Machine Learning Advancements in Hearing Aids
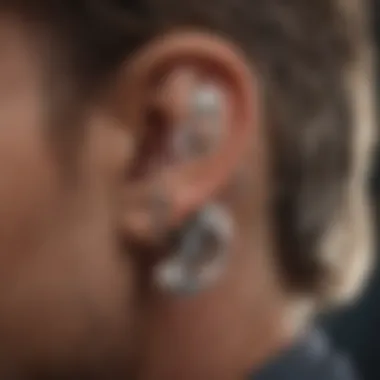
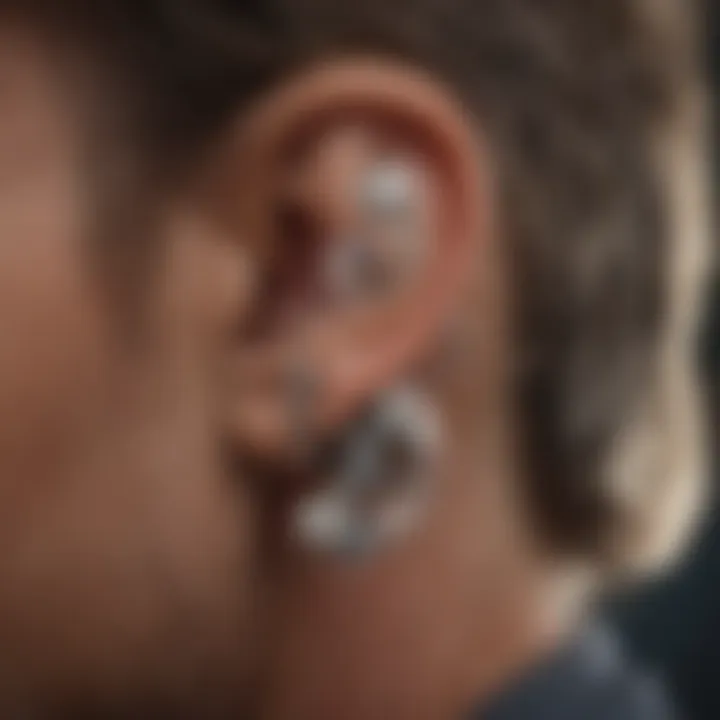
Intro
The integration of machine learning in hearing aids is a pivotal advancement that is reshaping the landscape of auditory care. This technology enhances traditional hearing devices, making them more adaptive and personalized to individual user needs. With continuous research and development, manufacturers are eager to leverage machine learning to create smarter solutions for hearing health. Users can benefit through improved sound quality, tailored settings, and greater ease of use.
Research Overview
Key Findings
Recent studies illustrate the significant efficacy of machine learning in optimizing hearing aid performance. Devices equipped with this technology can analyze diverse sound environments and adjust settings automatically. This ability to learn from user behavior results in better hearing experiences and minimizes manual adjustments. Additionally, research highlights a notable improvement in speech recognition ability, particularly in noisy environments, thanks to advanced signal processing algorithms.
Study Methodology
A variety of research methodologies have contributed to these findings. Evaluations typically involve controlled experiments, where users are monitored while interacting with various hearing aids. Data collection includes user feedback, performance metrics, and sound analysis in differing acoustic environments. Studies also utilize machine learning models to predict user preferences and sound characteristics, providing a more accurate understanding of user needs.
Background and Context
Historical Background
The evolution of hearing aids traces back to early mechanical devices designed to amplify sound. Over time, technology has shifted towards digital innovations. The late 20th century saw the advent of digital signal processing, vastly enhancing clarity and customization. The introduction of machine learning is a natural progression, allowing devices to adapt in real-time to the complexities of sound.
Current Trends in the Field
Today, there is a growing emphasis on personalized hearing solutions. Users' profiles can be dynamically updated based on their environments and preferences, leading to a more user-friendly experience. Furthermore, research is pushing boundaries around remote tuning capabilities, where audiologists can adjust settings based on user data collected remotely.
"Machine learning is not just a trend; it is a transformational force in auditory science that is enabling users to regain their auditory independence."
This trend signifies a shift not only in product development but also in how audiologists approach patient care. The combination of artificial intelligence and audiology represents an exciting frontier with ample potential for future enhancements.
Prolusion to Hearing Aids
Hearing aids have become critical tools in improving the quality of life for individuals with hearing loss. They amplify sound and enhance the listening experience, enabling users to engage meaningfully in conversations and social settings. Understanding hearing aids is essential as they are not merely devices; they represent a significant advancement in medical technology, addressing complex auditory challenges.
The evolution of hearing aids illustrates a remarkable journey fueled by innovation. Initially, these devices were purely analog, only amplifying sound without any sophistication. However, the integration of digital technologies marked a transformative phase. Today, hearing aids can adapt to various environments, learning user preferences and adjusting accordingly. This flexibility is increasingly crucial in our noise-polluted world.
Moreover, the advent of machine learning is set to revolutionize hearing aids even further. This technology enables devices to process sounds more intelligently, distinguishing between desired sounds and background noise. By analyzing user behavior, the systems can personalize settings for different situations, enhancing both effectiveness and user satisfaction. The intersection of machine learning and hearing aids signifies a profound shift towards creating more responsive and user-friendly solutions.
To appreciate how machine learning can enhance these devices, it is vital to look back at the historical context of hearing aids and understand the current technologies that are shaping their development today.
Understanding Machine Learning
Understanding the principles of machine learning is essential for grasping its application in hearing aids. This section will assess key elements, benefits, and considerations related to machine learning. It is vital to recognize that machine learning empowers hearing aids to analyze sound environments and adapt to user preferences. This adaptability is not merely a feature; it represents a significant leap in hearing aid technology.
Defining Machine Learning
Machine learning refers to the capability of algorithms to learn patterns from data rather than following explicit programming. It enables systems to improve their performance over time. In the context of hearing aids, these algorithms analyze sound inputs, categorize them, and respond in ways that enhance hearing experiences. This functionality is crucial because users often encounter diverse auditory environments.
Types of Machine Learning
Machine learning consists of various types; each has its role in the optimization of hearing aids. The core types are supervised learning, unsupervised learning, and reinforcement learning.
Supervised Learning
Supervised learning involves training a model on a labeled dataset. In this method, the model learns to make predictions based on input-output pairs. In hearing aids, this can help in identifying different sound types, such as speech or background noise.
- Key Characteristic: The reliance on labeled data.
- Contributions: It helps create precise models that can distinguish between sound types effectively.
- Advantages: It provides consistent predictions since the model learns from examples where the correct output is known.
- Disadvantages: It necessitates a large amount of labeled data for training, which can be difficult to obtain in some environments.
Unsupervised Learning
Unsupervised learning differs in that it works with unlabeled data. The goal is to uncover hidden patterns without predefined outputs. In hearing aids, this can play a role in categorizing various sounds in the environment, which can then be used to enhance sound processing.
- Key Characteristic: The absence of labeled outputs.
- Contributions: It delivers insights into the sound environment by autonomously detecting correlations between different sound inputs.
- Advantages: It reduces the burden of needing labeled data, allowing for flexibility in handling diverse sound scenarios.
- Disadvantages: The lack of supervision can lead to less precise predictions, as the trained model may misinterpret sounds if patterns are not clear.
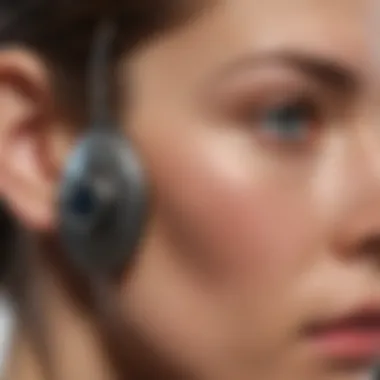
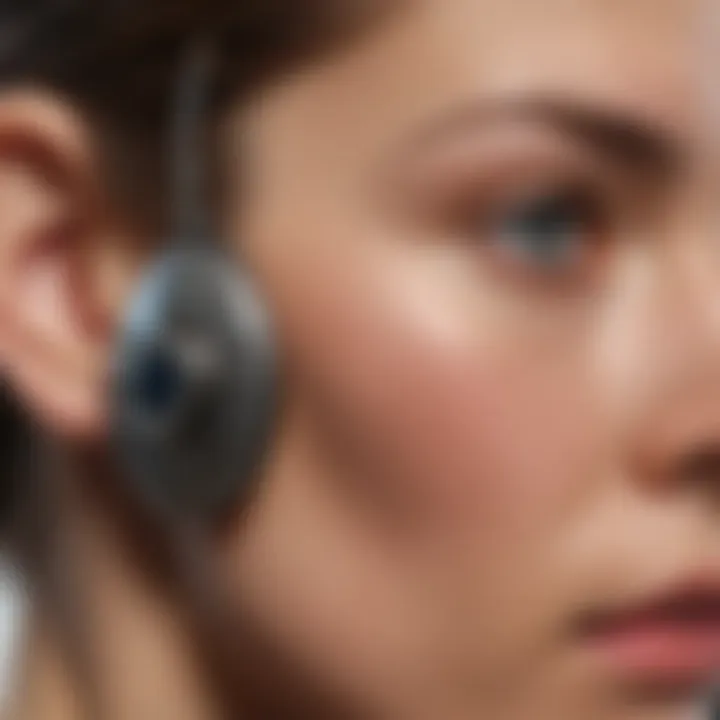
Reinforcement Learning
Reinforcement learning relies on a system of rewards and penalties to guide the learning process. This approach can be particularly useful in dynamic environments where hearing aids must continually adapt to varying sound conditions.
- Key Characteristic: Learning through interaction with the environment.
- Contributions: This allows hearing aids to continuously improve by learning the optimal adjustments for various settings.
- Advantages: It is effective in scenarios requiring real-time decision-making and continuous learning.
- Disadvantages: It requires a significant amount of interaction data, which can be computationally intensive and complex to manage.
In summary, understanding machine learning highlights its multifaceted applications within hearing aids. Each type offers unique benefits and consideration, facilitating a comprehensive approach to enhancing auditory experiences.
Integration of Machine Learning in Hearing Aids
The integration of machine learning in hearing aids represents a significant evolutionary step in the enhancement of auditory devices. Traditional hearing aids primarily amplified sound, which did not always meet the dynamic needs of users. Machine learning, however, introduces an adaptive capability that allows hearing aids to process and interpret sound with greater sophistication. This new approach not only improves the user's listening experience but also fundamentally changes how these devices interact with their environment.
Adaptive Sound Processing
Adaptive sound processing enables hearing aids to dynamically adjust sound amplification based on the surrounding environment. Machine learning algorithms analyze auditory inputs in real time, discerning different sound patterns. This means that, for instance, in a noisy restaurant, a hearing aid can automatically reduce background noise while enhancing the clarity of speech from a conversation partner. By employing data from user preferences and feedback, these devices can learn to optimize sound settings for various environments over time, providing a more tailored listening experience.
This self-learning feature is crucial, as it not only responds to immediate conditions but also gathers historical data. Users may find that their hearing aids develop a more personalized sound profile without requiring constant manual adjustments. The implications of such technology extend beyond user convenience; they can significantly impact social interactions, safety, and overall quality of life for individuals with hearing impairments.
Binaural Coordination
Binaural coordination refers to the ability of hearing aids to work together to enhance perception of sound from both ears. Through machine learning, modern hearing aids can communicate with each other, sharing data about the sound environment. This coordination can help users localize sounds better, distinguishing the directionality of noises and conversations. For example, if someone approaches from the left, both hearing aids can dynamically adjust to amplify the sound coming from that direction, providing a more natural hearing experience.
The process is not just about amplifying sound but also about processing it in a coordinated manner. When one hearing aid detects a specific sound pattern, it can inform the other, allowing both devices to react appropriately. This interaction can dramatically improve how wearers perceive their surroundings, increasing situational awareness and reducing the cognitive load associated with trying to comprehend sounds in complex auditory environments.
Through these advancements in adaptive sound processing and binaural coordination, hearing aids powered by machine learning are transforming the auditory landscape. These technologies not only address the core challenges faced by individuals with hearing loss but also pave the way for ongoing innovations in hearing assistance devices.
Personalization through Algorithms
Personalization through algorithms is one of the most pivotal aspects of modern hearing aids empowered by machine learning. This section delves into how tailored solutions enhance user experience and overall device performance. The primary aim here is to provide unique auditory experiences that reflect individual hearing profiles and preferences.
User-Specific Profiles
User-specific profiles are essentially tailored configurations that adapt to individual needs. Each person has distinct hearing abilities, preferences, and environments where they use their hearing aids. Collecting data at the outset, such as audiometric tests and daily listening habits, forms the basis of these profiles.
Machine learning algorithms use this data to create a personalized sound profile for each user. The algorithms can analyze environments and adjust settings automatically. For instance, if someone frequently attends loud events, the hearing aid can enhance speech clarity while reducing background noise in those situations.
Additionally, ongoing adjustments are crucial. As users gain more familiarity with their hearing aids, the information this equipment collects can refine their profiles. This process ensures optimal auditory feedback continually adapts to their preferences, leading to significant satisfaction improvements in hearing aid users.
Machine Learning Feedback Loops
Machine learning feedback loops provide an iterative mechanism for improvement based on user interaction. When users employ their hearing aids, they provide real-time data which the algorithms interpret. This feedback can include user ratings on sound quality or automatic adjustments that are more or less desirable.
These systems learn from every interaction. If a particular setting proves unpopular, the hearing aid can adjust accordingly. This allows for the continuous fine-tuning of the device, enhancing personalization over time. Moreover, such feedback mechanisms help in the detection of user patterns, further enriching their profiles with valuable insights.
The implementation of feedback loops can result in gradual yet significant improvements tailored to the individual's needs, ensuring the technology remains relevant in a dynamic environment.
In summary, personalization through algorithms is indispensable for modern hearing aids. User-specific profiles enable customization based on preferences and requirements, while machine learning feedback loops ensure continuous enhancement of user experience. This thoughtful integration of technology exemplifies how innovation can meet the nuanced demands of hearing aid users.
Benefits of Machine Learning Hearing Aids
The incorporation of machine learning into hearing aids presents a multitude of benefits that not only enhance the technology but also significantly improve user experience. These advancements address various challenges faced by traditional hearing devices. Machine learning algorithms offer smarter processing capabilities, promoting a more tailored listening experience. The benefits include improved sound quality, enhanced user experience, and real-time adaptability. These elements are crucial for individuals with hearing loss who rely on these devices in their daily lives.
Improved Sound Quality
Sound quality is paramount for effective communication. Machine learning contributes to this aspect by enabling adaptive sound processing. Advanced algorithms analyze and interpret the sound environment in real time. This means that the hearing aid can adjust settings instantly based on incoming sounds. Over time, the device learns the user’s preferences and habits. For instance, if a user frequently attends social gatherings, the hearing aid optimizes its settings to enhance speech clarity in noisy environments.
Moreover, machine learning aids in noise reduction technologies. The algorithms can differentiate between speech and background noise. This separation helps to focus on the desired sounds, thus improving clarity. More than just amplifying sounds, machine learning ensures that the amplification is context-aware. This results in a significant enhancement in the overall auditory experience
Enhanced User Experience
User experience is often critical in the perception of hearing aids. Machine learning promotes personalization through user-specific profiles. Each device can adjust its settings based on individual user data. By tracking user behaviors and preferences, the hearing aid can optimize itself for various situations.
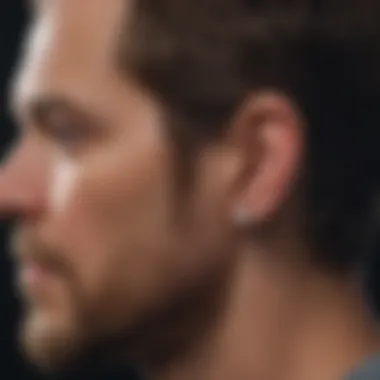
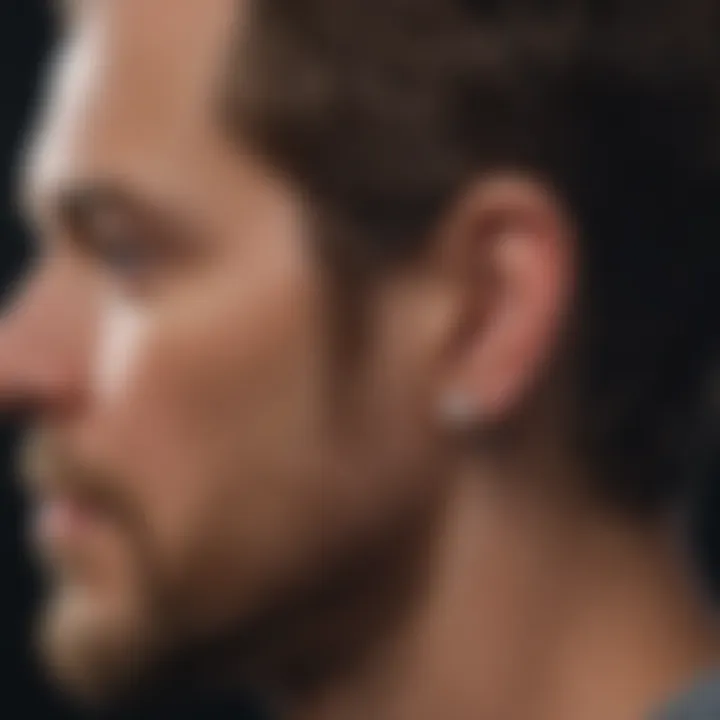
The user interface is also becoming more intuitive, largely due to machine learning. Users can receive alerts or suggestions that guide them on adjustments or settings for different environments. This proactive approach not only makes the devices easier to use but also increases user satisfaction.
Furthermore, the process of fitting these devices is more seamless. Machine learning aids audiologists in understanding user needs and optimizing the fit. Better fitting leads to better sound quality and comfort for users. Overall, the enhanced user experience results in greater adoption and continued use of these devices.
Real-Time Adaptability
Real-time adaptability is a key feature that machine learning brings to hearing aids. Users often encounter various sound environments throughout their day, from quiet rooms to bustling streets. Machine learning enables devices to adapt to these changing conditions without manual input.
The algorithms continuously learn from feedback loops, adjusting parameters on the fly. For example, during a conversation in a crowded area, the hearing aid can automatically reduce the level of background noise while emphasizing the voices around the user. This adaptability not only fosters better communication but also reduces user frustration.
In essence, real-time adaptability allows individuals with hearing aids to engage more naturally with their surroundings. This makes everyday interactions smoother, less taxing, and more enjoyable.
"Machine learning in hearing aids offers a revolutionary shift in how users interact with sound, providing them with tools that evolve alongside their needs."
In summary, the benefits of machine learning integrated into hearing aids are profound. From improved sound quality to a more personalized user experience and effective real-time adaptability, these technologies are changing the landscape of auditory assistance. They offer users a more finely tuned approach to managing their hearing loss, ensuring a clearer and more natural auditory experience.
Challenges in Implementing Machine Learning
The integration of machine learning into hearing aids is not without its hurdles. Although the potential benefits are vast, particularly in improving personal auditory experiences, understanding the challenges is crucial. Recognizing these obstacles helps in developing solutions by addressing the underlying issues that may impede progress.
Data Acquisition and Privacy
Access to a robust dataset is critical for machine learning algorithms to learn and adapt effectively. In the context of hearing aids, this data typically encompasses user behavior, environmental sounds, and specific hearing profiles. However, acquiring such data raises significant privacy concerns. Users often feel apprehensive about sharing sensitive information, fearing potential misuse.
The necessity for data anonymization becomes paramount here. Proper safeguards must ensure that personal information is not traceable back to individuals. Organizations need to clearly communicate to users how their data will be utilized, fostering trust in the technology. Transparency in data collection and utilization, along with obtaining proper consent, are vital steps towards ethical implementation of machine learning in hearing aids.
Key Considerations for Data Acquisition:
- User Consent: Clear and informed consent processes must be put in place.
- Anonymization Techniques: Employ methods to anonymize data to protect user identity.
- Legislation Compliance: Adhere to regulations, such as GDPR, which dictate data usage rights.
Algorithm Bias and Fairness
Another critical challenge lies in algorithm bias and concerns around fairness. Machine learning algorithms can unintentionally reflect the biases of the data they are trained on. For hearing aids, this could result in users receiving less than optimal performance based on demographic factors such as age, gender, or ethnicity.
This bias can lead to unequal access to technology’s benefits, thus raising ethical implications. To mitigate this, developers must prioritize diverse datasets that reflect various population segments. This approach ensures that machine learning models are robust and can serve a wider audience without inherent biases.
Mitigation Strategies for Algorithm Bias:
- Diverse Data Collection: Focus on capturing a wide range of user experiences and backgrounds.
- Regular Model Audits: Continually evaluate and update algorithms to eliminate biases.
- Multidisciplinary Teams: Engage specialists from diverse fields in the algorithm development process to highlight potential biases.
The challenges in implementing machine learning within hearing aid technology require an informed and conscientious approach to ensure accessibility and fairness for all users.
Clinical Considerations
The integration of machine learning into hearing aids complicates the clinical landscape in both exciting and challenging ways. This section looks at essential factors for healthcare providers who work with patients suffering from hearing loss. Addressing clinical considerations lays a foundation for optimal patient outcomes and ensures that the technology serves its purpose effectively.
Hearing Loss Assessment
A precise assessment of hearing loss is critical for the successful fitting and development of machine learning-driven hearing aids. Audiologists typically conduct several tests to determine the type and degree of hearing loss. These assessments usually include pure-tone audiometry, speech recognition tests, and tympanometry. The data collected during this phase informs device selection and ensures that the algorithms utilized in the hearing aids are tailored specifically to the individual’s needs.
Machine learning enhances this assessment by analyzing vast amounts of data from diverse populations. The algorithms can identify patterns that might elude traditional methods. For instance, an audiologist may rely on machine learning algorithms to predict how a patient might respond to different sound environments based on their unique profile. Thus, these algorithms can lead to a more accurate diagnosis, eventually facilitating better fitting outcomes.
"Assessing hearing loss accurately helps tailor machine learning models to users’ individual needs, enhancing overall satisfaction and performance."
Fit and Adaptation Process
Once assessments are complete, the next step is fitting the hearing aid. Machine learning plays a crucial role in this phase too. Each device incorporates algorithms that learn from a user’s experiences. During fitting, audiologists take into account user feedback to adjust settings and optimize sound quality. The adaptability provided by machine learning means that adjustments can often be made in real-time, adapting to various environments without requiring constant manual changes.
Moreover, the adaptation process is not just a one-time event. As users acclimate to their devices, machine learning algorithms continue to collect and analyze data to refine sound processing constantly. For instance, if a user frequently indicates dissatisfaction with certain frequencies, the adaptation can evolve to enhance those specific sounds in various environments. This ongoing adjustment fosters a feeling of ownership and enhances the overall user experience, making it easier for users to feel comfortable and satisfied with their hearing aids.
In summary, clinical considerations during the hearing loss assessment and fitting process underscore the importance of customizing machine learning applications. Audiologists must take an informed approach, understanding both the technology and the unique needs of each patient. This engagement leads to successful outcomes and fosters trust between patient and provider.
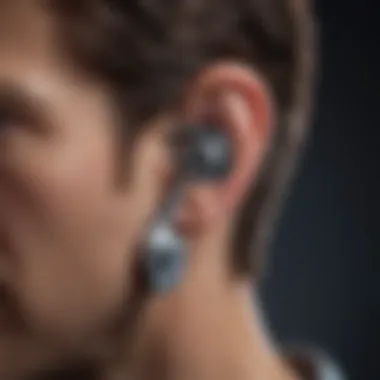
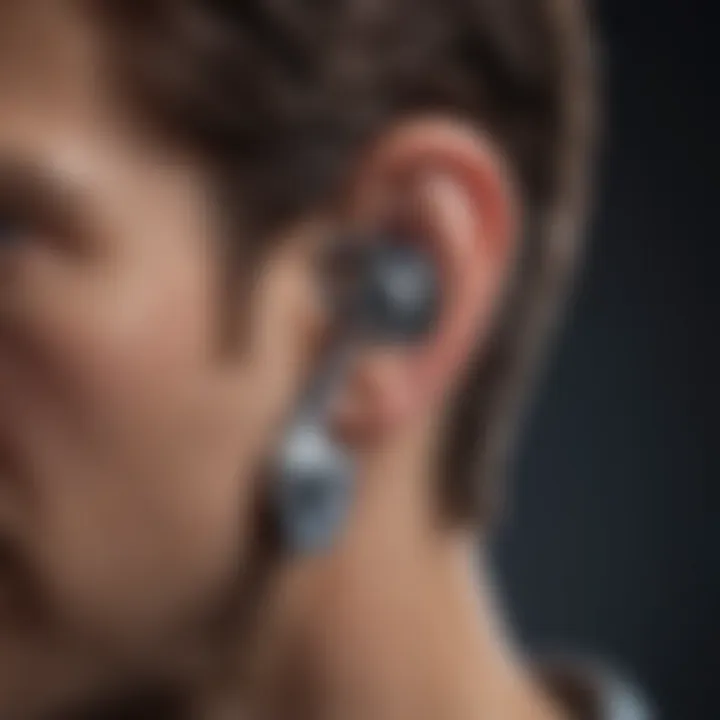
Future Directions in Hearing Aid Technology
The field of hearing aids is on the brink of significant transformation, primarily influenced by machine learning and artificial intelligence. Understanding future directions is crucial for comprehending how these technologies can enhance user experience and functionality. Optimizing hearing aids involves many research areas and potential innovations. This section delves into predicted scientific advances and the role of artificial intelligence in future hearing aid technology.
Predicted Scientific Advances
As technology evolves, so does the scientific understanding of auditory processes and user needs. Some projected advancements include:
- Enhanced Sound Processing: Future hearing aids will feature even more sophisticated algorithms. This would enable real-time sound enhancement that adapts to a user’s unique environment and auditory preferences.
- Biodegradable Materials: Research into eco-friendly materials is growing. Hearing aids may soon be developed using biodegradable alternatives, contributing to sustainability.
- Integration with Other Technologies: The potential for hearing aids to connect seamlessly with other smart devices, such as smartphones and home automation systems, is significant. This will allow for an interconnected experience that enhances usability.
- Brain-Computer Interfaces: In the long term, brain-computer interface technology might be utilized in hearing aids. This could lead to devices that interpret brain signals directly, adjusting to user needs without manual intervention.
These advances are not only theoretical but are actively being researched. They could significantly improve how users engage with their auditory world.
"The potential of combining innovative materials and intelligent algorithms could redefine the role of hearing aids in daily life."
Role of Artificial Intelligence
Artificial intelligence is set to play a crucial role in the next generation of hearing aids. Its applications will likely span across several aspects:
- User-Centric Adaptability: AI-powered hearing aids can learn from user behaviors and preferences. This helps in creating a customized listening experience.
- Improved Noise Reduction: Through continuous learning, AI can dramatically improve sound filtering in noisy environments, allowing users to focus on desired sounds.
- Predictive Diagnostics: AI could enable hearing aids to monitor ongoing user health metrics and alert users or caregivers about potential hearing-related issues.
- Data-Driven Improvements: By constantly gathering and analyzing usage data, manufacturers can refine their products based on real-world user interactions, resulting in smarter devices.
These developments suggest that artificial intelligence will not merely assist but fundamentally change how hearing aids are viewed and utilized.
Through continuous research and innovation, the future of hearing aids looks promising, prioritizing the user’s auditory needs and experiences.
Ethical Implications
The rapid advancement of technology, particularly in machine learning, raises critical ethical considerations, especially in the context of hearing aids. The integration of smart technologies into these devices enhances user experience but also brings forth questions surrounding user consent, data privacy, and equitable access to technology. Understanding these implications is essential for developers, users, and healthcare providers. It ensures that the benefits of these technologies are enjoyed without compromising fundamental ethical standards.
User Consent and Data Use
User consent is a cornerstone of ethical machine learning applications. In hearing aids that utilize machine learning, user data is often needed to train algorithms. This data can include audio recordings, usage patterns, and user preferences. Obtaining informed consent is crucial. Users must clearly understand what data is being collected, how it will be used, and any potential risks involved.
Moreover, the proposed uses of data should be transparent. Users have the right to know if their data will be shared with third parties or used for research purposes. Failure to address these concerns can lead to distrust among users, inhibiting the adoption of advanced hearing aid technologies.
Considerations for user consent include:
- Clear Communication: Manufacturers must present data usage policies in understandable language.
- Opt-In Mechanisms: Users should have the ability to choose if they want to participate in data collection.
- Revocation of Consent: Users should be able to withdraw consent easily if they have concerns about their personal data security.
Equity in Accessing Technology
Equity in accessing hearing aid technology is another pressing ethical issue. While machine learning can create highly personalized auditory experiences, disparities may emerge based on socioeconomic status or geographic location. High costs of advanced hearing aids equipped with machine learning can limit access for many individuals, potentially leading to a widening gap in hearing health outcomes.
Key factors to consider in ensuring equitable access include:
- Affordability: Innovations should aim to balance performance with affordability, ensuring that advancements do not exclude lower-income individuals.
- Availability of Resources: In rural or underserved areas, access to both the devices and the expertise required to fit and maintain them can be lacking.
- Public Policy Efforts: Advocacy for policies that support funding or subsidies for advanced hearing technology can help address disparities.
"As we continue to integrate machine learning into hearing aids, it is paramount to remember the ethical obligations that come with such advancements. Transparency, inclusivity, and fairness are crucial for the responsible development of technology."
By considering ethical implications such as user consent and equitable access, stakeholders can help ensure that machine learning enhances the hearing aid experience without compromising ethical values.
The End
The conclusion serves as a crucial section in articles addressing complex topics like the integration of machine learning in hearing aids. It provides a moment to reflect on the insights gained throughout the text and emphasizes the significance of understanding the evolving relationship between technology and audiology. With medical and technological landscapes continually shifting, articulating these insights strengthens the dialogue around innovative solutions for hearing impairments.
Summation of Key Insights
Machine learning technology has introduced a transformative approach to hearing aids, enhancing user experiences through personalized sound processing. This article has discussed several key themes:
- Adaptive Sound Processing: Machine learning allows for real-time adjustments that help users experience sound more naturally based on their environment.
- Binaural Coordination: The synchronization between devices ensures spatial awareness and improved sound localization, making communication more intuitive.
- User-Specific Algorithms: Tailored solutions based on individual hearing profiles present a new frontier in accessibility, enabling hearing aids to adapt to the unique preferences and challenges faced by each user.
These insights reflect a broader shift in how hearing aids operate, focusing not just on amplification but on creating a seamless auditory experience that evolves with the user’s needs.
Final Thoughts on the Future of Hearing Aids
Looking ahead, the marriage of machine learning and hearing aid technology appears not just beneficial but essential. As algorithmic strategies advance, hearing aids will likely incorporate more sophisticated features that can predict user preferences and contexts. Future developments could include:
- Continued Personalization: Future algorithms will learn and adapt even more efficiently, potentially using more diverse data points for better user profiles.
- Integration with Other Devices: The concept of the Internet of Things promises that hearing aids will become integrated within a broader connected ecosystem, enhancing user interactions.
- Ethical Standards: As technology evolves, maintaining a focus on privacy and user consent will be paramount to ensure trust in these innovations.