Understanding AGI: The Frontier of Artificial Intelligence
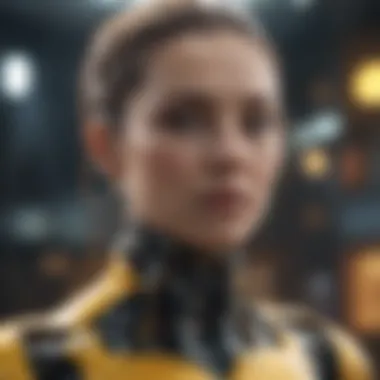
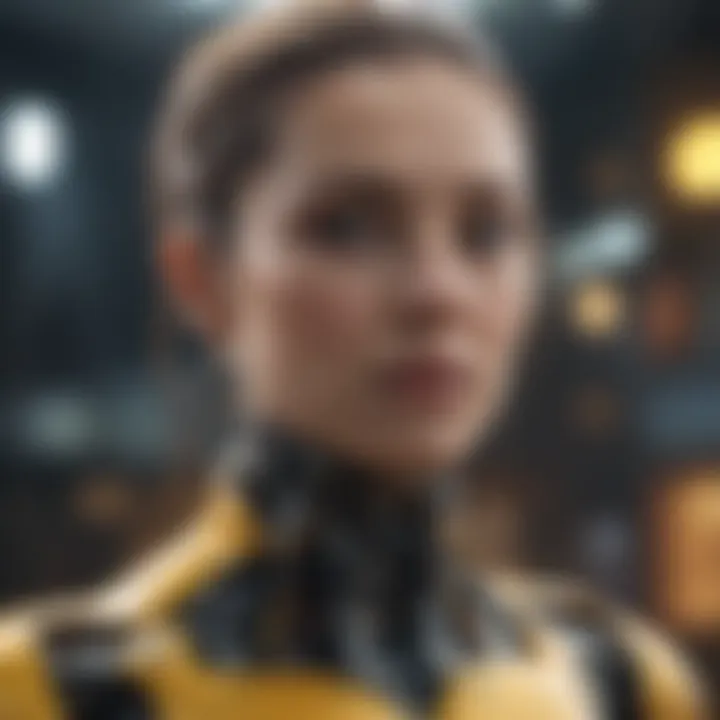
Intro
The pursuit of Artificial General Intelligence (AGI) is an ambitious endeavor within the broader field of artificial intelligence. Unlike narrow AI, which excels in a specific task, AGI represents a system that can understand, learn, and apply knowledge across a wide range of tasks with human-like flexibility. As the conversation around AGI evolves, it is crucial to dissect its layers, from foundational theories to pressing ethical concerns. This sets the stage for an in-depth examination of how AGI could reshape our relationship with technology and impact various sectors.
Research Overview
Key Findings
The exploration of AGI reveals several key findings:
- Definition Dilemma: AGI lacks a universally accepted definition, complicating both theoretical discourse and practical implementation.
- Technological Barriers: Significant technical challenges exist, including the integration of learning algorithms that can operate across different domains efficiently.
- Ethical Considerations: The potential for AGI raises ethical dilemmas, including questions about control, decision-making, and societal impacts.
Study Methodology
The methodology surrounding AGI research employs diverse approaches:
- Literature Review: Comprehensive reviews of existing studies and theoretical frameworks.
- Interdisciplinary Collaboration: Joining fields such as cognitive science, computer science, and philosophy to enhance understanding.
- Practical Experiments: Engaging in simulations and prototypes to test AGI theories in realistic settings.
Background and Context
Historical Background
The concept of AGI dates back to the mid-20th century, driven by pioneers like Alan Turing and John McCarthy. Initially, the goal was to create machines capable of rational thought. Over the decades, the trajectory of AGI shifted from theoretical discussions to practical experiments, though progress encountered numerous limitations. Notable milestones include the development of the first neural networks in the 1960s and later advances in machine learning in the 1990s.
Current Trends in the Field
Today, AGI research is marked by accelerated advancements:
- Deep Learning: This technology has enhanced the machine’s ability to learn from vast datasets and improve performance.
- Collaborative AI: Systems are increasingly designed to work alongside humans, creating a synergy that can lead to exponential learning.
- Global Initiatives: Various organizations, including Google DeepMind and OpenAI, are competing to make significant strides toward achieving AGI, reflecting both commercial and academic interest.
AGI stands at the frontier of artificial intelligence, beckoning researchers, ethicists, and technologists to navigate its complexities, possibilities, and inherent risks. The implications of developing a true AGI are expansive, warranting a thoughtful approach to its realization.
Defining Artificial General Intelligence
Artificial General Intelligence (AGI) represents a pivotal concept in the burgeoning field of artificial intelligence. It refers to the ability of a machine to understand, learn, and apply intelligence across a broad range of tasks, similar to human cognitive capabilities. This section is vital as it lays the groundwork for understanding the nuances that differentiate AGI from more specialized forms of AI, often termed Narrow AI.
The conceptual framework for AGI encompasses various dimensions, including its potential capabilities, the types of problems it could solve, and the significant benefits it may bring to multiple domains. Unlike narrow AI, which excels in specific tasks, AGI aspires for broader applicability in diverse areas. This ambition makes defining AGI integral to comprehending its implications for society, technology, and the economy.
Distinction Between AGI and Narrow AI
Narrow AI, characterized by its expertise in singular tasks, contrasts sharply with AGI. While narrow AI can outperform humans at specific activities like data analysis, image recognition, or language translation, it lacks the versatility or contextual understanding intrinsic to AGI. For instance, a narrow AI tool such as Google Translate can translate languages effectively, yet it cannot engage in conversation or comprehend cultural nuances.
In contrast, AGI would enable machines to tackle diverse challenges, leveraging a holistic understanding of information. This shift marks a significant evolution in the landscape of artificial intelligence, suggesting a future where machines can think, learn, and make decisions autonomously across various fields.
Core Characteristics of AGI
Autonomy
Autonomy is a crucial aspect heralded in discussions about AGI. A defining attribute, it allows systems to operate independently without constant human intervention. The potential of autonomous systems to make decisions presents numerous applications, from self-driving cars to intelligent chatbots that can navigate complex customer service interactions.
However, autonomy also introduces challenges. If machines can operate independently, concerns about control and accountability arise. In this light, ensuring ethical frameworks and guidelines is essential in technology development, as the balance between empowering autonomous systems and maintaining oversight remains paramount.
Adaptability
Adaptability stands as another significant trait of AGI. This characteristic enables machines to adjust their functions based on new information or changing environments. Such flexibility is critical in dynamic settings where unexpected variables can impact outcomes. For example, an AGI system used in healthcare can learn from each patient interaction, improving its diagnostic capabilities over time.
The unique advantage of adaptability lies in its potential to enhance performance in real-world scenarios. Confirming this attribute can foster innovation across fields, although it may also raise concerns about reliability, particularly when systems continuously evolve and learn.
Reasoning
Reasoning encapsulates the cognitive functions behind decision-making in AGI. It involves the ability to evaluate situations, infer conclusions, and devise solutions based on data analysis. This intellectual capacity is what sets AGI apart from existing AI systems, which often lack deep analytic abilities.
The inclusion of reasoning means AGI can tackle complex problems requiring comprehensive comprehension and critical thought. The path toward achieving this capability involves significant research and development, but realizing it could revolutionize industries such as finance, education, and healthcare.
"The pursuit of AGI is not just about creating intelligent machines; it is fundamentally about redefining our understanding of intelligence itself."
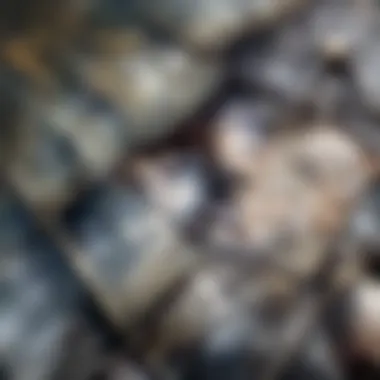
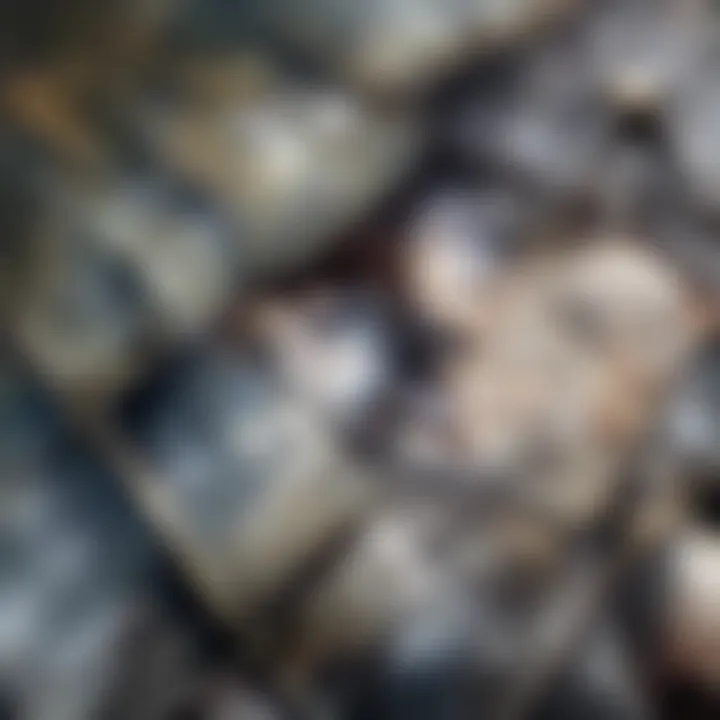
In sum, defining AGI requires an in-depth analysis of its core characteristics, including autonomy, adaptability, and reasoning. These traits contribute immensely to the promise of AGI, highlighting the need for careful consideration as research progresses.
Historical Development of AGI
The historical development of Artificial General Intelligence (AGI) is crucial for understanding its trajectory and current state. This section explores various milestones in AI research, setting a foundation for recognizing both where AGI has come from and where it might be headed. Each developmental phase lays essential groundwork for the ambitions and challenges presented in creating AGI, which could transform numerous sectors.
Milestones in AI Research
Early Theories
Early theories in artificial intelligence paved the way for the exploration of general intelligence. The work of pioneers, like Alan Turing and John McCarthy, established fundamental principles that guided future research. Their ideas about logic, computation, and decision-making presented a framework for envisioning machines capable of intelligent behavior. A key characteristic of these early theories was their focus on symbolic reasoning, which illustrated how machines could simulate human thought. This aspect is beneficial because it lays a clear groundwork for understanding AGI’s potential, even if it lacks the complexity of modern approaches. However, one disadvantage is that early theories sometimes oversimplified human cognition, leaving gaps in addressing emotional and social contexts of human intelligence.
Key Projects
Key projects like the Dartmouth Workshop in 1956 were significant landmarks in AGI history. These gatherings pushed the boundaries of AI research by fostering collaboration among various scientists. The key characteristic of such projects is their interdisciplinary nature; they encouraged insights from computer science, psychology, and philosophy. This intersection allowed for a more holistic view of intelligence, which is valuable for shaping AGI. However, the unique feature of these collective efforts was their limited scope, as they tended to focus on specific tasks rather than a comprehensive understanding of intelligence.
Influential Models
Influential models like neural networks and expert systems marked important developments in AGI research. These models demonstrated practical applications of AI, driving further exploration into machine learning. A notable characteristic of these models is their adaptability, allowing systems to learn from data over time. This characteristic provides a significant advantage for creating AGI, as learning is fundamental to any intelligent agent. However, one limitation is that early models often struggled with scalability and real-world applicability, leading to skepticism about achieving true general intelligence.
Evolution of AGI Concepts
Pre-AGI Theories
Before the term AGI became mainstream, various pre-AGI theories explored the concept of intelligence in machines. These theories ranged from simple algorithms to complex logical frameworks. A key characteristic of pre-AGI theories is their focus on replicating specific human cognitive functions. This focus is beneficial as it provided insight into the mechanisms of thought and problem-solving. However, the limitation of these theories is their often narrow approach, which failed to account for the intricate nature of human cognition and creativity.
Contemporary Approaches
Contemporary approaches to AGI take a more integrated view, combining traditional AI methods with advances in technology. These approaches include techniques such as deep learning and reinforcement learning, seemingly moving closer to creating AGI. A key characteristic of contemporary strategies is their reliance on vast data sets and powerful computational resources. This is advantageous, allowing for the training of more robust and intelligent systems. However, a unique challenge remains: the ethical and philosophical implications of creating systems that may surpass human intelligence.
Understanding the historical development of AGI provides pivotal insights into its potential, challenges, and ethical considerations, fundamentally shaping the future of artificial intelligence.
Current State of AGI Research
The exploration of Artificial General Intelligence (AGI) is gaining momentum in recent years. This section reflects on the current landscape of AGI research, stressing its significance in shaping future possibilities. As researchers delve into this field, they uncover challenges and breakthroughs that lay the groundwork for the future of intelligence in machines. The synergy between academic institutions and private entities fosters a comprehensive environment for innovation.
Ongoing Projects and Innovations
Leading Research Institutions
Leading research institutions play a vital role in the advancement of AGI. These organizations provide resources, expertise, and an environment conducive to groundbreaking innovations. Prominent institutions, such as MIT and Stanford University, focus on interdisciplinary approaches combining computer science, psychology, and neuroscience. Their reputation attracts top talent and fosters an ecosystem where ideas thrive.
A specific feature of these institutions is their collaboration with industry. They often partner with technology companies to further AGI research. This leads to a beneficial exchange of knowledge, ideas, and technologies. For example, MIT's partnerships with Google and IBM create avenues to apply theoretical research to practical applications.
However, reliance on institutional support can lead to the challenge of balancing academic pursuits with commercial interests. This tension may affect the direction of research, emphasizing outcomes appealing to investors over pure scientific discovery.
Private Sector Developments
The private sector is a key contributor to AGI research. Companies like OpenAI and DeepMind are pushing the boundaries of what is possible in this realm. Their focus on developing algorithms that can adapt and learn autonomously places them at the forefront of innovations in AGI.
The significant characteristic of these private entities is their ability to scale quickly. They can implement findings from research into products rapidly. This speed not only brings innovations to market sooner but also garners considerable investment, driving further progress.
However, a potential drawback is that profit motives may overshadow ethical considerations. The pressure to deliver rapid results can lead to practices that prioritize speed over safety or societal impact. This raises concerns about long-term implications of AGI technology.
Technologies Driving AGI
Machine Learning
Machine learning is at the core of AGI development. It allows systems to learn from data without explicit programming. Its ability to analyze vast amounts of information makes it essential for progress in AGI.
A critical feature of machine learning is its versatility. It can apply to various fields like finance, health care, and robotics, making it a popular choice. Its unique feature is the ability to improve performance over time through iterative learning.
Nevertheless, machine learning's dependency on large datasets raises issues. Large amounts of quality data are required for effective learning, which can be a barrier for smaller institutions or startups. This reliance on data also might introduce biases if the data is not representative.
Neural Networks
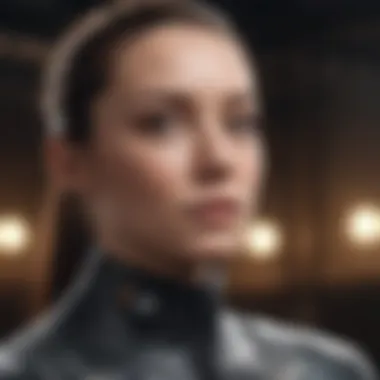
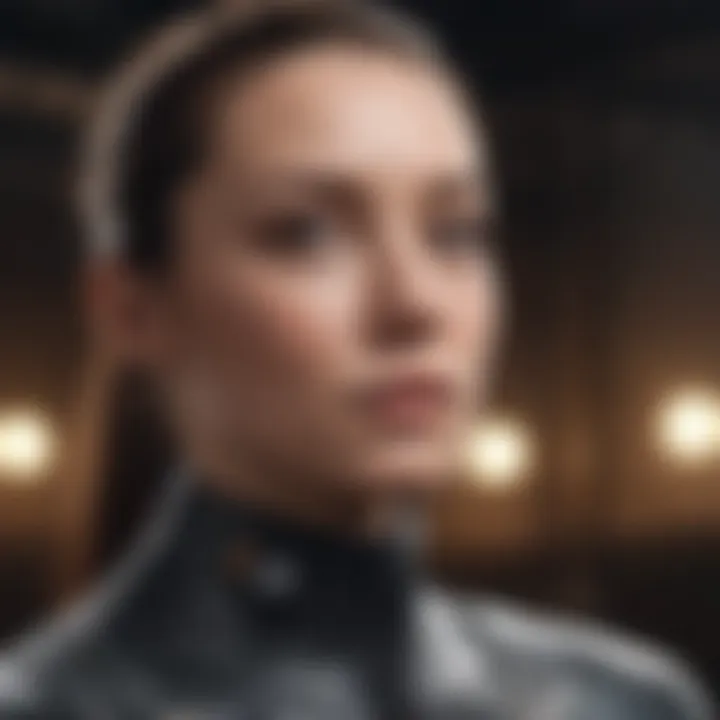
Neural networks mimic human brain functionality, making them crucial for AGI. They excel in recognizing patterns, similar to how humans learn from experience. Their ability to process unstructured data, like images and speech, makes them particularly powerful.
One appealing aspect of neural networks is their deep learning capability. This depth allows for complex problem-solving but requires extensive computational resources. The unique advantage is their suitability for tasks that were previously thought to be too complex for machines.
That said, neural networks can suffer from transparency issues. The so-called "black box" problem makes it hard to understand how decisions are made within a network, raising concerns about accountability and trustworthiness in AGI systems.
Natural Language Processing
Natural language processing (NLP) is another significant technology driving the path towards AGI. It enables machines to understand and generate human language, which is fundamental for human-computer interaction.
A key characteristic of NLP is its applicability in various contexts, such as chatbots and virtual assistants. This versatility increases user engagement and provides a more intuitive interface for technology.
Yet, NLP also faces challenges. Understanding context and managing nuance in language are complex tasks that remain difficult. Misinterpretations can lead to confusion, impacting user experience and limiting the technology’s effectiveness.
"The path to AGI is marked by both progress and challenges. Understanding the current state informs our expectations and ethical considerations regarding future developments."
Challenges in Achieving AGI
The pursuit of Artificial General Intelligence (AGI) is not without its hurdles. Understanding these challenges is essential for anyone involved in AI research or its application. In this section, we will explore the technical limitations and philosophical dilemmas that hinder the journey to achieving AGI.
Technical Limitations
Data Requirements
Data requirements play a critical role in the development of AGI. Models need vast amounts of data to learn effectively. This necessity creates a reliance on high-quality data sets. The sheer volume of data is often overwhelming. It can make the training process challenging and resource-intensive. Big data provides a wealth of examples for machines to learn from. Without adequate data, the capability of an AGI system is severely limited. The key characteristic of these data requirements is their scale. The more data, the better the learning.
However, this reliance on data has its drawbacks. For instance, sourcing high-quality, diverse data can be time consuming and diminish progress. Additionally, data privacy and security issues arise. These considerations make the handling of data a complex aspect of AGI's development.
Computational Power
Computational power is another significant technical limitation in achieving AGI. The need for high-performance computing resources is undeniable. AGI systems need to process and analyze vast amounts of data in real time. This requires a scale of computational power that is often beyond what is currently available.
The characteristic of computational power hinges on hardware capabilities. Advanced processors and high-speed processors enhance machine learning models. The unique feature of this requirement is that it continues to evolve. As AGI concepts progress, so does the need for more powerful systems. One important disadvantage of this situation is the cost involved—upgrading technology demands significant financial investment. Additionally, the energy costs associated with high-performance computing can also be a concern, especially when considering environmental impacts.
Philosophical Dilemmas
Consciousness in Machines
Consciousness in machines poses significant philosophical challenges. The question of whether machines can ever truly be conscious remains unsettled. This query influences how we perceive the potential of AGI. A key characteristic of consciousness is subjective experience. Understanding if machines have any such experience is critical for AGI's development.
Considering machine consciousness raises concerns about ethics and responsibility. What rights, if any, should a conscious machine possess? This leads to many unanswered questions. These philosophical aspects complicate the technical pursuit of AGI. Machines may have advanced data processing capabilities, but can they possess awareness?
Understanding Intelligence
Understanding intelligence is foundational to progress in AGI's landscape. Intelligence itself is complex and multifaceted. How can we define it in a way that encompasses human-like traits yet remains applicable to machines? The key characteristic here is its varying definitions. As research continues, various theories attempt to encapsulate what constitutes intelligence. This situation makes the standardization of AGI goals challenging.
The unique feature of the concept of intelligence is its subjective nature. Different cultures and disciplines may view intelligence differently. This subjectivity complicates expectations of what AGI should achieve. As a result, achieving consensus on how to guide AGI development becomes problematic. Misalignment in goals can stifle innovation and cause significant delays, thereby prolonging the achievement of true AGI.
Ethical Considerations of AGI
The ethical implications surrounding Artificial General Intelligence (AGI) are profound and multifaceted. As we advance in our capabilities to create machines that can reason, learn, and make decisions autonomously, it becomes essential to systematically explore the moral frameworks governing their development and deployment. Understanding these ethical considerations is crucial not just for researchers and developers, but also for the broader society that will be impacted by these emergent technologies.
The significance of this topic lies in its potential to influence regulations, market innovations, and public safety policies. The choices made in the ethical domain shape the landscape of AGI. From issues of unemployment due to job displacement to the potential for misuse in military applications, these challenges require careful thought and planning.
Potential Risks and Misuses
Autonomy and Control
Autonomy in AGI refers to the ability of a system to operate independently, making decisions without human intervention. This characteristic raises critical questions about control. Who retains the authority over AI when it acts autonomously? Such questions influence the framework of safe AGI usage.
One of the key characteristics of autonomy is its reliance on data input and machine learning processes, which can sometimes lead to unexpected decision-making outcomes. A beneficial aspect of this is the ability of AGI to optimize processes and enhance efficiency. However, a disadvantage lies in the potential for misalignment between human values and the decision-making frameworks of an autonomous AGI.
"The progress in AGI presents a unique challenge in the balance of control between human operators and autonomous systems."
Security Issues
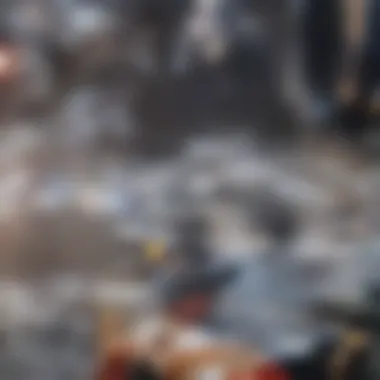
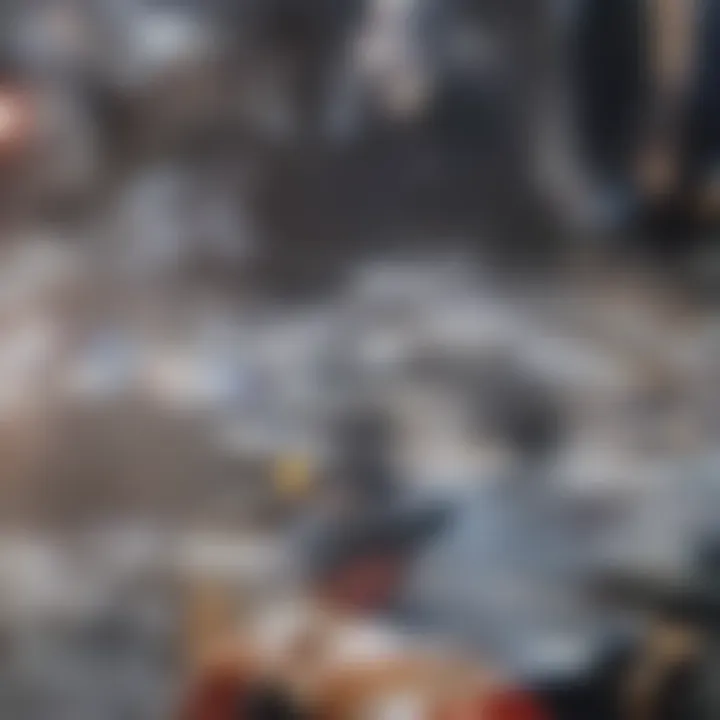
Security issues in AGI revolve around the risks of malicious exploitation and vulnerabilities in the systems. A significant concern is how AGI systems could be weaponized or turned against their creators.
The key characteristic of this aspect is its unpredictability. AGI systems operate based on patterns and data processing, which can be difficult to monitor or control. This unpredictability makes security a pressing concern for developers and stakeholders. The advantage of building strong security frameworks into AGI is the potential to mitigate threats proactively. However, it can prove to be a double-edged sword as too many restrictions might limit the system's abilities, thus affecting its overall functionality.
Regulatory Frameworks
Global Standards
Global standards refer to the essential guidelines and regulations developed internationally to govern AGI technology. The importance of setting these standards lies in ensuring safe and responsible use across various jurisdictions. Establishing global standards can create uniformity in ethical practices and technical specifications.
A key characteristic of these standards is their basis in broad collaboration among countries, corporations, and academic institutions. This can be a beneficial approach, as it helps prevent technological disparity and encourages best practices. However, the challenge often comes from differing legal systems and cultural perspectives on technology.
Policy Recommendations
Policy recommendations are strategic frameworks developed to aid in navigating the complexities of AGI implementation. These recommendations contribute significantly to informing governments and organizations on best practices.
The key characteristic of these policies is their focus on both immediate needs and long-term implications. This forward-thinking aspect is beneficial in ensuring that AGI technologies develop in alignment with ethical and societal values. Nonetheless, formulating comprehensive policies can be a challenging process, requiring significant resources and time to adapt to rapidly changing technological landscapes.
In summation, exploring the ethical considerations of AGI presents a vital opportunity for researchers, policymakers, and society at large. Addressing these issues is not merely academic but has real-world implications that extend beyond technology into the very fabric of human interactions with AI.
Future Implications of AGI
The concept of Artificial General Intelligence (AGI) continually beckons exploration, raising questions about its potential role in our future society. Understanding the implications of AGI is crucial because it can fundamentally alter the fabric of economic, social, and technological domains. As AGI technologies advance, they will bring about profound transformations, highlighting both opportunities and challenges that need careful consideration.
Impact on Employment
Job Displacement
One notable aspect of job displacement concerns the automation of numerous industries. AGI's capabilities can lead to efficient processes that reduce the need for human labor. This tendency poses a sincere threat to certain occupations, particularly those that require repetitive tasks. Automated systems can perform these tasks more quickly and with higher accuracy. As a result, this may lead to significant job losses.
Many individuals fear for their job security, as AGI becomes more integrated in the workplace. However, viewing job displacement solely as a negative is not entirely adequate. The prospect of efficiency gains cannot be ignored. In this context, embracing technology may enhance productivity and lower operational costs, benefitting organizations in the long run.
New Opportunities
On the contrary, the emergence of AGI also heralds new opportunities across various sectors. As certain roles become obsolete, new job categories will arise, primarily related to the development, maintenance, and oversight of AGI systems. These positions require technical skills, which may lead to a demand for training and education.
Moreover, job creation can occur in industries yet unknown. The evolution of AGI might facilitate innovations, enabling professionals to pursue roles in fields like AI ethics and safety. These opportunities emphasize a balanced view of AGI's impact on employment, recognizing that adaptation is vital for maximizing its potential benefits.
Advancements in Various Fields
Healthcare
In the healthcare arena, the implications of AGI can be transformative. AGI systems could enhance diagnostic accuracy and patient treatment protocols, leading to better healthcare outcomes. The ability to analyze vast datasets at incredible speed allows for more personalized treatment plans, ultimately improving overall patient care.
However, reliance on these technologies raises ethical concerns about patient data security and the dehumanization of care. Balancing technology's benefits against these concerns is critical to ensuring patient welfare.
Education
AGI could redefine education by personalizing the learning experience. With tailored content and adaptive teaching methods, students may achieve higher levels of understanding. Educators can leverage AGI-driven tools to identify students' unique learning styles, allowing for optimized instruction.
Despite these advantages, significant challenges remain. The necessity for educators to adapt to these technologies and the equity of access to AGI-driven educational tools requires careful scrutiny.
Transportation
In transportation, AGI stands to revolutionize how we navigate and manage the movement of goods and people. Autonomous vehicles relying on AGI can minimize accidents caused by human error and streamline traffic systems. This development could substantially decrease congestion and enhance overall safety on roads.
Yet, the shift to AGI-driven transportation raises concerns around job displacement for drivers, alongside the societal implications of automated systems. Addressing these issues will be essential to fully realize the benefits AGI can offer in this field.
"AGI has the potential to reshape industries, but the challenges it presents cannot be overlooked."
Navigating the future implications of AGI involves embracing both the possibilities and the responsibilities that come with such advanced technologies. Understanding this duality will be essential for fostering a society that benefits from AGI while being mindful of its challenges.
Epilogue
The conclusion of this article serves as a critical summation of the insights gleaned about Artificial General Intelligence (AGI). Through examining its definitions, historical context, current state, challenges, ethical dimensions, and future implications, we gain a nuanced view of AGI's potential and significance. The discussions of the various characteristics distinguishing AGI from narrow AI illustrate the complexity of achieving true general intelligence.
The historical landmarks in AI research provide valuable context for understanding where AGI stands today. It is essential to recognize how these milestones have informed contemporary theories and practical applications in the realm of AI.
Furthermore, the ongoing challenges surrounding technical limitations and philosophical dilemmas cannot be understated. They demand a concerted effort from researchers and policymakers alike to navigate the terrain of AGI responsibly. Ethical considerations play a significant role in shaping the discourse surrounding this technology. The potential risks, such as autonomy and misuse, combined with the pressing need for a regulatory framework, highlight that the path forward is fraught with considerations that extend beyond technical capability.
As we look to the future, the implications of AGI on various fields — including employment, healthcare, and education — suggest profound changes are on the horizon. Understanding these dynamics equips students, researchers, educators, and professionals with the tools they need to participate thoughtfully in discussions about AGI.
In summary, the importance of this conclusion lies in its ability to synthesize diverse elements of AGI, making clear that the evolution of this technology is not merely a technical challenge but also a societal one. As AGI continues to evolve, ongoing engagement with its challenges and implications will be necessary to harness its full potential while safeguarding human values.