Exploring Qiacuity and DPCR in Scientific Research
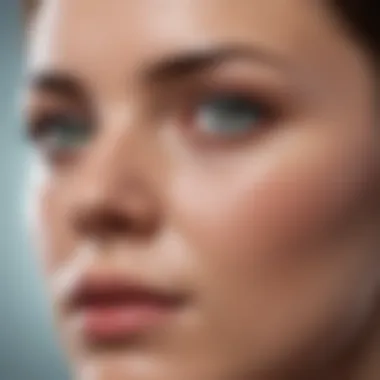
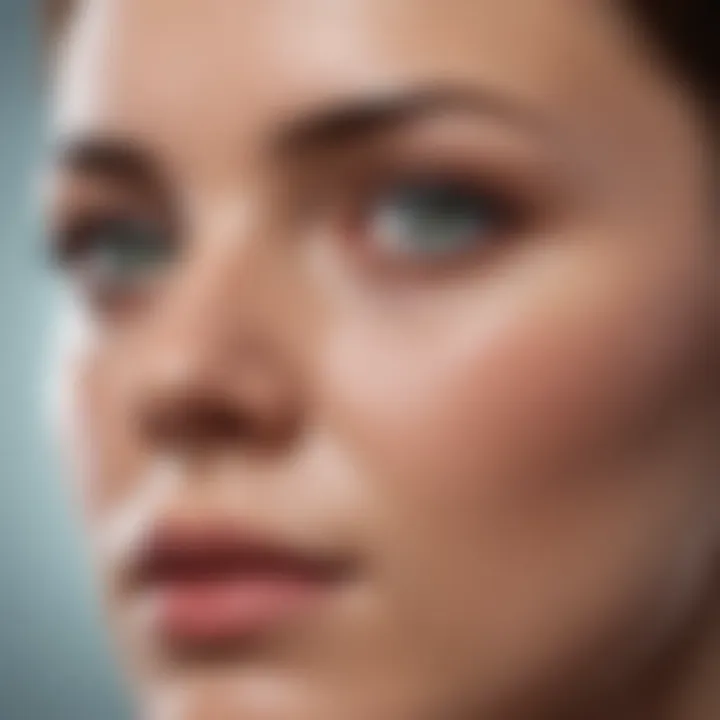
Intro
The synthesis of innovative ideas often propels scientific inquiry forward. In current research, two concepts are becoming increasingly relevant: qiacuity and DPCR. Qiacuity embodies the intricacies of cognitive processing within the realm of scientific investigation. It reflects how researchers synthesize information and apply critical thinking to their methods. DPCR, on the other hand, stands for Data-Driven Predictive Control Research. This concept emphasizes decision-making based on data analysis and empirical evidence.
Together, these concepts bridge the gap between theoretical frameworks and practical applications, encouraging researchers to enhance their methodologies. Exploring these ideas provides crucial insights that aim to elevate the quality of scientific research across various disciplines. This article will delve into their historical context, theoretical implications, and practical applications, setting a comprehensive stage for a deeper understanding of these concepts.
Research Overview
Key Findings
The exploration of qiacuity and DPCR reveals critical intersections in scientific methodology. Key findings from recent investigations include:
- Cognitive sophistication plays a vital role in how effectively researchers approach complex problems.
- Data-driven methodologies offer reliable pathways for conclusive decision-making, reducing biases associated with traditional approaches.
- The integration of qiacuity and DPCR fosters enhanced collaboration among researchers, leading to innovative solutions.
Study Methodology
To investigate these concepts, a mixed-methods approach was utilized. This included quantitative surveys distributed among researchers and qualitative interviews aimed at understanding their experiences. Data analysis highlighted patterns in cognitive strategies employed by researchers and their reliance on data-driven decision-making. This dual methodology allowed for a comprehensive view that points to the strengths and weaknesses of current practices.
Background and Context
Historical Background
The term qiacuity originates from ancient philosophical discussions on intellect and wisdom, evolving into its current scientific interpretation. Historically, cognitive processing has been linked to various paradigms in psychology and neuroscience, influencing how researchers approach problems today. Meanwhile, DPCR has roots in engineering and computer science, where data has long been considered essential in improving predictive models. The intersection of these fields marks a new era in research methodology, blending traditional cognitive theories with modern data analytics.
Current Trends in the Field
Currently, there is a strong emphasis on interdisciplinary collaboration. Researchers from varied backgrounds are coming together to harness the potential of qiacuity and DPCR. Current trends include:
- The rise of big data analytics, which provides extensive datasets for researchers.
- Increased focus on machine learning and its application in research design and execution.
- The shift towards open science practices, enhancing transparency and reproducibility in research methodologies.
Preface to Qiacuity and DPCR
The concepts of qiacuity and DPCR are becoming increasingly relevant in scientific inquiry. Understanding these notions can play a crucial role in shaping future research methodologies. Qiacuity signifies the sophistication of cognitive processing applied to scientific questions, whereas DPCR focuses on the significance of data-driven decision-making in research processes.
Researching these concepts is essential for modern scientists. In an age where data abounds, the ability to process it effectively is a requisite for successful outcomes. Familiarity with qiacuity facilitates enhanced cognition, helping researchers navigate complex information landscapes. Simultaneously, knowledge of DPCR empowers researchers to make informed decisions based on data rather than intuition alone.
To benefit maximally from these concepts, one requires not only an understanding of their definitions but also practical implications in various scientific fields. This article aims to dissect these elements and explore their intersections, hoping to improve investigative efforts across disciplines and contribute positively to the evolving landscape of scientific research.
As research evolves, the integration of qiacuity and DPCR becomes a matter of necessity rather than choice.
Understanding these concepts not only enriches one’s knowledge base but also sets the groundwork for more rigorous experimentation and analysis. The next sections will offer definitions, overviews, and highlight the importance of these ideas, creating a comprehensive picture for readers seeking to delve deeper into this essential area.
Historical Background
Understanding the historical background of qiacuity and data-driven decision making (DPCR) is crucial in grasping their current applications and significance in scientific research. The development of these concepts reflects shifting paradigms in how knowledge is acquired and utilized within various disciplines. By tracing the evolution of qiacuity and the emergence of DPCR, one can appreciate their roles in shaping modern research practices while acknowledging the lessons learned from past approaches.
Evolution of Qiacuity in Scientific Thought
The term qiacuity is relatively novel, but its roots can be traced back to longstanding discussions in cognitive science and philosophy. Historically, cognitive processing was largely viewed through the lens of rationality and logic. Scholars like René Descartes emphasized the role of clear and distinct ideas in understanding the world. Over time, as empirical methods gained traction, the focus shifted toward how individuals process, interpret, and integrate information in their scientific inquiries.
In the mid-20th century, researchers began to articulate more nuanced understandings of cognitive processes. The work of scholars like Jean Piaget and Lev Vygotsky laid the groundwork for recognizing the importance of context and social interaction in learning and cognitive development. This evolving perspective allowed for a broader definition of qiacuity, encompassing not only the capability to reason logically but also the ability to synthesize knowledge from various sources and apply it appropriately in novel situations.
"Qiacuity is not merely about individual intellect; it includes the awareness of one's cognitive biases and the capacity to adjust reasoning processes in light of new evidence."
By the late 20th and early 21st centuries, the interaction between cognitive processing and scientific inquiry became increasingly evident. Researchers in cognitive psychology, neuroscience, and educational theory emphasized the role of metacognition and reflective thinking as critical components of qiacuity. This evolution illustrates a shift toward appreciating the complexities of human cognition, emphasizing adaptability within scientific practices.
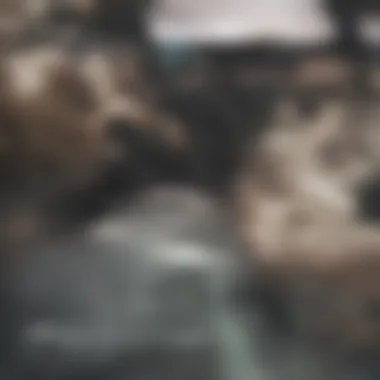
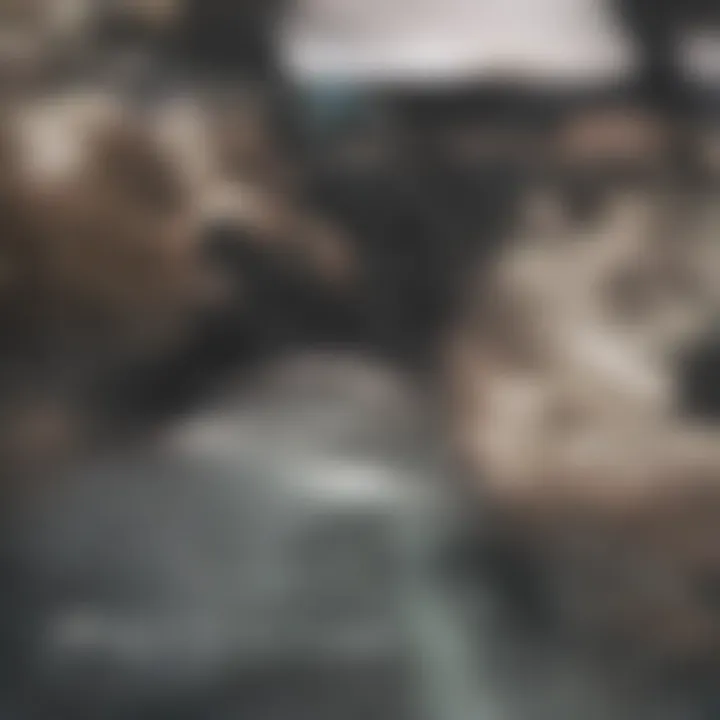
The Development of DPCR Over Time
DPCR arose from the confluence of various fields, including statistics, computer science, and data analytics. Its evolution reflects a growing recognition of data as a vital resource for making informed decisions in research. The concept gained momentum with the advent of advanced computational tools in the late 20th century. These tools enabled researchers to collect, analyze, and interpret increasingly large datasets.
In the early stages, data-driven approaches primarily focused on descriptive analytics. Researchers used data to describe observed phenomena, often without drawing substantial conclusions. As methods of statistical inference evolved, attention shifted toward predictive modeling and inferential statistics.
The late 1990s and early 2000s marked a significant turning point. The rapid proliferation of the internet and advancements in machine learning led to a transformation in how research questions are framed and addressed. Multivariate analysis and data mining gained prominence, allowing for more sophisticated applications of DPCR across disciplines.
Today, DPCR is recognized as a cornerstone of evidence-based practices in various fields, from healthcare to social sciences and environmental studies. The acknowledgment of data integrity and the ethical considerations surrounding data use are critical areas of focus. As researchers grapple with both the potential and limitations of data-driven decision-making, the understanding of DPCR continues to evolve, adapting to new challenges and opportunities in research.
In summary, both qiacuity and DPCR have seen significant development over time. The historical context of these concepts provides a foundation for understanding their current relevance and application in scientific research.
Theoretical Framework
The theoretical framework serves as a critical foundation within the realm of scientific research, especially when examining the confluence of qiacuity and DPCR. It provides a structured lens through which researchers can understand the intricate dynamics at play in cognitive processing and decision-making. This framework also addresses the assumptions and principles underlying these concepts, guiding future research directions and methodologies. An effective theoretical framework can illuminate the connections between qiacuity and DPCR, allowing the investigation to be more robust and sophisticated.
Cognitive Processing in Qiacuity
Cognitive processing is the cornerstone of qiacuity. It denotes how knowledge is acquired, used, and transformed within the mind. Qiacuity reflects the sophistication and efficacy of this cognitive processing in scientific inquiry. In this context, researchers who exhibit heightened qiacuity demonstrate the ability to synthesize complex information and derive meaningful insights.
The implications of cognitive processing for qiacuity are multi-faceted:
- Enhanced problem-solving: High levels of qiacuity empower scientists to tackle intricate research questions. They can navigate uncertainties and complexities, finding innovative solutions that may not be apparent to others.
- Critical thinking: Researchers with strong cognitive processing skills engage in critical analysis of data and theories. This skill is vital in validating research findings and ensuring that conclusions drawn are reliable.
- Adaptability: An adaptable cognitive approach allows researchers to pivot their focus as new information emerges. Qiacuity thus facilitates a responsive research environment.
In academic and professional settings, cultivating cognitive processing is essential for advancing one's qiacuity and, by extension, enhancing overall research outcomes.
Data-Driven Decision Making in DPCR
DPCR stands for Data-Driven Predictive Conceptualization and Research. The essence of DPCR lies in its reliance on empirical data to inform decision-making processes in scientific study. As research methodologies continue to evolve, the emphasis on data-driven approaches cannot be overstated. In this context, DPCR enhances the research process in several important ways:
- Evidence-based conclusions: Utilizing data enables researchers to draw conclusions backed by measurable evidence rather than assumptions or anecdotal experiences. This practice elevates the credibility of scientific research.
- Efficiency: Implementing a data-driven strategy streamlines research processes. It allows for quicker iterations and testing of hypotheses, maximizing the use of resources and time.
- Predictive analytics: Advanced analytical tools enable scientists to predict outcomes based on historical data. This predictive capability enhances strategic planning and risk management within research projects.
- Interdisciplinary collaboration: The commonality of data usage fosters collaborative opportunities across various scientific disciplines. This collaboration is crucial in addressing multidimensional scientific challenges.
Ultimately, leveraging DPCR not only refines research methodologies but also establishes a framework where data guides scientific discovery.
Methodological Implications
The topic of methodological implications is an essential focus in the exploration of qiacuity and DPCR within scientific research. As the sophistication of cognitive processing and the efficiency of data-driven decision-making emerge as critical components in modern inquiry, recognizing their impact on research design becomes paramount. This section will delve into the intricate relationship between these concepts and their methodological applications, enhancing the quality of research outcomes.
Research Design Incorporating Qiacuity
Research design that incorporates qiacuity reflects a sophisticated understanding of cognitive biases and processing capabilities. When researchers account for qiacuity, they recognize the variations in cognitive functions across individuals and how these differences affect interpretations and conclusions.
- Elements of Qiacuity in Research Design:
- Cognitive Diversity: Researchers must consider the diversity of cognitive processing styles among participants. This awareness creates a more inclusive environment that can yield richer, more varied insights.
- Bias Mitigation Measures: By integrating knowledge of qiacuity, researchers can apply specific strategies to minimize cognitive biases, ensuring that findings are reflective of true phenomena rather than individual misinterpretations.
The integration of qiacuity directly influences the formulation of hypotheses, data collection methods, and subsequent analysis, reinforcing the foundation of scientific rigor.
Leveraging DPCR in Experimental Studies
Data-driven decision-making, or DPCR, plays a vital role in experimental studies, providing a framework to utilize data effectively throughout the research process.
- Benefits of DPCR:
- Objective Evaluation: Leveraging DPCR promotes a more objective evaluation of data by emphasizing empirical evidence over subjective interpretation. This is crucial in enhancing the reliability and validity of research findings.
- Feedback Mechanisms: DPCR cultivates adaptive feedback mechanisms within experimental designs. This allows researchers to adjust methodologies in response to preliminary results, improving overall outcomes through iterative refinement.
Incorporating DPCR into experimental studies fosters an environment that values empirical evidence and enhances the capability of researchers to draw reliable conclusions. This approach underscores the necessity of grounding scientific inquiry in robust, data-informed frameworks that align with contemporary research needs.
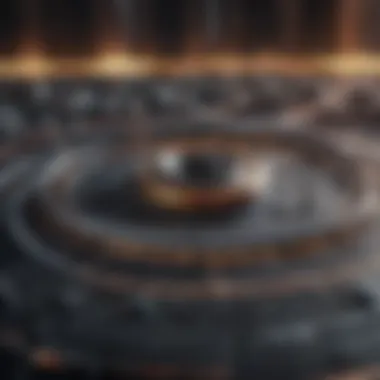
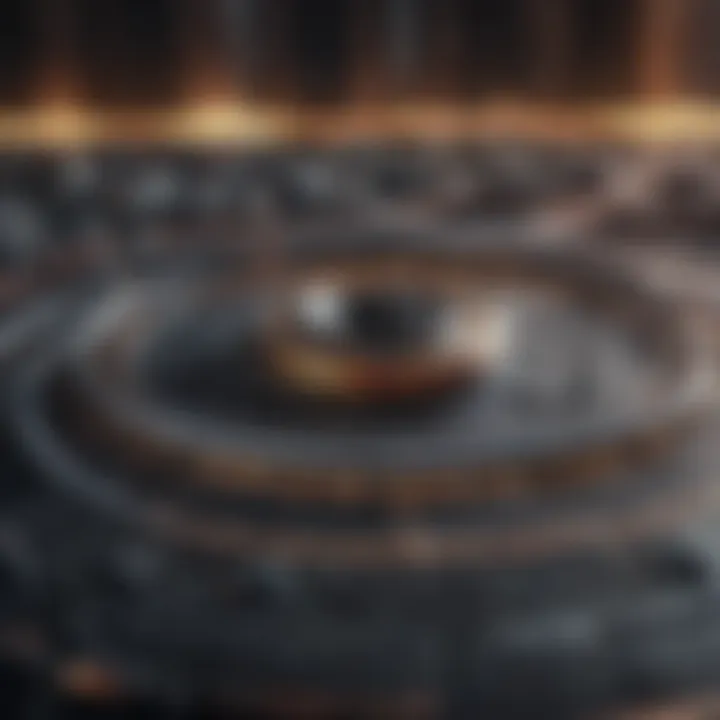
Applications in Various Scientific Fields
The concepts of qiacuity and DPCR possess substantial significance across diverse scientific fields. Their integration fosters enhanced research methods, leading to improved outcomes in various inquiries. Qiacuity influences cognitive processing, shaping how scientists approach complex problems. In contrast, DPCR emphasizes the reliance on data, guiding decisions derived from empirical evidence. As scientific disciplines continue to evolve, understanding the roles of these concepts remains critical.
Qiacuity in Biology
In biology, qiacuity is particularly relevant. It encompasses the mental frameworks scientists use to understand intricate biological processes. Researchers often deal with vast amounts of data, from genetic sequences to ecological patterns. A strong qiacuity allows for better hypothesis formation and experimental design.
- Applications:
- Investigating gene regulatory networks.
- Modeling population dynamics within ecosystems.
By applying qiacuity, biologists can identify connections and potential causations within the data, leading to innovative research breakthroughs.
DPCR in Chemistry
In the realm of chemistry, DPCR has transformed the way chemists conduct experiments and interpret results. By utilizing data-driven methodologies, chemists can make informed predictions about reactions and product yields. This approach minimizes errors and enhances the reliability of findings.
- Key Aspects:
- Utilization of computational chemistry simulations.
- Implementation of machine learning for reaction predictions.
DPCR fosters a culture of evidence-based decision-making, ensuring that theoretical assumptions are tested rigorously using real-world data.
Integrating Qiacuity and DPCR in Physics
Physics benefits uniquely from the synergy between qiacuity and DPCR. Theoretical constructs often necessitate a robust understanding of complex systems, which relies on qiacuity. Simultaneously, DPCR equips physicists with the analytical tools necessary to support or refute these theories.
- Importance in Research:
- Enhancing the development of new theories in classical and quantum mechanics.
- Streamlining experimental designs in particle physics.
Integrating both concepts enables physicists to push the boundaries of knowledge, advancing our understanding of fundamental principles.
Earth Sciences: The Role of Qiacuity and DPCR
In earth sciences, combining qiacuity and DPCR provides a comprehensive framework for tackling environmental challenges. Researchers study complex systems, such as climate patterns and geological formations. Here, qiacuity assists in conceptualizing intricate relationships, while DPCR facilitates data analysis and environmental modeling.
- Significance:
- Analyzing climate change impacts.
- Predicting geological hazards using data trends.
Both qiacuity and DPCR serve as indispensable tools, guiding scientists toward making informed decisions based on empirical evidence creating solutions that address urgent global issues.
Challenges and Limitations
Understanding challenges and limitations is essential in the exploration of qiacuity and DPCR. Challenges within scientific frameworks not only shape research outcomes but also demand that researchers critically assess factors impacting their findings. This section addresses critical elements such as cognitive biases in relation to qiacuity and issues regarding data integrity in DPCR. Each of these elements has implications for the accuracy and reliability of scientific work, thus warranting attention.
Cognitive Biases Affecting Qiacuity
Cognitive biases are systematic patterns of deviation from norm or rationality in judgment. In the context of qiacuity, these biases can significantly influence how scientists interpret data and formulate hypotheses. Whether it is confirmation bias, where researchers favor information that supports their preconceptions, or anchoring bias, where initial information unduly influences subsequent assessments, these effects can alter the trajectory of scientific inquiry.
Some common cognitive biases affecting qiacuity include:
- Confirmation Bias: Researchers might only seek out data that corroborates their existing beliefs.
- Hindsight Bias: This leads to overestimating the predictability of outcomes after they happen, skewing future research focus.
- Overconfidence Effect: Researchers may overestimate their understanding of phenomena, leading to misinterpretation of results.
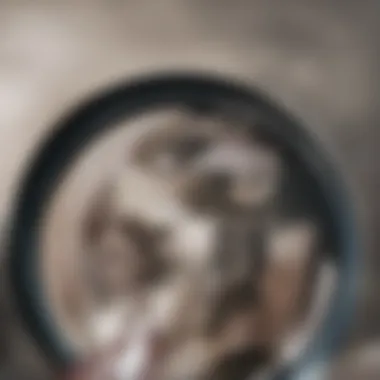
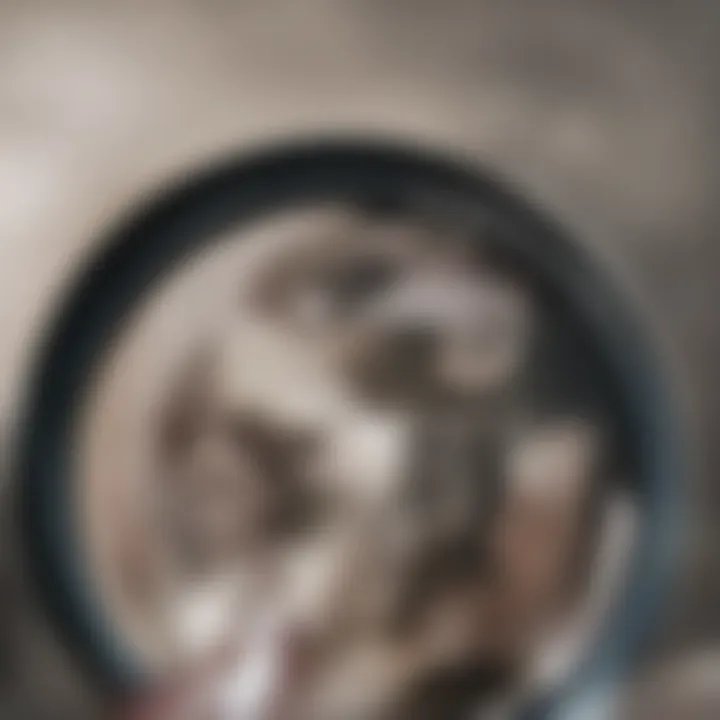
Addressing these biases requires conscious effort. Researchers must engage in reflective practices and incorporate peer review to mitigate bad judgments. This also involves promoting an environment where diverse perspectives are valued, allowing for more balanced interpretation of complex data.
Data Integrity Issues in DPCR
Data integrity is a cornerstone of robust DPCR methodologies. The accuracy, consistency, and reliability of data directly affect decision-making processes in research. Yet, several threats challenge data integrity, including human error, inadequate data management systems, and intentional misconduct.
Possible issues impacting data integrity involve:
- Data Entry Errors: Simple mistakes during data input can lead to significant discrepancies.
- Lack of Standardization: Without standardized procedures for data collection, variability can emerge, complicating analyses.
- Data Fabrication/Falsification: Intentionally altering data misleads subsequent conclusions and can have severe ethical implications.
To safeguard data integrity, researchers must employ rigorous validation techniques and employ technology that enhances accuracy. Establishing protocols for data sharing and transparency is also crucial to uphold trust within the research community.
"Data without integrity is like a building without a foundation; it cannot stand firm."
In summation, recognizing these challenges and limitations not only informs researchers but primes them to engage in more robust scientific exploration. As we reflect on qiacuity and DPCR, vigilant awareness of these factors will ultimately enrich the quality of contemporary scientific research.
Future Directions of Research
The exploration of qiacuity and DPCR continues to evolve, presenting numerous opportunities for advancement in the scientific field. Understanding future research directions in these areas is essential for optimizing methodologies and improving outcomes. Key elements to consider include the integration of cognitive strategies and harnessing the latest in data analytics technology. These considerations emphasize both theoretical and practical implications, encouraging researchers to adopt innovative techniques while addressing existing limitations.
Enhancing Qiacuity in Research Practices
Improving qiacuity in research practices involves refining cognitive processing capabilities among researchers. Here are some strategies that can enhance this aspect:
- Training Programs: Implementing targeted training sessions focusing on critical thinking and analytical skills can substantially improve researchers' qiacuity.
- Collaborative Efforts: Encouraging collaboration between disciplines can foster diverse thinking and enhance cognitive flexibility.
- Feedback Mechanisms: Establishing transparent feedback channels in research teams can help identify cognitive biases and improve decision-making processes over time.
These methods not only elevate the quality of research but also contribute to more effective problem-solving approaches in varied scientific contexts.
Innovative Approaches to DPCR
The domain of DPCR is rapidly changing, with several innovative approaches emerging. These include:
- Machine Learning: Utilizing machine learning algorithms can significantly improve data analysis processes, allowing for more accurate predictions and informed decision-making.
- Visual Analytics: Employing interactive visual analytics tools aids researchers in comprehending complex datasets, facilitating better insights.
- Continuous Learning Systems: Implementing systems that update and learn from new data continuously can enhance the reliability of the decision-making process in research.
Incorporating these approaches not only advances DPCR but also ensures that research remains relevant and impactful in a fast-paced scientific landscape.
"The synergy between qiacuity and DPCR can transform research practices, enabling more robust scientific inquiries and fostering innovation within the research community."
The future of scientific research depends on integrating both concepts effectively, leading to a more cohesive understanding and application in various fields.
Ending
In this article, we explored the critical themes surrounding Qiacuity and DPCR, emphasizing their significance in scientific research. Understanding these concepts has numerous benefits for researchers engaged in various fields. For one, Qiacuity aids in refining cognitive processing, essential for formulating hypotheses and interpreting data effectively. Enhanced cognitive skills mean better analytical capabilities, allowing researchers to draw more nuanced conclusions from their studies.
On the other hand, DPCR is pivotal for the modern research environment. In an era where data is abundant, making informed decisions based on solid data interpretation is crucial. Researchers can leverage DPCR to optimize their methodologies, ensuring that the insights drawn from studies are both reliable and actionable. Integrating both Qiacuity and DPCR results in a more robust research framework, enhancing the overall quality of scientific inquiry.
The discourse on these topics highlights several critical considerations, such as the need for researchers to develop both cognitive and technical skills. Fostering an environment where these two concepts are valued can significantly influence the progress of scientific research. By understanding and implementing Qiacuity and DPCR, researchers can effectively navigate the complexities of modern inquiries, leading to substantial advancements in their respective fields.
Summarizing the Key Insights
The exploration of Qiacuity and DPCR reveals important insights into their roles in scientific research. Key points include:
- Qiacuity refers to the sophistication of cognitive processing crucial for evaluating scientific phenomena.
- DPCR underscores the importance of making decisions based on data analysis rather than intuition alone.
- The integration of both allows for more comprehensive research methodologies.
- Researchers enhancing their Qiacuity can improve their ability to analyze and understand complex data.
- Adopting DPCR aids in establishing a reliable research environment, facilitating reproducibility.
In summary, the intersection of Qiacuity and DPCR creates a framework that supports ongoing scientific innovation and improves the quality of research outcomes.
The Interconnectedness of Qiacuity and DPCR
The relationship between Qiacuity and DPCR is not merely complementary but rather intertwined. Both concepts rely on each other to maximize the effectiveness of research practices. Qiacuity fosters the critical thinking necessary for researchers to ask the right questions, which is essential for effective data gathering and analysis. On the flip side, DPCR enhances the ability to process information effectively, translating insights from data into informed decisions.
- The synergy between the two encourages researchers to continuously question their assumptions, leading to a more dynamic scientific process.
- Adopting a Qiacuity approach can improve the interpretation of data produced through DPCR methodologies.
- This interconnectedness also emphasizes the importance of training in both areas to cultivate well-rounded researchers equipped to tackle today’s scientific challenges.
By cultivating both Qiacuity and DPCR, researchers pave the way for innovative solutions and more significant contributions to science.