Understanding Statistics: Foundations and Implications
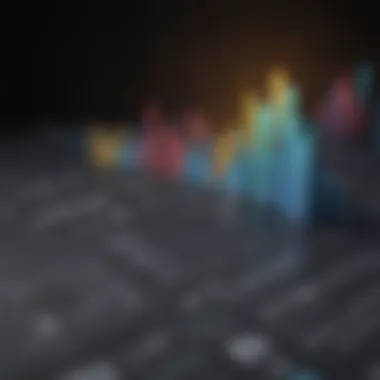
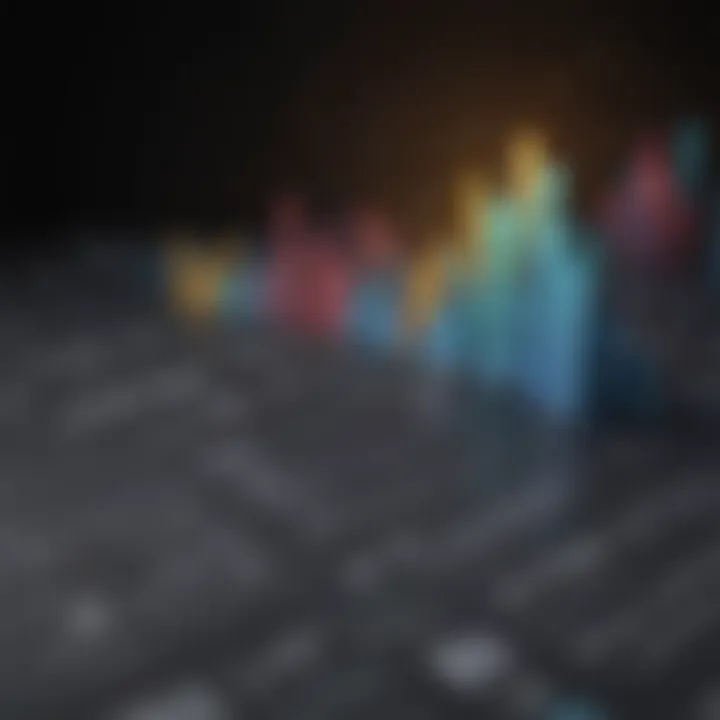
Intro
Statistics is fundamental in many fields, from science to social studies. It allows us to grasp complex data and inform decisions. Whether it is about healthcare, economics, or education, understanding statistics is key to interpreting the world.
In this article, several insights into statistics will be explored, covering foundational principles, applications, and ethical considerations. By understanding the basics, individuals can use statistical methods effectively in their research and professional work.
Research Overview
In this section, we will look at the important findings in the field of statistics. We will also review methodologies that are commonly used in statistical analysis.
Key Findings
Researchers have identified many critical aspects of statistics that are essential for valid interpretations of data. Some key findings include:
- Importance of Data Quality: The accuracy of any statistical conclusion hinges on the quality of the data collected.
- Role of Sample Size: Larger sample sizes tend to yield more reliable results, minimizing the margin of error.
- Impact of Bias: Understanding and mitigating bias in data collection is crucial for the authenticity of research outcomes.
Study Methodology
Common methodologies in statistics include:
- Descriptive statistics: This involves summarizing data through numbers such as means and medians.
- Inferential statistics: This allows for generalizations about a population based on sample data using techniques such as hypothesis testing.
- Predictive analytics: This involves forecasting future trends based on historical data.
Background and Context
To really appreciate the importance of statistics today, it's useful to understand its history and current trends.
Historical Background
Statistics has a long history, evolving from early attempts to quantify and understand population demographics to complex analytical techniques used in various sectors today. Its growth has been linked closely to the advancement of technology and mathematics. In ancient times, people gathered basic numerical data for administrative purposes.
Current Trends in the Field
Today, statistics is widely applied across different fields:
- Machine Learning and AI: Statistical methods are foundational to algorithms that drive artificial intelligence.
- Healthcare: Statistics help in analyzing the effectiveness of treatments and understanding public health trends.
- Social Media Analytics: Companies now gather enormous amounts of data, using statistics to gauge user engagement and preferences.
With the rise of big data, the demand for statistical literacy has never been higher. Understanding the implications of data and statistical methods is crucial for informed decision-making in society.
Prologue to Statistics
Statistics serves as a fundamental pillar in numerous fields, bridging the gap between data and informed decision-making. The systematic analysis of data not only aids in understanding complex situations but also supports hypothesis testing, empirical research, and making predictions. Through this section, we will outline the definition of statistics, its significance across various disciplines, and the historical context that has shaped its evolution.
Definition and Importance
Statistics can be defined as the science of collecting, analyzing, interpreting, presenting, and organizing data. Its primary goal is to provide insights and understanding from data through various methodologies. This discipline encompasses both descriptive and inferential techniques that allow researchers and practitioners to draw conclusions based on samples or entire populations.
The importance of statistics cannot be overstated in today’s data-driven world. Businesses utilize statistics to gauge consumer behavior, government agencies rely on statistical data for policy-making, and healthcare professionals employ statistical tools to improve patient outcomes. By understanding statistical concepts, researchers can avoid the pitfalls of incorrect analyses and misinterpretations that can lead to inaccurate conclusions. Moreover, sound statistical practices lead to better research outcomes, enhancing credibility and effectiveness across various fields.
Historical Context
The development of statistics has roots that stretch back to ancient civilizations. In early history, societies used basic forms of statistical methods for censuses, leading to the collection of data on populations. The term "statistics" itself emerged in the 18th century, evolving from the Latin word "status," which means state or condition. This era marked the beginning of more formal data collection and analysis practices.
The 19th century saw significant advancements in statistical techniques. Pioneers like Karl Pearson and Ronald A. Fisher laid down methods still used today, including correlation coefficients and analysis techniques that form the backbone of modern statistical analysis. The historical context underscores the evolution of statistical methods from simple observations to complex analytical tools.
In understanding statistics, one recognizes its journey through history, while grasping its essential role in contemporary society. Thus, as statistics continue to evolve with technology, its fundamental principles remain crucial for anyone engaged in data-driven analysis.
Key Concepts in Statistics
Understanding key concepts in statistics is essential for interpreting data correctly and making informed decisions. These concepts lay the groundwork for statistical studies and help in enhancing analytical capabilities. Accurate comprehension of these principles is vital in various fields, such as healthcare, social sciences, and business. Misunderstanding the core ideas can lead to misinterpretation of research findings, which can have serious implications.
Population vs. Sample
The distinction between population and sample is a fundamental concept in statistics. The population refers to the entire group of individuals or items that one wants to study. It could be all the residents of a city or every unit of a specific product. Gathering data from the whole population may not always be practical due to time, cost, or logistical constraints. Therefore, researchers often work with a sample, which is a subset of the population.
The sample should ideally represent the population to ensure that conclusions drawn are valid. A poor choice of sample can result in biased results, making the findings unreliable. Sample size also plays a critical role. Larger sample sizes generally lead to more accurate and reliable results, reducing the margin of error.
It's important to use appropriate sampling methods, such as random sampling or stratified sampling, to enhance the representativeness of the sample. Here are a few key points about population and sample:
- Population encompasses all subjects of a study.
- Sample is a selection from the population.
- Representative samples allow for generalization of results.
- Larger samples typically yield more reliable results.
Variables and Data Types
Another significant aspect of statistics involves understanding different variables and data types. A variable is any characteristic, number, or quantity that can be measured or counted. Variables can be classified into various types, which include qualitative and quantitative variables.
- Qualitative variables (or categorical variables) describe qualities or characteristics. They can be nominal (no particular order, e.g., gender, colors) or ordinal (implying order, e.g., rankings).
- Quantitative variables (or numerical variables) are numerical and can be further divided into discrete (whole numbers, e.g., number of students) and continuous (any value within a range, e.g., height or temperature).
Understanding variables is crucial for selecting appropriate statistical methods and analyses. Other considerations include:
- Correct identification of variable types informs analytical techniques.
- Choosing the right data type affects how data should be processed and analyzed.
- Data types influence visualization and interpretation.
In summary, a solid grasp of population versus sample distinctions and understanding various variables and their types are vital components of statistics. They form the basis for data collection, analysis, and interpretation, ensuring that conclusions are valid and meaningful.
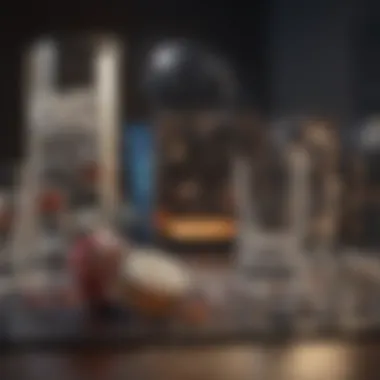
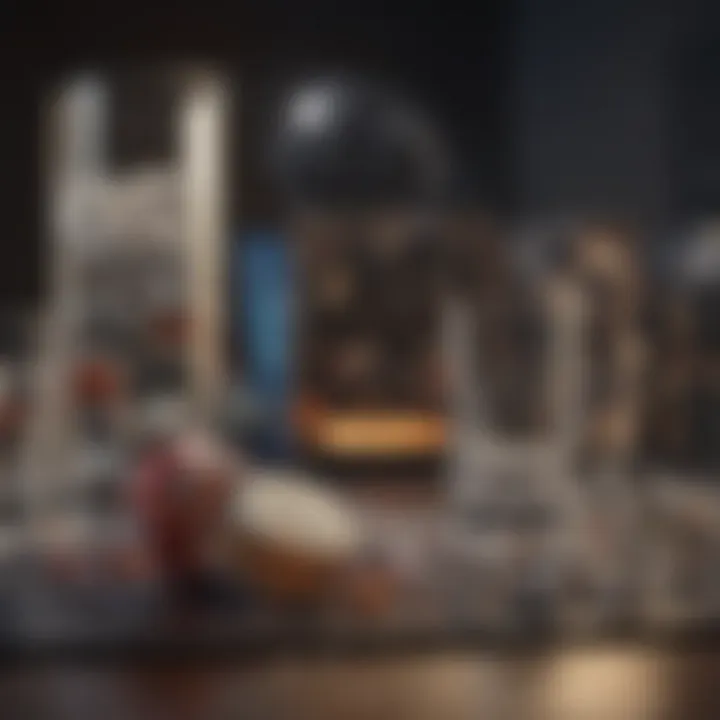
Descriptive Statistics
Descriptive statistics play a critical role in summarizing and interpreting data. This branch of statistics is essential because it provides simple summaries about sample and population data. It helps researchers and practitioners convey complex data sets in a more understandable way. The insights gained from descriptive statistics can help identify trends, patterns, and outliers within the data. This is especially beneficial in fields like health sciences, social sciences, and business, where making data-driven decisions is crucial.
Measures of Central Tendency
Measures of central tendency are key in descriptive statistics. They provide a single value that represents the entire dataset, indicating where most data points tend to cluster. These measures include the mean, median, and mode, each offering unique insights.
Mean
The mean, or average, is calculated by summing all observations and dividing by the number of observations. Its contribution lies in its ability to provide a simple overall estimate of a dataset. A primary characteristic of the mean is that it takes all data points into account, making it a universal representative of the data.
However, the mean can be sensitive to outliers. For instance, in a dataset with extreme values, the mean might not accurately reflect the majority of the data. This makes understanding its advantages and limitations critical when analyzing data.
Median
The median represents the middle value when a data set is arranged in ascending or descending order. This measure is particularly useful in skewed distributions, where extreme values can mislead the mean. The primary characteristic of the median is its robustness against outliers, making it a beneficial choice in many scenarios.
For example, in income data where a few individuals earn significantly more than the rest, the median offers a more accurate reflection of typical income levels. However, it does not take into account the actual values of all data points, which can be seen as a disadvantage when considering total data characteristics.
Mode
The mode is the value that appears most frequently in a dataset. Its importance lies in identifying the most common observation and is particularly useful when dealing with categorical data. A key characteristic of the mode is that it can have multiple values in multimodal distributions, which can provide deeper insight into the data's distribution.
However, relying solely on the mode can be limiting, especially in numerical datasets, where it might not indicate the central tendency effectively if the data is not evenly distributed. Understanding when to use the mode is essential for accurate data analysis.
Measures of Dispersion
Measures of dispersion provide insight into the spread or variability of a dataset. They complement measures of central tendency by indicating how much the data points differ from one another.
Range
The range is the difference between the maximum and minimum values in a dataset. Its simplicity makes it easy to calculate, but it only considers the two extreme values. While providing a quick snapshot of variability, the range can be misleading in datasets with significant outliers. Therefore, it should be interpreted cautiously.
Variance
Variance quantifies how far each number in the set is from the mean and thus from every other number in the set. A higher variance indicates a wider spread of scores. This measure is essential for understanding data variability, although it can be more difficult to interpret because it is in squared units. Variance serves as a foundational element for many statistical tests and procedures.
Standard Deviation
Standard deviation, the square root of variance, offers a more intuitive measure of dispersion as it reflects the average distance of each data point from the mean in the same unit as the data itself. It is widely used because it conveys a clear sense of distribution spread. However, like variance, it can be influenced by outliers, and depending on the nature of the dataset, might not fully represent the underlying distribution.
Inferential Statistics
Inferential statistics plays a critical role in research, allowing scientists and analysts to make predictions or inferences about a population based on a sample of data. Understanding this topic is essential for those seeking to grasp how conclusions are drawn from statistical analyses. Inferential statistics enables researchers to extend findings from a sample to a broader context. This ability is especially important in fields such as medicine, social sciences, and economics, where making decisions based on partial information is often necessary.
Hypothesis Testing
Null and Alternative Hypothesis
The null and alternative hypotheses form the backbone of hypothesis testing. The null hypothesis typically represents a statement of no effect or no difference, while the alternative hypothesis posits that something is different or effective. This structure is crucial because it establishes a framework that guides the research. In this article, the use of null and alternative hypotheses is beneficial because it allows the researcher to formulate clear, testable predictions.
The key characteristic of these hypotheses is their oppositional nature. When conducting a test, one aims to gather evidence that supports the alternative hypothesis while simultaneously testing the validity of the null. The unique feature here is the rejection or failure to reject the null hypothesis based on sample data.
Its main advantage in research is providing a systematic approach to making decisions about data significance. Nevertheless, it is not without disadvantages; incorrectly rejecting the null hypothesis can lead to Type I errors, misrepresenting the findings.
Type and Type Errors
Type I and Type II errors are critical concepts in hypothesis testing. A Type I error occurs when the null hypothesis is incorrectly rejected, suggesting a false positive. A Type II error, on the other hand, arises when the null hypothesis is not rejected, indicating a false negative. Understanding these errors is vital for comprehensive research evaluation.
The key characteristic of these errors is their implications on research credibility. A Type I error can lead to unfounded conclusions, while a Type II error could miss a significant finding. These are particularly relevant in this article's context as they underscore the caution needed in statistical analysis.
The advantage of being aware of these errors is that it can guide researchers in choosing appropriate significance levels and designing better experiments. However, the crucial downside is that there are trade-offs involved; reducing the likelihood of one often increases the risk of the other.
Confidence Intervals
Confidence intervals provide a range of values, derived from sample data, that likely contain the population parameter. They express the uncertainty around an estimate and play a significant role in inferential statistics. Understanding confidence intervals is vital, as they inform researchers about how reliable their estimates are. A higher confidence level indicates greater certainty about the population parameter, which can greatly influence decision-making processes in research.
P-Values and Significance Levels
P-values measure the strength of evidence against the null hypothesis. A lower p-value suggests stronger evidence for the alternative hypothesis. Significance levels, often denoted as alpha, indicate the probability of committing a Type I error. Thus, determining an appropriate alpha level is essential for reliable results.
A key characteristic of p-values is their role in hypothesis testing. They provide an objective measure that helps researchers make informed conclusions. However, the interpretation can sometimes be misleading if not understood properly. Researchers must communicate significance levels transparently to ensure results are not overstated.
Statistical Models
Statistical models serve as a framework for analyzing complex data sets. They simplify reality, allowing researchers to make sense of data and draw conclusions that guide decision-making. By employing statistical models, we can identify patterns, assess relationships, and predict outcomes. This method is integral to effective statistical analysis across various disciplines such as health sciences, social sciences, and business.
Regression Analysis
Regression analysis is a powerful statistical technique that examines the relationship between variables. This method helps uncover insights that could otherwise remain hidden in raw data, making it crucial for data interpretation.
Linear Regression
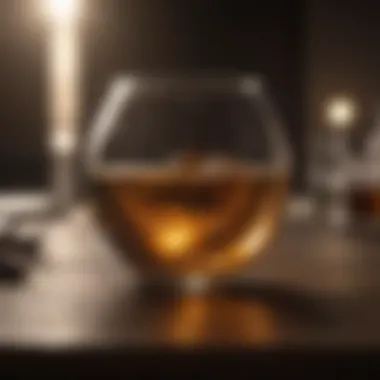
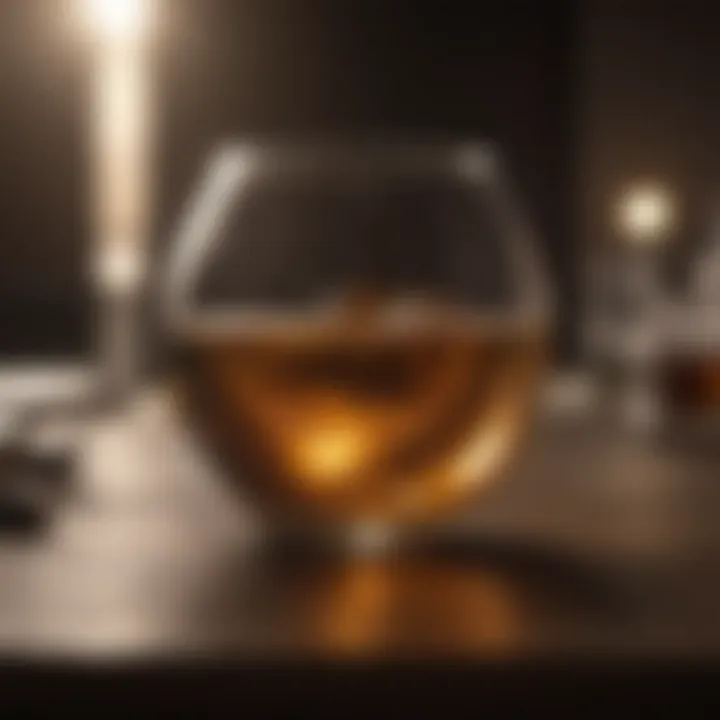
Linear regression is a fundamental approach in regression analysis. It assesses the linear relationship between the dependent variable and one or more independent variables. One key characteristic of linear regression is its simplicity and ease of understanding, making it a popular choice in both academic and professional settings.
Unique feature: Linear regression assumes a straight-line relationship between variables.
Advantages: The results are interpretable, allowing quick insights into how changes in independent variables can predict changes in the dependent variable. However, this linear assumption can also be its disadvantage, as it may not capture more complex relationships.
Logistic Regression
Logistic regression, on the other hand, is used when the dependent variable is categorical. This method estimates the probability that a certain event occurs, such as success or failure. The key characteristic of logistic regression is its ability to handle binary outcomes, making it suitable for many practical applications.
Unique feature: Logistic regression uses the logistic function to model the probability of a certain class.
Advantages: It provides meaningful outcomes in the form of probabilities, enabling more straightforward decision-making in many fields. A downside is the possible assumption of independence, which might not hold in certain datasets, leading to misinterpretations.
ANOVA (Analysis of Variance)
ANOVA is another essential statistical model. It is used to compare means across multiple groups to determine if at least one group mean is significantly different from the others. This method is particularly useful in experimental designs and helps understand group variances.
Key considerations in ANOVA include:
- Assumption of Normality: The data should be normally distributed for valid results.
- Homogeneity of Variances: Variances among groups should be approximately equal.
It is important for researchers to recognize the assumptions behind ANOVA. If these are not met, the results may lead to incorrect conclusions.
By understanding and applying statistical models, one can unlock valuable insights from data, driving informed decisions across various sectors.
Data Visualization in Statistics
Data visualization plays a critical role in statistics, acting as a bridge between complex data structures and comprehensible insights. The mere act of looking at raw data can not only overwhelm a reader but also obscure the underlying patterns and relationships within it. Thus, effective visualization transforms data into a more digestible format, allowing for easier interpretation and faster decision-making. This section will explore the importance of visualization, as well as some commonly used techniques that are beneficial for conveying statistical information.
Importance of Visualization
Visualization serves multiple purposes in the realm of statistics. First, it facilitates understanding. Presenting data graphically enables readers to quickly grasp the information and identify trends, correlations, or anomalies that might otherwise remain hidden in a sea of numbers. Furthermore, visualization can simplify complex concepts, making them more accessible to a broader audience.
Additionally, effective data visualization can enhance presentation. In academic and professional settings, incorporating visuals can engage the audience, making presentations less monotonous and more interactive. Visuals can also provide a uniform way of communicating results. Clarity in representation helps in reducing misinterpretations that may arise from textual data alone, thus maintaining the integrity of the statistical analysis.
Common Visualization Techniques
In statistics, there are several popular visualization techniques that can be employed. Each has its own strengths and suits different types of data. The following subsections detail three commonly used techniques: Histograms, Box Plots, and Scatter Plots.
Histograms
Histograms are a specific type of bar chart that display the distribution of a dataset. They group data points into bins, portraying how many data points fall within each bin. This characteristic makes histograms particularly useful for visualizing the frequency distribution of continuous data.
One of the key attributes of histograms is their ability to show the shape of the data distribution—whether it is normal, skewed, or has outliers. This visual aid is beneficial, as it helps to quickly identify patterns within the data that can inform further analysis or hypothesis testing. However, histograms can have limitations; the choice of bin width can significantly influence the shape of the histogram, potentially leading to different interpretations.
Box Plots
Box plots, also known as whisker plots, summarize a data set by highlighting its median, quartiles, and potential outliers. The key characteristic of box plots is their ability to provide a visual summary of the data's central tendency and variability, all within a single graphical representation.
Box plots are highly beneficial because they allow for easy comparison between multiple data sets, facilitating the identification of differences across groups. The unique feature of box plots is their emphasis on the distribution's spread and potential outliers. Nevertheless, box plots may not convey all necessary details about the data, such as its exact distribution shape, which can limit their interpretative power in deeper statistical analyses.
Scatter Plots
Scatter plots display values for two different variables, providing insights into potential relationships or correlations between them. Each point on the scatter plot represents an observation in the dataset. The key characteristic of scatter plots lies in their ability to allow viewers to visualize both the direction and strength of the relationships present.
Scatter plots are popular because they can reveal trends, clusters, and outliers in the data. They are particularly useful when investigating linear relationships or assessing the correlation between variables. One notable advantage is that they can accommodate a large amount of data without overwhelming the viewer. On the downside, scatter plots can become cluttered when displaying too many points, making it challenging to discern noticeable patterns or trends.
Visual representation of data is essential for effective communication and understanding in statistical practice.
Applications of Statistics
The application of statistics is vital across various fields, acting as a bridge between raw data and informed decision-making. In an era characterized by data proliferation, understanding the practical implications of statistical methods is crucial for researchers, educators, and professionals. Proper application of statistics enables precise predictions, evaluations, and insights that are essential for advancing disciplines such as health sciences, social sciences, and business. The challenges involved in interpreting data, however, underline the importance of applying proper statistical techniques.
Statistics in Health Sciences
In the health sciences, statistics plays a fundamental role in both research and practice. It assists in the design of clinical trials, allowing researchers to determine the efficacy of new treatments or medications. For instance, biostatistics provides the framework to analyze variations in data, offering insights into population health trends.
Several methodologies are employed to ensure the integrity of health data. For example:
- Descriptive statistics summarize patient demographics and clinical characteristics.
- Inferential statistics facilitate hypothesis testing, helping to infer the larger population outcomes based on sample data.
- Multivariate analysis allows researchers to examine the effect of multiple variables simultaneously.
Moreover, epidemiology—a branch of public health—heavily relies on statistical analyses to understand disease patterns, identify risk factors, and formulate intervention strategies. Without a proper statistical approach, findings could lead to misinterpretation, potentially impacting public health initiatives.
Statistics in Social Sciences
Social sciences utilize statistics to analyze behaviors, attitudes, and trends within societies. Fields such as psychology, sociology, and political science depend on statistical methods to interpret data from surveys, experiments, and observational studies. By applying statistics, social scientists can derive meaningful conclusions about social phenomena, such as voting behavior or social networks.
Key statistical applications in social sciences include:
- Survey Sampling: Ensuring that sample populations accurately reflect larger groups.
- Regression Analysis: Understanding relationships between different variables, such as income level and education.
- Factor Analysis: Identifying underlying relationships in large sets of variables, helping to reduce data complexity.
Such statistical techniques enable researchers to quantify social behaviors and ultimately shape public policy based on solid evidence. Ignoring statistical rigor in social sciences risks creating policies based on flawed assumptions.
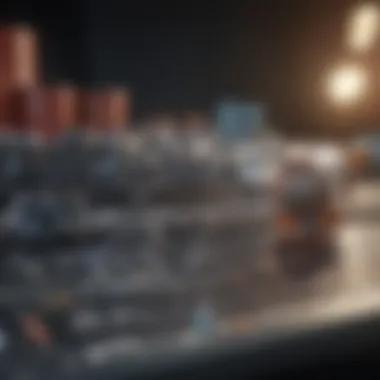
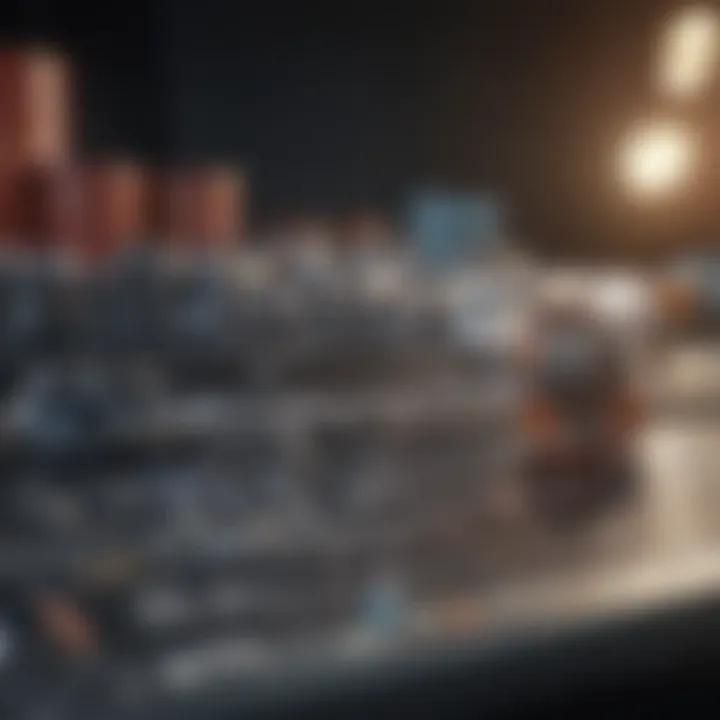
Statistics in Business and Economics
In the business realm, statistics serves as an essential tool for making informed decisions and optimizing operations. Businesses rely on statistical models to forecast sales trends, analyze consumer behavior, and manage risk effectively. For example, market research utilizes sampling and surveys to determine consumer preferences, allowing companies to tailor products accordingly.
Some critical uses of statistics in business and economics include:
- Quality Control: Statistical methods help monitor production processes to maintain product standards.
- Financial Analysis: Businesses employ statistical techniques to interpret financial data and predict market conditions.
- Operational Efficiency: Utilizing statistical models to enhance supply chain management and minimize costs.
Significantly, the implications of these analyses can determine a company's competitive edge in the marketplace. Without proper statistical application, organizations risk making poor decisions that can lead to financial loss or a decline in market share.
Statistics is not just about numbers; it is about drawing actionable insights from data.
Challenges in Statistics
Statistics serves as a tool for elucidating complex phenomena. However, it is not without its challenges. Understanding these issues is crucial for researchers and professionals alike, as the validity and utility of statistical analysis value significantly depend on the quality of the data and the interpretation of results. This section outlines the challenges faced in statistics today, focusing on two critical areas: data quality and integrity, as well as misinterpretation of data. Recognizing these factors can enhance the accuracy of insights derived from statistical work.
Data Quality and Integrity
Data quality is a fundamental pillar in any statistical analysis. High-quality data is accurate, consistent, and reliable. When data quality is compromised, so too are the conclusions drawn from it. For instance, data collection methods play a vital role in determining its quality. Poor sampling methods can lead to skewed results, which may misrepresent reality. In addition, data entry errors, whether human-induced or systemic, can introduce inaccuracies that alter findings.
Integrity concerns often arise in data management. Issues like data fabrication or selective reporting can seriously bias results. Ethical guidelines emphasize the responsibility of researchers to maintain transparency about their methodologies and findings. This integrity not only affects individual studies but also undermines public trust in statistical conclusions and research as a discipline.
It is integral for practitioners to implement rigorous data validation processes. This includes systematic checks for accuracy, adherence to ethical standards in data collection, and maintaining detailed documentation of methodologies.
Misinterpretation of Data
Misinterpretation often occurs due to a lack of understanding of statistical principles. It can lead to misleading conclusions, affecting actions taken based on these findings. For example, failing to distinguish between correlation and causation can result in erroneous assumptions about relationships between variables.
Moreover, the presentation of data can contribute to misinterpretation. Charts and graphs, while useful for summarizing information, can sometimes be misleading. Selecting inappropriate scales or omitting significant data can alter perceptions and interpretations. This highlights the importance of clear communication in data representation.
"Quality data leads to quality insights, while misinterpretation can cloud even the best analysis."
Ethics in Statistical Analysis
Ethics in statistical analysis is a fundamental principle that guides researchers, analysts, and professionals in the responsible handling of data. It is crucial for maintaining the integrity of research findings and ensuring that statistical practices do not mislead or harm individuals or communities. Without ethical considerations, the validity of data interpretation could become compromised, leading to detrimental effects in various fields such as healthcare, social sciences, and business.
One major aspect of this topic is the commitment to honesty in reporting and presenting data. Analysts must ensure that they do not selectively use data that supports their conclusions while ignoring contradictory evidence. Additionally, transparency in methodology is essential. By clearly outlining the methods used for data collection and analysis, researchers can allow others to replicate their studies, fostering trust and accountability in the science community.
Moreover, ethical guidelines help protect the rights and welfare of individuals involved in research. This includes obtaining informed consent when collecting data from participants and ensuring confidentiality where required. Ultimately, adhering to ethical standards promotes public trust in statistical findings, which is essential for informed decision-making and policy formulation.
Ethical Guidelines
Ethical guidelines in statistical analysis provide a framework for ensuring that research is conducted responsibly. These guidelines include:
- Informed Consent: Participants should be given clear information about the nature of the study and must voluntarily agree to partake.
- Confidentiality: Personal data must be kept confidential to protect the identities of participants. Data should be anonymized wherever feasible.
- Integrity in Reporting: Researchers must present their findings honestly, avoiding manipulation of data to fit a particular narrative.
- Respect for Participants: All studies should treat participants respectfully, considering their rights, needs, and welfare at all times.
- Accountability: Researchers and analysts should take responsibility for their work, ensuring that ethical standards guide their practices.
By adhering to these guidelines, researchers can ensure that their work contributes positively to scientific knowledge and societal welfare.
Consequences of Ethical Violations
Violations of ethical standards in statistical analysis can lead to serious consequences, both for individuals and the broader community. Some potential outcomes include:
- Loss of Credibility: Researchers who engage in unethical practices may lose credibility in their field, which can diminish future research opportunities and collaborations.
- Misleading Conclusions: Unethical manipulation of data can lead to erroneous conclusions that result in flawed policies or decisions.
- Legal Ramifications: In some cases, violations can lead to legal action, particularly if personal data is misused or if participants' rights are violated.
- Harm to Individuals and Society: Ultimately, unethical statistical practices can have significant repercussions, including harm to individuals who may be affected by incorrect findings or poor decision-making based on misleading data.
It is essential for professionals to recognize that the implications of their statistical analyses extend beyond the confines of academic debate, influencing real-world outcomes.
In summary, ethical considerations are not merely an afterthought in statistical analysis; they form the backbone of responsible research practices. Addressing ethical guidelines and understanding potential consequences is vital for fostering integrity in research and ensuring the beneficial use of statistical tools in society.
Future Trends in Statistics
Statistics is evolving rapidly, driven by technological advancements and the growing importance of data in decision-making processes. In this section, we will explore the significance of emerging trends in the field of statistics. Understanding these trends allows researchers, educators, and professionals to remain at the forefront of their discipline.
Integration of Big Data
Big data refers to the massive volumes of data generated every second from various sources like social media, IoT devices, and online transactions. The integration of big data into statistical analysis has revolutionized how we approach problems. This trend enables statisticians to analyze complex datasets to derive insights that were once unattainable.
Some key benefits of integrating big data include:
- Improved Predictive Analytics: With access to vast datasets, statistical models can be more precise in forecasting outcomes, from stock market trends to public health crises.
- Real-Time Analysis: The capability to analyze data in real-time allows for immediate interventions in various fields, such as emergency response and financial markets.
- Enhanced Understanding of Patterns: Large datasets reveal patterns that smaller samples often miss, leading to more comprehensive insights across disciplines.
However, this integration also poses several challenges:
- Data Quality: Ensuring the reliability and accuracy of big data remains a primary concern.
- Privacy Considerations: As data collection becomes more extensive, the ethical implications surrounding personal data usage must be addressed.
"Integrating big data into statistical analysis transcends traditional methods, allowing for more nuanced and informed decision-making."
Advancements in Statistical Software
The advancement of statistical software has had a profound impact on the field of statistics. Tools like R, Python, and SPSS evolve continuously to support more sophisticated analyses and visualizations.
Key considerations regarding these advancements include:
- Increased Accessibility: Modern software platforms have user-friendly interfaces, making statistical analysis more accessible to non-experts, which broadens the scope of statistical applications.
- Collaboration Features: Many software solutions allow for collaborative work, enhancing the ability to share insights and findings among researchers and practitioners.
- Automation of Analyses: Automation features within these tools can expedite the process of data cleaning, analysis, and reporting.
Despite these advancements, users must remain aware of:
- Dependence on Software: Over-reliance on software could potentially obscure fundamental statistical concepts.
- Need for Ongoing Education: As these tools evolve, continuous education becomes crucial for statisticians to leverage new features effectively.
In summary, the landscape of statistics is undergoing significant changes with the integration of big data and advancements in statistical software. These trends present both opportunities and challenges that require thoughtful consideration to harness their full potential.