hurdle.
- Algorithmic bias, where disparities can result in uneven patient outcomes.
- Regulatory challenges, as frameworks strive to catch up with rapid technological advancements.
- Workforce dynamics, which include job displacement and the need for developing new skills.
- Public perception issues, informing how AI technologies are embraced.
All these areas contribute to a complex narrative that intertwines technology with the delicate fabric of human health.
Future Directions
Going forward, future directions must focus on enhancing robust regulatory structures that address the nuances of AI applications in healthcare. Collaboration among interdisciplinary teams can foster innovation while adhering to ethical standards. Education and training programs for healthcare professionals should seek to bridge knowledge gaps, ensuring that the workforce is adequately equipped to work alongside AI solutions.
Furthermore, ongoing research is key in continuously evaluating the risks associated with AI. Mechanisms for stakeholder involvement must be implemented to ensure diverse voices contribute to decision-making, steering AI technologies toward equitable health outcomes.
By fostering an environment where AI can develop with vigilance, society can harness its potential while mitigating concerns that currently cloud its implementation.