Top Clinical Decision Support Systems Reviewed
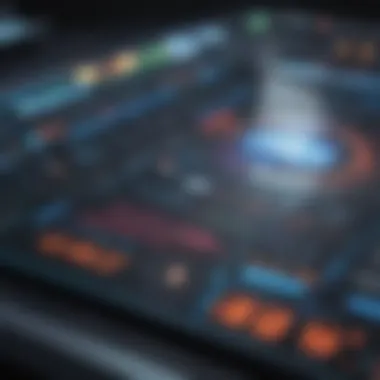
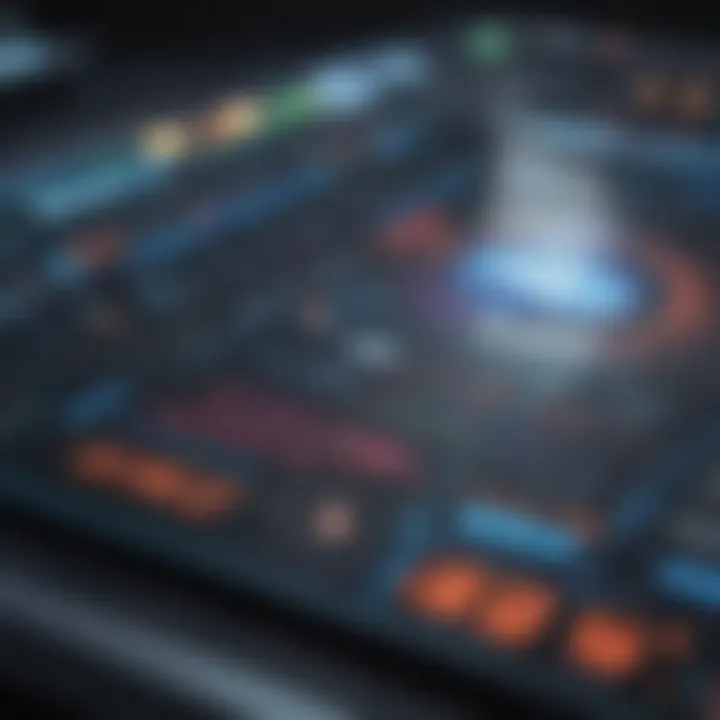
Intro
The healthcare landscape has evolved significantly in recent years, driven by advancements in technology and increasing demand for efficient patient care. Clinical decision support systems (CDSS) are powerful tools that assist healthcare providers in making informed decisions. As these systems continue to gain traction, understanding their functionalities, technologies, and applications becomes essential for students, researchers, and professionals alike.
In this article, we will examine the top clinical decision support systems currently utilized in the healthcare sector. We will analyze their capabilities, underlying technologies, and user experiences. Additionally, we will discuss the critical impact these systems have on patient care and healthcare workflows.
Research Overview
Key Findings
Research highlights various aspects of CDSS, including:
- Enhanced Patient Outcomes: Effective CDSS improve diagnostic accuracy and treatment efficacy.
- Workflow Optimization: By integrating seamlessly with electronic health records, these systems streamline clinical operations.
- User Satisfaction: Feedback from healthcare professionals indicates a positive experience when utilizing these technologies in practice.
Study Methodology
This analysis employs a multi-method approach:
- Literature Review: Comprehensive examination of existing research on CDSS technology and applications.
- Case Studies: Detailed exploration of specific systems in real-world scenarios, highlighting successes and shortcomings.
- User Surveys: Analysis of user experiences and satisfaction levels with selected CDSS technologies.
Background and Context
Historical Background
The genesis of clinical decision support systems can be traced back to the late 20th century. Initially, their focused applications involved simple rule-based algorithms to assist with diagnostic processes. Over time, technological advancements have enabled more complex systems that leverage artificial intelligence and machine learning.
Current Trends in the Field
Today, the CDSS landscape is characterized by:
- Integration with Advanced Analytics: Many systems are now paired with predictive analytics for more robust decision-making.
- Personalized Medicine: CDSS is beginning to tailor recommendations based on individual patient data.
- Interoperability: Increasing emphasis on integrative solutions that communicate across different platforms is prominent.
"Clinical decision support systems represent a shift towards data-driven decision-making in healthcare, significantly contributing to improved outcomes and operational efficiency."
Through this discussion, we aim to provide a clearer understanding of the role of clinical decision support systems in modern healthcare, preparing readers to engage with this vital and evolving field.
Prolusion to Clinical Decision Support Systems
Clinical decision support systems (CDSS) play a crucial role in modern healthcare environments. These systems are designed to assist healthcare professionals with patient data and clinical guidelines, enhancing the decision-making process. The incorporation of CDSS into healthcare can lead to significant improvements in patient outcomes, operational efficiency, and overall quality of care.
The relevance of CDSS lies not only in their technological capabilities but also in their potential to provide actionable insights. In a rapidly evolving medical landscape, these systems help in analyzing vast amounts of data, offering evidence-based recommendations tailored to individual patient needs. The utility of CDSS is particularly evident in complex clinical situations where the integration of numerous variables is necessary for effective decision-making.
It is also important to consider the challenges associated with implementing CDSS. Issues such as data security, clinician usability, and integration with existing health records systems must be addressed. Hence, understanding the fundamentals of CDSS is critical for stakeholders in the healthcare sector, encompassing their historical context, technological evolution, and future potential.
Definition of Clinical Decision Support Systems
Clinical decision support systems are defined as interactive computer programs designed to assist healthcare providers in decision-making related to patient care. These systems analyze data from various sources, including patient records and medical guidelines, to generate alerts, reminders, or recommendations. An effective CDSS can provide appropriate interventions based on specific patient characteristics and medical histories, thereby supporting personalized care.
Historical Background
The concept of decision support in medicine is not new. The origins can be traced back to the early computer systems developed in the 1960s and 1970s. Early applications of CDSS focused on simple rule-based systems that provided recommendations based on diagnostic criteria. Over decades, the sophistication of these systems increased, influenced by advances in medical knowledge, computing capabilities, and data availability. The subsequent integration with electronic health records has also accelerated their adoption within clinical practices.
Evolution of Clinical Decision Support Systems
The evolution of CDSS has witnessed several significant transitions. Initially, systems were limited to generating alerts based purely on predefined rules. However, modern iterations have become more robust, employing artificial intelligence and machine learning to enhance their functionality. Today's CDSS leverage large-scale data analytics, enabling them to predict outcomes and suggest medical interventions with a level of complexity that was previously unattainable.
Furthermore, the rise of cloud computing has facilitated the widespread implementation of CDSS in various healthcare settings. This evolution marks a shift from standalone applications to integrated systems that can communicate seamlessly across platforms, enhancing coordination among healthcare providers. The changing healthcare landscape also represents a shift toward patient-centered care, where CDSS play a vital role in ensuring that decisions are informed, relevant, and timely.
Importance of Clinical Decision Support Systems
Clinical Decision Support Systems (CDSS) are crucial in the healthcare landscape today. Their importance cannot be overstated, especially as they contribute significantly to patient care, operational efficiency, and the overall healthcare environment. The integration of these systems into clinical practice is not merely a trend; it represents a necessary evolution in how health professionals make decisions, manage patient information, and enhance health outcomes.
Enhancing Patient Outcomes
One of the primary roles of CDSS is the improvement of patient outcomes. By providing evidence-based recommendations and alerts, these systems empower clinicians to make informed decisions. For instance, if a doctor is prescribing medication, the CDSS can check for potential drug interactions or allergies specific to the patient's profile. This real-time guidance significantly reduces the risk of adverse drug events and enhances patient safety.
Moreover, the analytical capabilities of these systems ensure tailored treatment plans. Studies show that patients treated with the support of CDSS experience better health results, including faster recovery times and reduced hospital readmission rates. This shift from generalized to more personalized care models reflects the promise of CDSS in fostering improved health outcomes across diverse patient populations.
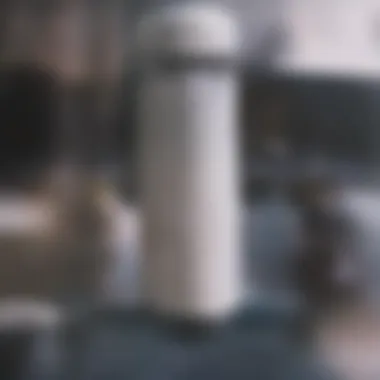
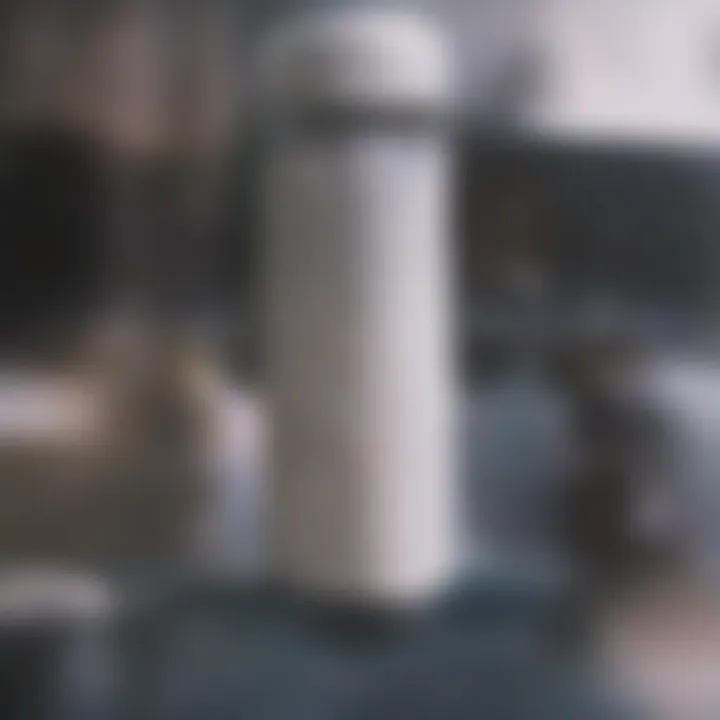
Reducing Errors in Clinical Decision Making
Clinical errors can have serious implications for patient safety, sometimes leading to life-threatening situations. CDSS can be a powerful ally in reducing these errors. By flagging potential issues such as contraindications or deviations from clinical guidelines, these systems help clinicians avoid potentially harmful mistakes.
Data from various studies indicates that systems like Epic Systems and Cerner have contributed to significant drops in medication errors among users. When healthcare providers have better access to consolidated information about patient history and clinical guidelines, they can make more accurate decisions. The proactive nature of CDSS acts as a second pair of eyes, enhancing the reliability of clinical judgments.
Supporting Workflow Efficiency
Efficient workflow is essential in clinical settings. CDSS can streamline processes that might otherwise be time-consuming and cumbersome. These systems automate routine data entry, provide quick access to patient records, and facilitate better communication among healthcare team members.
With the integration of CDSS, healthcare professionals can focus more on patient interaction rather than administrative burdens. This increased efficiency can lead to reduced wait times for patients and improved satisfaction for both patients and providers.
By enhancing workflows and reducing the administrative load, CDSS directly contributes to a more productive healthcare environment.
As healthcare systems face increasing demands, the role of CDSS in optimizing clinical workflows becomes ever more essential.
Overview of Leading Clinical Decision Support Systems
In the evolving landscape of healthcare, Clinical Decision Support Systems (CDSS) have emerged as indispensable tools. The importance of examining the leading systems lies in understanding their contribution to improved clinical outcomes, efficiency, and informed decision-making. With technology progressing rapidly, healthcare professionals need robust systems that can handle complex data and provide actionable insights.
The discussion surrounding these systems includes several critical elements. First, it is essential to consider various criteria for selecting a CDSS. Factors such as integration capabilities, user-friendliness, scalability, and support services play a significant role in determining the systems' effectiveness within healthcare settings.
Additionally, the landscape is filled with numerous options. Therefore, an evaluation of their functionalities, user experiences, and performance outcomes is crucial. This article facilitates such an evaluation, drawing attention to key features of the leading systems, highlighting advantages and possible drawbacks.
Criteria for Selection
When selecting a Clinical Decision Support System, healthcare organizations must set criteria that align with their goals and needs. Here are some key factors to consider:
- Integration: Compatibility with existing Electronic Health Records and other systems is vital for seamless operations.
- Usability: An intuitive interface helps healthcare providers navigate the system with ease.
- Evidence-Based: Systems should offer decision support based on the latest clinical guidelines and studies.
- Cost-Effectiveness: Analysis of both direct costs and potential savings through enhanced efficiency and reduced errors.
- Support Services: Ongoing customer support and training ensure that users can maximize the system's benefits.
Determining these criteria helps stakeholders make informed decisions about which system to implement.
Research Methodology
The methodology employed in assessing the leading Clinical Decision Support Systems blends both qualitative and quantitative approaches. The research involves:
- Literature Review: Analyzing peer-reviewed articles and reports to gather insights on various systems' effectiveness.
- User Surveys: Collecting feedback from healthcare practitioners who use these systems in their daily practice. This offers a real-world perspective on user satisfaction and functionality.
- Case Studies: Examining specific implementations of each system, looking at outcomes related to patient care and workflow improvements.
- Comparative Analysis: Drawing comparisons among the systems based on performance metrics and usage statistics, providing a clear picture of their strengths and weaknesses.
This comprehensive research methodology provides a well-rounded view of the leading CDSS, helping to articulate their individual contributions and overall impact on healthcare.
Epic Systems
Epic Systems is a significant player in the clinical decision support system landscape. Its noteworthy influence spans various aspects of healthcare, ranging from enhancing patient care to improving operational efficiencies. The functioning of Epic Systems not only demonstrates technological advancements but also reflects the critical needs of healthcare providers.
Overview of Epic Systems
Epic Systems was founded in 1979 and has since established itself as a leader in electronic health records and clinical decision support. The platform is widely used in hospitals and healthcare organizations across the globe. Epic offers a comprehensive solution that integrates patient data, facilitating better clinical decision-making among healthcare professionals. This integration is essential, as it streamlines access to patient health information and enables timely interventions based on data-driven insights.
Key Features and Functionality
Epic Systems boasts an array of features tailored to enhance clinical workflows. Key functionalities include:
- Clinical Documentation: Streamlines the process of recording patient information, making it easier for providers to note essential details during consultations.
- Decision Support Tools: Provides evidence-based recommendations tailored to patient needs, enhancing decision-making accuracy.
- Interoperability: Assures seamless data exchange among different systems, which is crucial for coordinated care.
- Patient Engagement: Offers tools that engage patients in their own care processes, leading to improved adherence and outcomes.
These elements collectively contribute to Epic's reputation for reliability and effectiveness in clinical environments.
User Experience and Clinical Feedback
User experience is a critical determinant in the effectiveness of any clinical decision support system. Feedback from healthcare providers utilizing Epic Systems indicates a generally positive reception. Many users highlight the intuitive interface and ease of use, which help minimize disruption to clinical workflows.
However, it is important to note that some users report challenges in customization. Tailoring the system to meet specific institutional needs can be complex. Thus, training and ongoing support become pivotal in maximizing the platform's potential. Providers often express an appreciation for continuous updates that incorporate user suggestions.
Real-World Case Studies
Real-world applications of Epic Systems illustrate its impact on healthcare delivery. For example, a major hospital group implemented Epic’s system to improve patient tracking and care coordination. As a result, they reported a 30% reduction in patient readmission rates within six months.
Another instance involved a community health center adopting Epic's decision support tools to enhance chronic disease management. The center observed improved health outcomes among diabetic patients due to more personalized care approaches.
Cerner
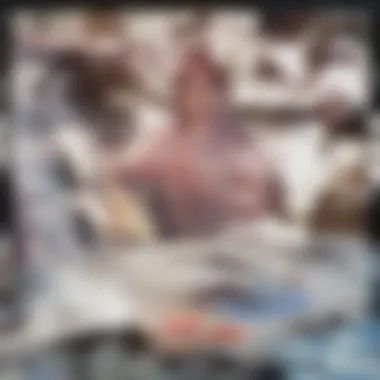
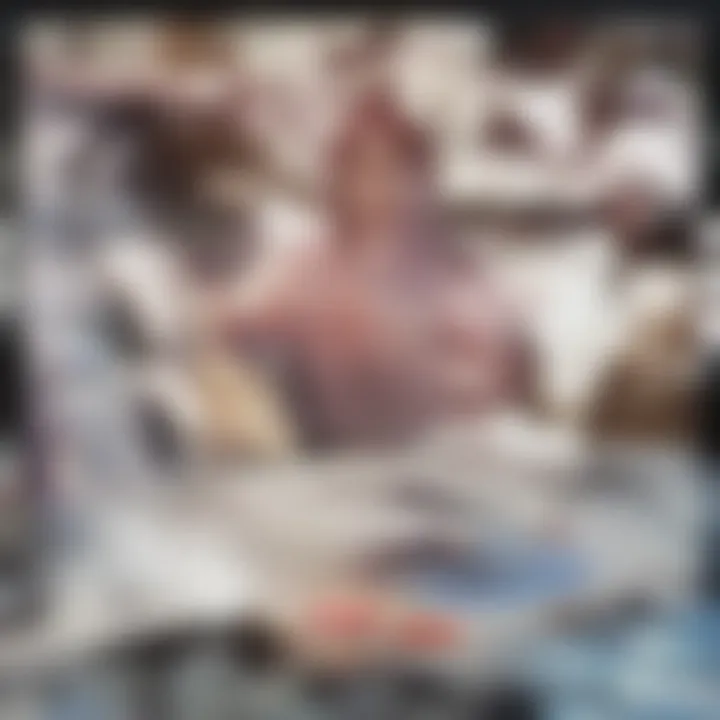
Cerner Corporation stands as a pivotal player in the realm of clinical decision support systems (CDSS). With a focus on enhancing healthcare quality, Cerner facilitates informed decision-making for healthcare professionals. By leveraging advanced technology, it provides an infrastructure that integrates seamlessly with various healthcare settings. This section delves into the specific entities that make Cerner a respected choice in CDSS, examining its innovations, user engagement, and practical implementations in real-world scenarios.
Overview of Cerner
Cerner is a global health technology company that specializes in electronic health record (EHR) solutions. Founded in 1979, it has evolved from simple information management systems to sophisticated, intelligent solutions that support clinical workflows. Cerner’s platform is designed to deliver actionable insights through data analytics and comprehensive patient information. The company emphasizes interoperability, ensuring that systems work cohesively across different healthcare facilities. This underscores Cerner's commitment to enabling healthcare professionals to make data-driven decisions that prioritize patient health.
Key Features and Technological Innovations
Cerner’s system is built on a foundation of several key features that distinctly enhance clinical workflows:
- Interoperability: Cerner's ability to integrate with various systems allows for seamless data exchange among differing platforms. This is crucial for providing a holistic view of patient data.
- Evidence-based Recommendations: The system uses algorithms to offer evidence-based suggestions that aid clinicians in choosing optimal treatment paths.
- Real-time Analytics: Data analytics tools provide instant feedback on patient conditions, enabling timely interventions.
In recent years, Cerner has invested heavily in innovative technologies such as AI and machine learning. These enhancements allow for predictive analytics and personalized medicine, making CDSS more proactive rather than reactive.
User Experience and Clinical Feedback
User experience is at the forefront of Cerner’s design philosophy. Feedback from healthcare professionals who utilize the system indicates that usability is a critical factor in the system’s effectiveness. Reviews often emphasize the intuitive interfaces and streamlined workflows.
However, like any system, it is not without its challenges. Some users express concerns over initial training time. New users may find the learning curve steep. Nevertheless, once acclimated, clinicians report higher satisfaction levels due to increased efficiency in information retrieval and reduced cognitive load during patient evaluations.
Real-World Case Studies
Practical applications of Cerner’s CDSS are illustrated through several real-world implementations. For instance, the use of Cerner's tools in the OhioHealth system has led to a measurable decrease in medication errors. Through its alert system, OhioHealth has managed to flag potential adverse drug interactions effectively.
Another case involves the Children’s Hospital of Philadelphia, where Cerner's predictive algorithms have helped identify patients at risk of sepsis earlier. This proactive approach has significantly improved patient care outcomes by facilitating timely interventions.
These examples underscore Cerner’s impact on clinical practice and provide tangible evidence of the value brought by effective clinical decision support systems.
"The integration of Cerner into our hospital's framework has transformed our clinical processes, allowing for more accurate and timely patient care decisions." - A Clinical Director
IBM Watson Health
IBM Watson Health represents a significant advancement in clinical decision support systems. Its ability to process vast amounts of healthcare data and provide actionable insights sets it apart in the field. This section delves into the facets of IBM Watson Health that contribute to its reputation and efficiency within healthcare institutions.
Overview of IBM Watson Health
IBM Watson Health is a sophisticated analytics platform designed to assist healthcare professionals in decision-making. It utilizes machine learning and artificial intelligence to analyze medical data, including clinical notes, research papers, and patient records. By ingesting and interpreting this information, Watson can help detect patterns that may not be immediately obvious to human analysts.
The system's mission is to advance health knowledge and promote a more personalized approach to medical care. By harnessing enormous datasets, it aims to empower practitioners with tools that facilitate more informed decisions for patient care.
Key Features and Advanced Analytics
IBM Watson Health is known for its comprehensive features, which include:
- Natural Language Processing (NLP): Watson uses NLP to read and interpret unstructured data from diverse sources, enabling it to understand complex medical language and provide insights.
- Predictive Analytics: Using historical data, the system predicts patient outcomes, aiding in proactive intervention strategies.
- Clinical Trial Matching: Watson can match patients to clinical trials, which can significantly enhance treatment options and speed up the research process.
These powerful analytics capabilities enhance the decision-making process, allowing healthcare professionals to deliver timely and more effective care.
User Experience and Clinical Feedback
User experience is crucial for the successful implementation of IBM Watson Health. Healthcare providers often express their need for systems that are intuitive and seamlessly integrate within their existing workflows. Feedback from clinical settings highlights several strengths of Watson, such as its ease of use and the clarity of its recommendations.
However, some users also point out challenges. Occasionally, the system may return results that require further validation from medical professionals, underscoring the need for not solely relying on tech-based solutions in clinical settings. Continuous user training is essential to maximize the benefits that IBM Watson Health can offer.
Real-World Case Studies
Real-world applications of IBM Watson Health provide essential insights into its effectiveness. For instance, a prominent cancer treatment center in the United States integrated Watson into its clinical operations. The system analyzed patient-specific information and assisted oncologists in selecting optimal treatment plans based on the latest research and clinical guidelines.
Another case study illustrates Watson's role in a hospital's emergency department. The system identified high-risk patients quickly, allowing staff to prioritize interventions. Enhancing patient flow and outcomes demonstrates Watson's practical value in critical healthcare settings.
"IBM Watson Health has transformed the way we approach patient care by providing unparalleled data insights that save time and improve outcomes."
In summary, IBM Watson Health stands out among clinical decision support systems due to its advanced analytics capabilities, strong user feedback, and proven real-world applications. Its continuous evolution holds the potential to redefine the landscape of patient care in healthcare environments.
Comparative Analysis of the Three Leading Systems
In this section, we will explore the comparative analysis of three prominent clinical decision support systems: Epic Systems, Cerner, and IBM Watson Health. This analysis is crucial as it highlights how each system aligns with the needs of healthcare providers and enhances patient care. Understanding the strengths and weaknesses of these systems can inform choices made by practitioners and decision-makers in healthcare settings. Each of these systems offers unique functionalities, technological innovations, and user experiences that directly impact clinical outcomes and workflow efficacy.
Functionality Comparison
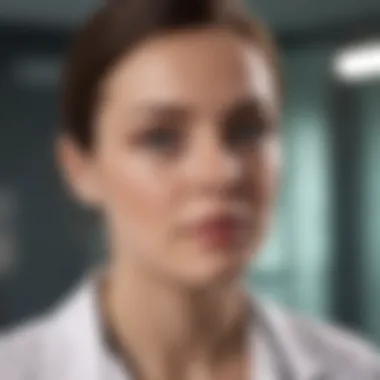
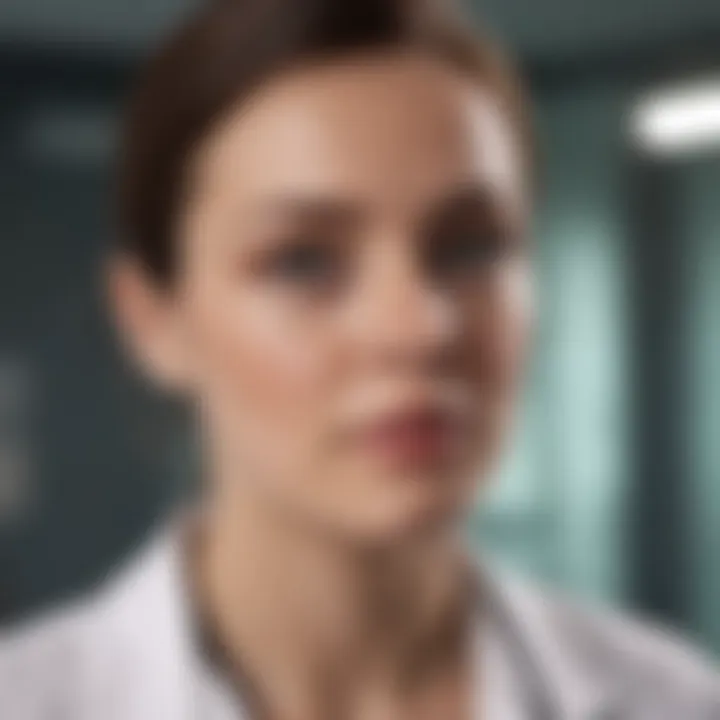
When comparing functionality among Epic Systems, Cerner, and IBM Watson Health, it is essential to consider how each one supports clinical decision-making processes. Epic Systems is known for its comprehensive electronic health record (EHR) integration, enabling seamless access to patient information across various healthcare settings. Its features include alert systems, patient engagement tools, and decision-making prompts that facilitate timely intervention.
Cerner, on the other hand, emphasizes its interoperability and cloud-based solutions. This allows for real-time data sharing among different healthcare entities, which is vital for coordinated care. With advanced analytics capabilities, Cerner supports predictive modeling that helps in identifying at-risk populations, thus aiding in preventive healthcare strategies.
IBM Watson Health stands out for its capability in processing vast amounts of unstructured data using natural language processing. This feature enables the system to generate clinical insights that may not be apparent from standard data sets. Its advanced analytics and cognitive computing make it a crucial tool for evidence-based decision-making.
- Epic Systems: Excellent EHR integration and comprehensive decision support.
- Cerner: Strong focus on interoperability with cloud solutions.
- IBM Watson Health: Unique insight generation through natural language processing.
Cost-Effectiveness
Cost-effectiveness is a pivotal factor when evaluating clinical decision support systems. Epic Systems generally requires substantial upfront investment due to its extensive functionalities and tailored solutions. However, many users report long-term savings through improved patient outcomes and operational efficiency.
Cerner allows for more flexible pricing structures because of its cloud-based model. This has made it more accessible for smaller healthcare facilities looking to implement effective decision support without excessive financial burden. The ongoing costs are typically lower compared to more traditional systems, which can be advantageous, particularly for resource-limited environments.
IBM Watson Health also brings considerable value, especially in improving diagnostic accuracy, which can directly reduce treatment costs and hospital readmissions. The innovative technology might come with a higher initial cost, but the return on investment through enhanced clinical outcomes can justify the expense over time.
User Satisfaction Surveys
User satisfaction surveys provide valuable insights into the effectiveness and usability of each system. Feedback from physicians and staff can reveal how well these systems contribute to their workflows. Generally, Epic Systems garners positive reviews regarding its usability and robust functionality, although some users mention a steep learning curve initially.
Cerner’s feedback tends to focus on its user-friendly interface and adaptability to various healthcare settings. Many users appreciate the cloud access and constant updates that Cerner offers, which contribute to an overall productive experience.
IBM Watson Health experiences mixed reviews, largely because its advanced features can be daunting for some practitioners without adequate training. However, users who invest time in learning the system often highlight its powerful insights and analytical abilities as significant advantages.
"Understanding the comparative landscape of these systems is essential for informed decision-making in healthcare settings."
In summary, this comparative analysis sheds light on how Epic Systems, Cerner, and IBM Watson Health not only differ in features and costs but also how they contribute to the overall effectiveness of healthcare delivery. By considering functionality, cost-effectiveness, and user satisfaction, stakeholders can make educated choices regarding the adoption of clinical decision support technologies.
Future Trends in Clinical Decision Support Systems
The landscape of clinical decision support systems (CDSS) is consistently evolving in response to technological advancements and changing healthcare needs. Understanding future trends in CDSS is crucial for various stakeholders, including healthcare providers, policymakers, and software developers. Such insights help shape effective strategies, enhance service delivery, and improve patient outcomes.
Integration with Artificial Intelligence
Artificial Intelligence (AI) is becoming increasingly significant in clinical decision support systems. The integration of AI can improve diagnostic accuracy and decision-making efficiency. Machine learning algorithms can analyze vast datasets to identify patterns and correlations that are often overlooked by human practitioners.
One of the primary benefits of AI integration is enhanced predictive analytics. By assessing patient data, AI can forecast potential health issues, enabling timely interventions. For instance, predictive models can notify clinicians about patients at risk of developing chronic conditions, facilitating proactive care and personalized treatment plans.
Moreover, AI can streamline the user experience. Intelligent interfaces can tailor recommendations based on individual clinician preferences and historical data usage, thereby reducing cognitive overload. This customization ensures that healthcare providers receive relevant insights without unnecessary information redundancy, thus supporting more efficient workflow.
However, the adoption of AI in CDSS is not without challenges. Concerns regarding data privacy, algorithm bias, and accountability persist. These issues require ongoing dialogue among healthcare professionals, technologists, and ethicists to ensure that AI applications are safe and equitable.
Challenges and Opportunities
The integration of advanced technologies in clinical decision support systems creates a dual landscape of challenges and opportunities.
Challenges:
- Data Quality and Accessibility:
To effectively harness AI and other technologies, it is vital to have access to high-quality patient data. Inconsistent data across various platforms can hinder the development of reliable predictive models. - User Training and Acceptance:
Healthcare professionals may face a learning curve when adapting to new technologies. Resistance to change can slow down the implementation of innovative CDSS. Institutions must invest in training and support to foster acceptance. - Regulatory Concerns:
The regulatory landscape in healthcare is complex and can impede the rapid deployment of new technologies. Ensuring compliance with regulations while innovating remains a significant hurdle for CDSS developers.
Opportunities:
- Enhanced Interoperability:
The continued development of standards for healthcare data sharing can facilitate better interoperability between CDSS and electronic health records (EHRs). This can lead to comprehensive care and improved patient management. - Personalized Medicine:
As CDSS evolves, the potential for personalized therapeutic approaches becomes more achievable. By analyzing genetic, environmental, and lifestyle factors, systems can provide tailored recommendations that optimize patient care. - Global Collaboration:
International partnerships can advance research and development in CDSS. Collaborative efforts can share resources, innovative ideas, and data that can lead to breakthroughs in clinical decision support.
In summary, Future trends in clinical decision support systems are defined by the integration of AI, potential challenges to implementation, and numerous opportunities for healthcare innovation. Stakeholders must navigate this complex terrain with foresight and diligence, ensuring that the primary goal remains the delivery of optimal patient care.
The End
The conclusion of this article synthesizes the insights gained from examining the leading clinical decision support systems (CDSS). These systems play a crucial role in the healthcare landscape, impacting patient outcomes and the efficiency of clinical workflows.
Summary of Findings
Through extensive analysis, several key points emerge.
- Enhanced Patient Care: The integration of CDSS leads to improved diagnostic accuracy and treatment recommendations. Healthcare providers are equipped with tools that assist in making informed decisions, ultimately benefiting patient safety and care quality.
- Error Reduction: Clinical decision support systems assist in minimizing errors, which can have serious consequences in healthcare settings. The systems provide alerts, reminders, and evidence-based guidelines that help clinicians avoid potential pitfalls in their decision-making process.
- Workflow Efficiency: By streamlining processes, CDSS allow healthcare professionals to focus on patient care rather than administrative burdens. This efficiency is crucial in fast-paced environments where time is often limited. The user-friendly designs and functionalities of leading systems such as Epic Systems, Cerner and IBM Watson Health facilitate smooth integration into existing workflows.
In essence, the findings emphasize the pivotal role of CDSS in modern healthcare, underscoring their benefits in enhancing the overall quality of care and operational workflows.
Final Thoughts on the Role of CDSS
The ongoing evolution of clinical decision support systems indicates their enduring significance in healthcare. As technology advances, we can expect CDSS to become even more sophisticated, leveraging artificial intelligence to provide even deeper insights.
The transformative power of CDSS lies not just in their technology, but in their ability to support clinical judgment, enhance patient safety, and ultimately improve healthcare delivery.
It is essential for stakeholders, including healthcare providers, administrators, and policymakers, to recognize the importance of investing in and optimizing these tools. Doing so will not only advance healthcare but also foster a culture of continuous improvement and learning.